What is Image Outpainting? Image outpainting is the process of generating new image content outside the boundaries of an existing image.
Papers and Code
Jun 24, 2025
Abstract:Existing 4D synthesis methods primarily focus on object-level generation or dynamic scene synthesis with limited novel views, restricting their ability to generate multi-view consistent and immersive dynamic 4D scenes. To address these constraints, we propose a framework (dubbed as CoCo4D) for generating detailed dynamic 4D scenes from text prompts, with the option to include images. Our method leverages the crucial observation that articulated motion typically characterizes foreground objects, whereas background alterations are less pronounced. Consequently, CoCo4D divides 4D scene synthesis into two responsibilities: modeling the dynamic foreground and creating the evolving background, both directed by a reference motion sequence. Given a text prompt and an optional reference image, CoCo4D first generates an initial motion sequence utilizing video diffusion models. This motion sequence then guides the synthesis of both the dynamic foreground object and the background using a novel progressive outpainting scheme. To ensure seamless integration of the moving foreground object within the dynamic background, CoCo4D optimizes a parametric trajectory for the foreground, resulting in realistic and coherent blending. Extensive experiments show that CoCo4D achieves comparable or superior performance in 4D scene generation compared to existing methods, demonstrating its effectiveness and efficiency. More results are presented on our website https://colezwhy.github.io/coco4d/.
* 16 pages,10 figures
Via
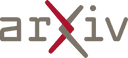
Jun 14, 2025
Abstract:Image inpainting is the task of reconstructing missing or damaged parts of an image in a way that seamlessly blends with the surrounding content. With the advent of advanced generative models, especially diffusion models and generative adversarial networks, inpainting has achieved remarkable improvements in visual quality and coherence. However, achieving seamless continuity remains a significant challenge. In this work, we propose two novel methods to address discrepancy issues in diffusion-based inpainting models. First, we introduce a modified Variational Autoencoder that corrects color imbalances, ensuring that the final inpainted results are free of color mismatches. Second, we propose a two-step training strategy that improves the blending of generated and existing image content during the diffusion process. Through extensive experiments, we demonstrate that our methods effectively reduce discontinuity and produce high-quality inpainting results that are coherent and visually appealing.
Via
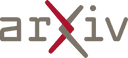
Jun 16, 2025
Abstract:Diffusion models are advancing autonomous driving by enabling realistic data synthesis, predictive end-to-end planning, and closed-loop simulation, with a primary focus on temporally consistent generation. However, the generation of large-scale 3D scenes that require spatial coherence remains underexplored. In this paper, we propose X-Scene, a novel framework for large-scale driving scene generation that achieves both geometric intricacy and appearance fidelity, while offering flexible controllability. Specifically, X-Scene supports multi-granular control, including low-level conditions such as user-provided or text-driven layout for detailed scene composition and high-level semantic guidance such as user-intent and LLM-enriched text prompts for efficient customization. To enhance geometrical and visual fidelity, we introduce a unified pipeline that sequentially generates 3D semantic occupancy and the corresponding multiview images, while ensuring alignment between modalities. Additionally, we extend the generated local region into a large-scale scene through consistency-aware scene outpainting, which extrapolates new occupancy and images conditioned on the previously generated area, enhancing spatial continuity and preserving visual coherence. The resulting scenes are lifted into high-quality 3DGS representations, supporting diverse applications such as scene exploration. Comprehensive experiments demonstrate that X-Scene significantly advances controllability and fidelity for large-scale driving scene generation, empowering data generation and simulation for autonomous driving.
