May 16, 2025
Abstract:Recently, training-free methods for improving large language models (LLMs) have attracted growing interest, with token-level attention tuning emerging as a promising and interpretable direction. However, existing methods typically rely on auxiliary mechanisms to identify important or irrelevant task-specific tokens, introducing potential bias and limiting applicability. In this paper, we uncover a surprising and elegant alternative: the semantically empty initial token is a powerful and underexplored control point for optimizing model behavior. Through theoretical analysis, we show that tuning the initial token's attention sharpens or flattens the attention distribution over subsequent tokens, and its role as an attention sink amplifies this effect. Empirically, we find that: (1) tuning its attention improves LLM performance more effectively than tuning other task-specific tokens; (2) the effect follows a consistent trend across layers, with earlier layers having greater impact, but varies across attention heads, with different heads showing distinct preferences in how they attend to this token. Based on these findings, we propose ZeroTuning, a training-free approach that improves LLM performance by applying head-specific attention adjustments to this special token. Despite tuning only one token, ZeroTuning achieves higher performance on text classification, multiple-choice, and multi-turn conversation tasks across models such as Llama, Qwen, and DeepSeek. For example, ZeroTuning improves Llama-3.1-8B by 11.71% on classification, 2.64% on QA tasks, and raises its multi-turn score from 7.804 to 7.966. The method is also robust to limited resources, few-shot settings, long contexts, quantization, decoding strategies, and prompt variations. Our work sheds light on a previously overlooked control point in LLMs, offering new insights into both inference-time tuning and model interpretability.
Via
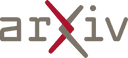