What is Object Detection? Object detection is a computer vision task in which the goal is to detect and locate objects of interest in an image or video. The task involves identifying the position and boundaries of objects in an image, and classifying the objects into different categories. It forms a crucial part of vision recognition, alongside image classification and retrieval.
Papers and Code
Jun 10, 2025
Abstract:Adversarial attacks on Natural Language Processing (NLP) models expose vulnerabilities by introducing subtle perturbations to input text, often leading to misclassification while maintaining human readability. Existing methods typically focus on word-level or local text segment alterations, overlooking the broader context, which results in detectable or semantically inconsistent perturbations. We propose a novel adversarial text attack scheme named Dynamic Contextual Perturbation (DCP). DCP dynamically generates context-aware perturbations across sentences, paragraphs, and documents, ensuring semantic fidelity and fluency. Leveraging the capabilities of pre-trained language models, DCP iteratively refines perturbations through an adversarial objective function that balances the dual objectives of inducing model misclassification and preserving the naturalness of the text. This comprehensive approach allows DCP to produce more sophisticated and effective adversarial examples that better mimic natural language patterns. Our experimental results, conducted on various NLP models and datasets, demonstrate the efficacy of DCP in challenging the robustness of state-of-the-art NLP systems. By integrating dynamic contextual analysis, DCP significantly enhances the subtlety and impact of adversarial attacks. This study highlights the critical role of context in adversarial attacks and lays the groundwork for creating more robust NLP systems capable of withstanding sophisticated adversarial strategies.
* Proceedings of the IEEE Calcutta Conference (CALCON), Kolkata,
India, 2024, pp. 1-6
* This is the accepted version of the paper, which was presented at
IEEE CALCON. The conference was organized at Jadavpur University, Kolkata,
from December 14 to 15, 2025. The paper is six pages long, and it consists of
six tables and six figures. This is not the final camera-ready version of the
paper
Via
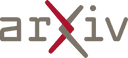
May 30, 2025
Abstract:Visual Grounding is a task that aims to localize a target region in an image based on a free-form natural language description. With the rise of Transformer architectures, there is an increasing need for larger datasets to boost performance. However, the high cost of manual annotation poses a challenge, hindering the scale of data and the ability of large models to enhance their effectiveness. Previous pseudo label generation methods heavily rely on human-labeled captions of the original dataset, limiting scalability and diversity. To address this, we propose D2AF, a robust annotation framework for visual grounding using only input images. This approach overcomes dataset size limitations and enriches both the quantity and diversity of referring expressions. Our approach leverages multimodal large models and object detection models. By implementing dual-driven annotation strategies, we effectively generate detailed region-text pairs using both closed-set and open-set approaches. We further conduct an in-depth analysis of data quantity and data distribution. Our findings demonstrate that increasing data volume enhances model performance. However, the degree of improvement depends on how well the pseudo labels broaden the original data distribution. Based on these insights, we propose a consistency and distribution aware filtering method to further improve data quality by effectively removing erroneous and redundant data. This approach effectively eliminates noisy data, leading to improved performance. Experiments on three visual grounding tasks demonstrate that our method significantly improves the performance of existing models and achieves state-of-the-art results.
* 16pages, 8figures
Via
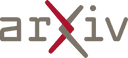
Jun 05, 2025
Abstract:The use of robotics in humanitarian demining increasingly involves computer vision techniques to improve landmine detection capabilities. However, in the absence of diverse and realistic datasets, the reliable validation of algorithms remains a challenge for the research community. In this paper, we introduce MineInsight, a publicly available multi-sensor, multi-spectral dataset designed for off-road landmine detection. The dataset features 35 different targets (15 landmines and 20 commonly found objects) distributed along three distinct tracks, providing a diverse and realistic testing environment. MineInsight is, to the best of our knowledge, the first dataset to integrate dual-view sensor scans from both an Unmanned Ground Vehicle and its robotic arm, offering multiple viewpoints to mitigate occlusions and improve spatial awareness. It features two LiDARs, as well as images captured at diverse spectral ranges, including visible (RGB, monochrome), visible short-wave infrared (VIS-SWIR), and long-wave infrared (LWIR). Additionally, the dataset comes with an estimation of the location of the targets, offering a benchmark for evaluating detection algorithms. We recorded approximately one hour of data in both daylight and nighttime conditions, resulting in around 38,000 RGB frames, 53,000 VIS-SWIR frames, and 108,000 LWIR frames. MineInsight serves as a benchmark for developing and evaluating landmine detection algorithms. Our dataset is available at https://github.com/mariomlz99/MineInsight.