* 28 pages, 9 figures, Project page at https://x-scene.github.io/
Via
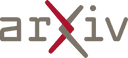
Jun 05, 2025
Abstract:Reconstructing semantic-aware 3D scenes from sparse views is a challenging yet essential research direction, driven by the demands of emerging applications such as virtual reality and embodied AI. Existing per-scene optimization methods require dense input views and incur high computational costs, while generalizable approaches often struggle to reconstruct regions outside the input view cone. In this paper, we propose OGGSplat, an open Gaussian growing method that expands the field-of-view in generalizable 3D reconstruction. Our key insight is that the semantic attributes of open Gaussians provide strong priors for image extrapolation, enabling both semantic consistency and visual plausibility. Specifically, once open Gaussians are initialized from sparse views, we introduce an RGB-semantic consistent inpainting module applied to selected rendered views. This module enforces bidirectional control between an image diffusion model and a semantic diffusion model. The inpainted regions are then lifted back into 3D space for efficient and progressive Gaussian parameter optimization. To evaluate our method, we establish a Gaussian Outpainting (GO) benchmark that assesses both semantic and generative quality of reconstructed open-vocabulary scenes. OGGSplat also demonstrates promising semantic-aware scene reconstruction capabilities when provided with two view images captured directly from a smartphone camera.
Via
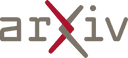
May 22, 2025
Abstract:Recent progress in panoramic image generation has underscored two critical limitations in existing approaches. First, most methods are built upon diffusion models, which are inherently ill-suited for equirectangular projection (ERP) panoramas due to the violation of the identically and independently distributed (i.i.d.) Gaussian noise assumption caused by their spherical mapping. Second, these methods often treat text-conditioned generation (text-to-panorama) and image-conditioned generation (panorama outpainting) as separate tasks, relying on distinct architectures and task-specific data. In this work, we propose a unified framework, Panoramic AutoRegressive model (PAR), which leverages masked autoregressive modeling to address these challenges. PAR avoids the i.i.d. assumption constraint and integrates text and image conditioning into a cohesive architecture, enabling seamless generation across tasks. To address the inherent discontinuity in existing generative models, we introduce circular padding to enhance spatial coherence and propose a consistency alignment strategy to improve generation quality. Extensive experiments demonstrate competitive performance in text-to-image generation and panorama outpainting tasks while showcasing promising scalability and generalization capabilities.
Via
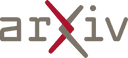
Mar 13, 2025
Abstract:Vision-and-language navigation (VLN) tasks require agents to navigate three-dimensional environments guided by natural language instructions, offering substantial potential for diverse applications. However, the scarcity of training data impedes progress in this field. This paper introduces PanoGen++, a novel framework that addresses this limitation by generating varied and pertinent panoramic environments for VLN tasks. PanoGen++ incorporates pre-trained diffusion models with domain-specific fine-tuning, employing parameter-efficient techniques such as low-rank adaptation to minimize computational costs. We investigate two settings for environment generation: masked image inpainting and recursive image outpainting. The former maximizes novel environment creation by inpainting masked regions based on textual descriptions, while the latter facilitates agents' learning of spatial relationships within panoramas. Empirical evaluations on room-to-room (R2R), room-for-room (R4R), and cooperative vision-and-dialog navigation (CVDN) datasets reveal significant performance enhancements: a 2.44% increase in success rate on the R2R test leaderboard, a 0.63% improvement on the R4R validation unseen set, and a 0.75-meter enhancement in goal progress on the CVDN validation unseen set. PanoGen++ augments the diversity and relevance of training environments, resulting in improved generalization and efficacy in VLN tasks.
* This paper was accepted by Neural Networks
Via
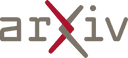
Mar 13, 2025
Abstract:We introduce ARPG, a novel visual autoregressive model that enables randomized parallel generation, addressing the inherent limitations of conventional raster-order approaches, which hinder inference efficiency and zero-shot generalization due to their sequential, predefined token generation order. Our key insight is that effective random-order modeling necessitates explicit guidance for determining the position of the next predicted token. To this end, we propose a novel guided decoding framework that decouples positional guidance from content representation, encoding them separately as queries and key-value pairs. By directly incorporating this guidance into the causal attention mechanism, our approach enables fully random-order training and generation, eliminating the need for bidirectional attention. Consequently, ARPG readily generalizes to zero-shot tasks such as image inpainting, outpainting, and resolution expansion. Furthermore, it supports parallel inference by concurrently processing multiple queries using a shared KV cache. On the ImageNet-1K 256 benchmark, our approach attains an FID of 1.94 with only 64 sampling steps, achieving over a 20-fold increase in throughput while reducing memory consumption by over 75% compared to representative recent autoregressive models at a similar scale.
