Topic:Time Series Analysis
What is Time Series Analysis? Time series analysis comprises statistical methods for analyzing a sequence of data points collected over an interval of time to identify interesting patterns and trends.
Papers and Code
Mar 24, 2025
Abstract:This work presents a novel framework for time series analysis using entropic measures based on the kernel density estimate (KDE) of the time series' Takens' embeddings. Using this framework we introduce two distinct analytical tools: (1) a multi-scale KDE entropy metric, denoted as $\Delta\text{KE}$, which quantifies the evolution of time series complexity across different scales by measuring certain entropy changes, and (2) a sliding baseline method that employs the Kullback-Leibler (KL) divergence to detect changes in time series dynamics through changes in KDEs. The $\Delta{\rm KE}$ metric offers insights into the information content and ``unfolding'' properties of the time series' embedding related to dynamical systems, while the KL divergence-based approach provides a noise and outlier robust approach for identifying time series change points (injections in RF signals, e.g.). We demonstrate the versatility and effectiveness of these tools through a set of experiments encompassing diverse domains. In the space of radio frequency (RF) signal processing, we achieve accurate detection of signal injections under varying noise and interference conditions. Furthermore, we apply our methodology to electrocardiography (ECG) data, successfully identifying instances of ventricular fibrillation with high accuracy. Finally, we demonstrate the potential of our tools for dynamic state detection by accurately identifying chaotic regimes within an intermittent signal. These results show the broad applicability of our framework for extracting meaningful insights from complex time series data across various scientific disciplines.
Via
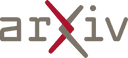
Mar 17, 2025
Abstract:Multi-modal time series analysis has recently emerged as a prominent research area in data mining, driven by the increasing availability of diverse data modalities, such as text, images, and structured tabular data from real-world sources. However, effective analysis of multi-modal time series is hindered by data heterogeneity, modality gap, misalignment, and inherent noise. Recent advancements in multi-modal time series methods have exploited the multi-modal context via cross-modal interactions based on deep learning methods, significantly enhancing various downstream tasks. In this tutorial and survey, we present a systematic and up-to-date overview of multi-modal time series datasets and methods. We first state the existing challenges of multi-modal time series analysis and our motivations, with a brief introduction of preliminaries. Then, we summarize the general pipeline and categorize existing methods through a unified cross-modal interaction framework encompassing fusion, alignment, and transference at different levels (\textit{i.e.}, input, intermediate, output), where key concepts and ideas are highlighted. We also discuss the real-world applications of multi-modal analysis for both standard and spatial time series, tailored to general and specific domains. Finally, we discuss future research directions to help practitioners explore and exploit multi-modal time series. The up-to-date resources are provided in the GitHub repository: https://github.com/UConn-DSIS/Multi-modal-Time-Series-Analysis
Via
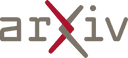
Mar 29, 2025
Abstract:Cryptocurrencies have transformed financial markets with their innovative blockchain technology and volatile price movements, presenting both challenges and opportunities for predictive analytics. Ethereum, being one of the leading cryptocurrencies, has experienced significant market fluctuations, making its price prediction an attractive yet complex problem. This paper presents a comprehensive study on the effectiveness of Large Language Models (LLMs) in predicting Ethereum prices for short-term and few-shot forecasting scenarios. The main challenge in training models for time series analysis is the lack of data. We address this by leveraging a novel approach that adapts existing pre-trained LLMs on natural language or images from billions of tokens to the unique characteristics of Ethereum price time series data. Through thorough experimentation and comparison with traditional and contemporary models, our results demonstrate that selectively freezing certain layers of pre-trained LLMs achieves state-of-the-art performance in this domain. This approach consistently surpasses benchmarks across multiple metrics, including Mean Squared Error (MSE), Mean Absolute Error (MAE), and Root Mean Squared Error (RMSE), demonstrating its effectiveness and robustness. Our research not only contributes to the existing body of knowledge on LLMs but also provides practical insights in the cryptocurrency prediction domain. The adaptability of pre-trained LLMs to handle the nature of Ethereum prices suggests a promising direction for future research, potentially including the integration of sentiment analysis to further refine forecasting accuracy.
Via
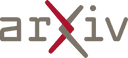
Apr 14, 2025
Abstract:Clinical case reports encode rich, temporal patient trajectories that are often underexploited by traditional machine learning methods relying on structured data. In this work, we introduce the forecasting problem from textual time series, where timestamped clinical findings--extracted via an LLM-assisted annotation pipeline--serve as the primary input for prediction. We systematically evaluate a diverse suite of models, including fine-tuned decoder-based large language models and encoder-based transformers, on tasks of event occurrence prediction, temporal ordering, and survival analysis. Our experiments reveal that encoder-based models consistently achieve higher F1 scores and superior temporal concordance for short- and long-horizon event forecasting, while fine-tuned masking approaches enhance ranking performance. In contrast, instruction-tuned decoder models demonstrate a relative advantage in survival analysis, especially in early prognosis settings. Our sensitivity analyses further demonstrate the importance of time ordering, which requires clinical time series construction, as compared to text ordering, the format of the text inputs that LLMs are classically trained on. This highlights the additional benefit that can be ascertained from time-ordered corpora, with implications for temporal tasks in the era of widespread LLM use.