* This work has been submitted to the IEEE for possible publication
Via
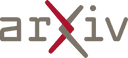
Jun 08, 2025
Abstract:While multimodal large language models excel at various tasks, they still suffer from hallucinations, which limit their reliability and scalability for broader domain applications. To address this issue, recent research mainly focuses on objective hallucination. However, for sequential images, besides objective hallucination, there is also behavioral hallucination, which is less studied. This work aims to fill in the gap. We first reveal that behavioral hallucinations mainly arise from two key factors: prior-driven bias and the snowball effect. Based on these observations, we introduce SHE (Sequence Hallucination Eradication), a lightweight, two-stage framework that (1) detects hallucinations via visual-textual alignment check using our proposed adaptive temporal window and (2) mitigates them via orthogonal projection onto the joint embedding space. We also propose a new metric (BEACH) to quantify behavioral hallucination severity. Empirical results on standard benchmarks demonstrate that SHE reduces behavioral hallucination by over 10% on BEACH while maintaining descriptive accuracy.
Via
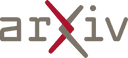
Jun 06, 2025
Abstract:Multi-label Classification (MLC) assigns an instance to one or more non-exclusive classes. A challenge arises when the dataset contains a large proportion of instances with no assigned class, referred to as negative data, which can overwhelm the learning process and hinder the accurate identification and classification of positive instances. Nevertheless, it is common in MLC applications such as industrial defect detection, agricultural disease identification, and healthcare diagnosis to encounter large amounts of negative data. Assigning a separate negative class to these instances further complicates the learning objective and introduces unnecessary redundancies. To address this challenge, we redesign standard MLC loss functions by deriving a likelihood of any class being present, formulated by a normalized weighted geometric mean of the predicted class probabilities. We introduce a regularization parameter that controls the relative contribution of the absent class probabilities to the any-class presence likelihood in positive instances. The any-class presence likelihood complements the multi-label learning by encouraging the network to become more aware of implicit positive instances and improve the label classification within those positive instances. Experiments on large-scale datasets with negative data: SewerML, modified COCO, and ChestX-ray14, across various networks and base loss functions show that our loss functions consistently improve MLC performance of their standard loss counterparts, achieving gains of up to 6.01 percentage points in F1, 8.06 in F2, and 3.11 in mean average precision, all without additional parameters or computational complexity. Code available at: https://github.com/ML-for-Sensor-Data-Western/gmean-mlc
Via
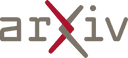
Jun 05, 2025
Abstract:Integrated sensing and communication (ISAC) has been envisioned to play a more important role in future wireless networks. However, the design of ISAC networks is challenging, especially when there are multiple communication and sensing (C\&S) nodes and multiple sensing targets. We investigate a multi-base station (BS) ISAC network in which multiple BSs equipped with multiple antennas simultaneously provide C\&S services for multiple ground communication users (CUs) and targets. To enhance the overall performance of C\&S, we formulate a joint user association (UA) and multi-BS transmit beamforming optimization problem with the objective of maximizing the total sum rate of all CUs while ensuring both the minimum target detection and parameter estimation requirements. To efficiently solve the highly non-convex mixed integer nonlinear programming (MINLP) optimization problem, we propose an alternating optimization (AO)-based algorithm that decomposes the problem into two sub-problems, i.e., UA optimization and multi-BS transmit beamforming optimization. Inspired by large language models (LLMs) for prediction and inference, we propose a unified framework integrating LLMs with convex-based optimization methods. First, we propose a comprehensive design of prompt engineering, including few-shot, chain of thought, and self-reflection techniques to guide LLMs in solving the binary integer programming UA optimization problem. Second, we utilize convex-based optimization methods to handle the non-convex beamforming optimization problem based on fractional programming (FP), majorization minimization (MM), and the alternating direction method of multipliers (ADMM) with an optimized UA from LLMs. Numerical results demonstrate that our proposed LLM-enabled AO-based algorithm achieves fast convergence and near upper-bound performance with the GPT-o1 model, outperforming various benchmark schemes.