Via
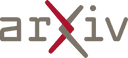
Mar 03, 2025
Abstract:In this paper, we propose a novel garment-centric outpainting (GCO) framework based on the latent diffusion model (LDM) for fine-grained controllable apparel showcase image generation. The proposed framework aims at customizing a fashion model wearing a given garment via text prompts and facial images. Different from existing methods, our framework takes a garment image segmented from a dressed mannequin or a person as the input, eliminating the need for learning cloth deformation and ensuring faithful preservation of garment details. The proposed framework consists of two stages. In the first stage, we introduce a garment-adaptive pose prediction model that generates diverse poses given the garment. Then, in the next stage, we generate apparel showcase images, conditioned on the garment and the predicted poses, along with specified text prompts and facial images. Notably, a multi-scale appearance customization module (MS-ACM) is designed to allow both overall and fine-grained text-based control over the generated model's appearance. Moreover, we leverage a lightweight feature fusion operation without introducing any extra encoders or modules to integrate multiple conditions, which is more efficient. Extensive experiments validate the superior performance of our framework compared to state-of-the-art methods.
Via
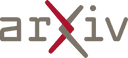
Mar 14, 2025
Abstract:Camera control has been actively studied in text or image conditioned video generation tasks. However, altering camera trajectories of a given video remains under-explored, despite its importance in the field of video creation. It is non-trivial due to the extra constraints of maintaining multiple-frame appearance and dynamic synchronization. To address this, we present ReCamMaster, a camera-controlled generative video re-rendering framework that reproduces the dynamic scene of an input video at novel camera trajectories. The core innovation lies in harnessing the generative capabilities of pre-trained text-to-video models through a simple yet powerful video conditioning mechanism -- its capability often overlooked in current research. To overcome the scarcity of qualified training data, we construct a comprehensive multi-camera synchronized video dataset using Unreal Engine 5, which is carefully curated to follow real-world filming characteristics, covering diverse scenes and camera movements. It helps the model generalize to in-the-wild videos. Lastly, we further improve the robustness to diverse inputs through a meticulously designed training strategy. Extensive experiments tell that our method substantially outperforms existing state-of-the-art approaches and strong baselines. Our method also finds promising applications in video stabilization, super-resolution, and outpainting. Project page: https://jianhongbai.github.io/ReCamMaster/
Via
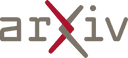
Feb 08, 2025
Abstract:Recent deep learning-based image completion methods, including both inpainting and outpainting, have demonstrated promising results in restoring corrupted images by effectively filling various missing regions. Among these, Generative Adversarial Networks (GANs) and Denoising Diffusion Probabilistic Models (DDPMs) have been employed as key generative image completion approaches, excelling in the field of generating high-quality restorations with reduced artifacts and improved fine details. In previous work, we developed a method aimed at synthesizing views from novel microscope positions for mastoidectomy surgeries; however, that approach did not have the ability to restore the surrounding surgical scene environment. In this paper, we propose an efficient method to complete the surgical scene of the synthetic postmastoidectomy dataset. Our approach leverages self-supervised learning on real surgical datasets to train a Single-Step Denoising Diffusion-GAN (SSDD-GAN), combining the advantages of diffusion models with the adversarial optimization of GANs for improved Structural Similarity results of 6%. The trained model is then directly applied to the synthetic postmastoidectomy dataset using a zero-shot approach, enabling the generation of realistic and complete surgical scenes without the need for explicit ground-truth labels from the synthetic postmastoidectomy dataset. This method addresses key limitations in previous work, offering a novel pathway for full surgical microscopy scene completion and enhancing the usability of the synthetic postmastoidectomy dataset in surgical preoperative planning and intraoperative navigation.
Via
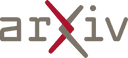