May 26, 2025
Abstract:Text-driven video editing is rapidly advancing, yet its rigorous evaluation remains challenging due to the absence of dedicated video quality assessment (VQA) models capable of discerning the nuances of editing quality. To address this critical gap, we introduce TDVE-DB, a large-scale benchmark dataset for text-driven video editing. TDVE-DB consists of 3,857 edited videos generated from 12 diverse models across 8 editing categories, and is annotated with 173,565 human subjective ratings along three crucial dimensions, i.e., edited video quality, editing alignment, and structural consistency. Based on TDVE-DB, we first conduct a comprehensive evaluation for the 12 state-of-the-art editing models revealing the strengths and weaknesses of current video techniques, and then benchmark existing VQA methods in the context of text-driven video editing evaluation. Building on these insights, we propose TDVE-Assessor, a novel VQA model specifically designed for text-driven video editing assessment. TDVE-Assessor integrates both spatial and temporal video features into a large language model (LLM) for rich contextual understanding to provide comprehensive quality assessment. Extensive experiments demonstrate that TDVE-Assessor substantially outperforms existing VQA models on TDVE-DB across all three evaluation dimensions, setting a new state-of-the-art. Both TDVE-DB and TDVE-Assessor will be released upon the publication.
* 25 pages, 14 figures, 8 tables
Via
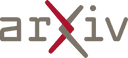