Via
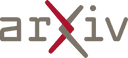
Jun 10, 2025
Abstract:Objective: To characterize stigma dimensions, social, and related behavioral circumstances in people living with HIV (PLWHs) seeking care, using natural language processing methods applied to a large collection of electronic health record (EHR) clinical notes from a large integrated health system in the southeast United States. Methods: We identified 9,140 cohort of PLWHs from the UF Health IDR and performed topic modeling analysis using Latent Dirichlet Allocation (LDA) to uncover stigma dimensions, social, and related behavioral circumstances. Domain experts created a seed list of HIV-related stigma keywords, then applied a snowball strategy to iteratively review notes for additional terms until saturation was reached. To identify more target topics, we tested three keyword-based filtering strategies. Domain experts manually reviewed the detected topics using the prevalent terms and key discussion topics. Word frequency analysis was used to highlight the prevalent terms associated with each topic. In addition, we conducted topic variation analysis among subgroups to examine differences across age and sex-specific demographics. Results and Conclusion: Topic modeling on sentences containing at least one keyword uncovered a wide range of topic themes associated with HIV-related stigma, social, and related behaviors circumstances, including "Mental Health Concern and Stigma", "Social Support and Engagement", "Limited Healthcare Access and Severe Illness", "Treatment Refusal and Isolation" and so on. Topic variation analysis across age subgroups revealed differences. Extracting and understanding the HIV-related stigma dimensions, social, and related behavioral circumstances from EHR clinical notes enables scalable, time-efficient assessment, overcoming the limitations of traditional questionnaires and improving patient outcomes.
Via
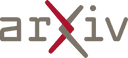
Jun 09, 2025
Abstract:While existing benchmarks probe the reasoning abilities of large language models (LLMs) across diverse domains, they predominantly assess passive reasoning, providing models with all the information needed to reach a solution. By contrast, active reasoning-where an LLM must interact with external systems to acquire missing evidence or data-has received little systematic attention. To address this shortfall, we present AR-Bench, a novel benchmark designed explicitly to evaluate an LLM's active reasoning skills. AR-Bench comprises three task families-detective cases, situation puzzles, and guessing numbers-that together simulate real-world, agentic scenarios and measure performance across commonsense, logical, and symbolic reasoning challenges. Empirical evaluation on AR-Bench demonstrates that contemporary LLMs exhibit pronounced difficulties with active reasoning: they frequently fail to acquire or leverage the information needed to solve tasks. This gap highlights a stark divergence between their passive and active reasoning abilities. Moreover, ablation studies indicate that even advanced strategies, such as tree-based searching or post-training approaches, yield only modest gains and fall short of the levels required for real-world deployment. Collectively, these findings highlight the critical need to advance methodology for active reasoning, e.g., incorporating interactive learning, real-time feedback loops, and environment-aware objectives for training. The benchmark is publicly available at: https://github.com/tmlr-group/AR-Bench.
* Accepted by ICML 2025
Via
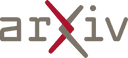
Jun 06, 2025
Abstract:Sensor fusion is crucial for a performant and robust Perception system in autonomous vehicles, but sensor staleness, where data from different sensors arrives with varying delays, poses significant challenges. Temporal misalignment between sensor modalities leads to inconsistent object state estimates, severely degrading the quality of trajectory predictions that are critical for safety. We present a novel and model-agnostic approach to address this problem via (1) a per-point timestamp offset feature (for LiDAR and radar both relative to camera) that enables fine-grained temporal awareness in sensor fusion, and (2) a data augmentation strategy that simulates realistic sensor staleness patterns observed in deployed vehicles. Our method is integrated into a perspective-view detection model that consumes sensor data from multiple LiDARs, radars and cameras. We demonstrate that while a conventional model shows significant regressions when one sensor modality is stale, our approach reaches consistently good performance across both synchronized and stale conditions.
* This paper has been accepted by CVPR 2025 Precognition Workshop
Via
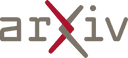
Jun 04, 2025
Abstract:Finding reliable matches is essential in multi-object tracking to ensure the accuracy and reliability of perception systems in safety-critical applications such as autonomous vehicles. Effective matching mitigates perception errors, enhancing object identification and tracking for improved performance and safety. However, traditional metrics such as Intersection over Union (IoU) and Center Point Distances (CPDs), which are effective in 2D image planes, often fail to find critical matches in complex 3D scenes. To address this limitation, we introduce Contour Errors (CEs), an ego or object-centric metric for identifying matches of interest in tracking scenarios from a functional perspective. By comparing bounding boxes in the ego vehicle's frame, contour errors provide a more functionally relevant assessment of object matches. Extensive experiments on the nuScenes dataset demonstrate that contour errors improve the reliability of matches over the state-of-the-art 2D IoU and CPD metrics in tracking-by-detection methods. In 3D car tracking, our results show that Contour Errors reduce functional failures (FPs/FNs) by 80% at close ranges and 60% at far ranges compared to IoU in the evaluation stage.
Via
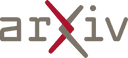