Dec 02, 2024
Abstract:Nowadays, many tools are used to facilitate forensic tasks about data extraction and data analysis. In particular, some tools leverage Artificial Intelligence (AI) to automatically label examined data into specific categories (\ie, drugs, weapons, nudity). However, this raises a serious concern about the robustness of the employed AI algorithms against adversarial attacks. Indeed, some people may need to hide specific data to AI-based digital forensics tools, thus manipulating the content so that the AI system does not recognize the offensive/prohibited content and marks it at as suspicious to the analyst. This could be seen as an anti-forensics attack scenario. For this reason, we analyzed two of the most important forensics tools employing AI for data classification: Magnet AI, used by Magnet Axiom, and Excire Photo AI, used by X-Ways Forensics. We made preliminary tests using about $200$ images, other $100$ sent in $3$ chats about pornography and teenage nudity, drugs and weapons to understand how the tools label them. Moreover, we loaded some deepfake images (images generated by AI forging real ones) of some actors to understand if they would be classified in the same category as the original images. From our preliminary study, we saw that the AI algorithm is not robust enough, as we expected since these topics are still open research problems. For example, some sexual images were not categorized as nudity, and some deepfakes were categorized as the same real person, while the human eye can see the clear nudity image or catch the difference between the deepfakes. Building on these results and other state-of-the-art works, we provide some suggestions for improving how digital forensics analysis tool leverage AI and their robustness against adversarial attacks or different scenarios than the trained one.
Via
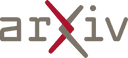