Apr 24, 2025
Abstract:Unresolved questions about how autonomous vehicles (AVs) should meet the informational needs of riders hinder real-world adoption. Complicating our ability to satisfy rider needs is that different people, goals, and driving contexts have different criteria for what constitutes interaction success. Unfortunately, most human-AV research and design today treats all people and situations uniformly. It is crucial to understand how an AV should communicate to meet rider needs, and how communications should change when the human-AV complex system changes. I argue that understanding the relationships between different aspects of the human-AV system can help us build improved and adaptable AV communications. I support this argument using three empirical studies. First, I identify optimal communication strategies that enhance driving performance, confidence, and trust for learning in extreme driving environments. Findings highlight the need for task-sensitive, modality-appropriate communications tuned to learner cognitive limits and goals. Next, I highlight the consequences of deploying faulty communication systems and demonstrate the need for context-sensitive communications. Third, I use machine learning (ML) to illuminate personal factors predicting trust in AVs, emphasizing the importance of tailoring designs to individual traits and concerns. Together, this dissertation supports the necessity of transparent, adaptable, and personalized AV systems that cater to individual needs, goals, and contextual demands. By considering the complex system within which human-AV interactions occur, we can deliver valuable insights for designers, researchers, and policymakers. This dissertation also provides a concrete domain to study theories of human-machine joint action and situational awareness, and can be used to guide future human-AI interaction research. [shortened for arxiv]
* PhD Dissertation from University of California, San Diego; 175 pages
Via
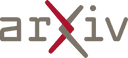