May 22, 2025
Abstract:Generative models, particularly diffusion model, have emerged as powerful tools for sequential recommendation. However, accurately modeling user preferences remains challenging due to the noise perturbations inherent in the forward and reverse processes of diffusion-based methods. Towards this end, this study introduces FMRec, a Flow Matching based model that employs a straight flow trajectory and a modified loss tailored for the recommendation task. Additionally, from the diffusion-model perspective, we integrate a reconstruction loss to improve robustness against noise perturbations, thereby retaining user preferences during the forward process. In the reverse process, we employ a deterministic reverse sampler, specifically an ODE-based updating function, to eliminate unnecessary randomness, thereby ensuring that the generated recommendations closely align with user needs. Extensive evaluations on four benchmark datasets reveal that FMRec achieves an average improvement of 6.53% over state-of-the-art methods. The replication code is available at https://github.com/FengLiu-1/FMRec.
* 11 pages, 8 figures, IJCAI 2025 Accepted Work
Via
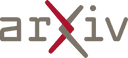