Nov 26, 2024
Abstract:The widespread dissemination of hate speech, harassment, harmful and sexual content, and violence across websites and media platforms presents substantial challenges and provokes widespread concern among different sectors of society. Governments, educators, and parents are often at odds with media platforms about how to regulate, control, and limit the spread of such content. Technologies for detecting and censoring the media contents are a key solution to addressing these challenges. Techniques from natural language processing and computer vision have been used widely to automatically identify and filter out sensitive content such as offensive languages, violence, nudity, and addiction in both text, images, and videos, enabling platforms to enforce content policies at scale. However, existing methods still have limitations in achieving high detection accuracy with fewer false positives and false negatives. Therefore, more sophisticated algorithms for understanding the context of both text and image may open rooms for improvement in content censorship to build a more efficient censorship system. In this paper, we evaluate existing LLM-based content moderation solutions such as OpenAI moderation model and Llama-Guard3 and study their capabilities to detect sensitive contents. Additionally, we explore recent LLMs such as GPT, Gemini, and Llama in identifying inappropriate contents across media outlets. Various textual and visual datasets like X tweets, Amazon reviews, news articles, human photos, cartoons, sketches, and violence videos have been utilized for evaluation and comparison. The results demonstrate that LLMs outperform traditional techniques by achieving higher accuracy and lower false positive and false negative rates. This highlights the potential to integrate LLMs into websites, social media platforms, and video-sharing services for regulatory and content moderation purposes.
* 55 pages, 16 figures
Via
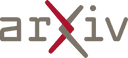