* Machine Learning for Healthcare (MLHC 2025)
Via
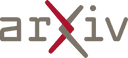
Mar 10, 2025
Abstract:With the recent development and advancement of Transformer and MLP architectures, significant strides have been made in time series analysis. Conversely, the performance of Convolutional Neural Networks (CNNs) in time series analysis has fallen short of expectations, diminishing their potential for future applications. Our research aims to enhance the representational capacity of Convolutional Neural Networks (CNNs) in time series analysis by introducing novel perspectives and design innovations. To be specific, We introduce a novel time series reshaping technique that considers the inter-patch, intra-patch, and cross-variable dimensions. Consequently, we propose TVNet, a dynamic convolutional network leveraging a 3D perspective to employ time series analysis. TVNet retains the computational efficiency of CNNs and achieves state-of-the-art results in five key time series analysis tasks, offering a superior balance of efficiency and performance over the state-of-the-art Transformer-based and MLP-based models. Additionally, our findings suggest that TVNet exhibits enhanced transferability and robustness. Therefore, it provides a new perspective for applying CNN in advanced time series analysis tasks.
* ICLR 2025
Via
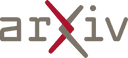
Mar 14, 2025
Abstract:Time series analysis is crucial for understanding dynamics of complex systems. Recent advances in foundation models have led to task-agnostic Time Series Foundation Models (TSFMs) and Large Language Model-based Time Series Models (TSLLMs), enabling generalized learning and integrating contextual information. However, their success depends on large, diverse, and high-quality datasets, which are challenging to build due to regulatory, diversity, quality, and quantity constraints. Synthetic data emerge as a viable solution, addressing these challenges by offering scalable, unbiased, and high-quality alternatives. This survey provides a comprehensive review of synthetic data for TSFMs and TSLLMs, analyzing data generation strategies, their role in model pretraining, fine-tuning, and evaluation, and identifying future research directions.
Via
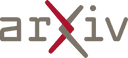
Mar 14, 2025
Abstract:Time series analysis (TSA) is a longstanding research topic in the data mining community and has wide real-world significance. Compared to "richer" modalities such as language and vision, which have recently experienced explosive development and are densely connected, the time-series modality remains relatively underexplored and isolated. We notice that many recent TSA works have formed a new research field, i.e., Multiple Modalities for TSA (MM4TSA). In general, these MM4TSA works follow a common motivation: how TSA can benefit from multiple modalities. This survey is the first to offer a comprehensive review and a detailed outlook for this emerging field. Specifically, we systematically discuss three benefits: (1) reusing foundation models of other modalities for efficient TSA, (2) multimodal extension for enhanced TSA, and (3) cross-modality interaction for advanced TSA. We further group the works by the introduced modality type, including text, images, audio, tables, and others, within each perspective. Finally, we identify the gaps with future opportunities, including the reused modalities selections, heterogeneous modality combinations, and unseen tasks generalizations, corresponding to the three benefits. We release an up-to-date GitHub repository that includes key papers and resources.
Via
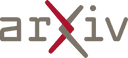
Apr 27, 2025
Abstract:High-quality preference data is essential for aligning foundation models with human values through preference learning. However, manual annotation of such data is often time-consuming and costly. Recent methods often adopt a self-rewarding approach, where the target model generates and annotates its own preference data, but this can lead to inaccuracies since the reward model shares weights with the target model, thereby amplifying inherent biases. To address these issues, we propose Anyprefer, a framework designed to synthesize high-quality preference data for aligning the target model. Anyprefer frames the data synthesis process as a cooperative two-player Markov Game, where the target model and the judge model collaborate together. Here, a series of external tools are introduced to assist the judge model in accurately rewarding the target model's responses, mitigating biases in the rewarding process. In addition, a feedback mechanism is introduced to optimize prompts for both models, enhancing collaboration and improving data quality. The synthesized data is compiled into a new preference dataset, Anyprefer-V1, consisting of 58K high-quality preference pairs. Extensive experiments show that Anyprefer significantly improves model alignment performance across four main applications, covering 21 datasets, achieving average improvements of 18.55% in five natural language generation datasets, 3.66% in nine vision-language understanding datasets, 30.05% in three medical image analysis datasets, and 16.00% in four visuo-motor control tasks.
Via
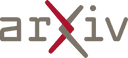
Apr 25, 2025
Abstract:Traditional human reliability analysis (HRA) methods, such as IDHEAS-ECA, rely on expert judgment and empirical rules that often overlook the cognitive underpinnings of human error. Moreover, conducting human-in-the-loop experiments for advanced nuclear power plants is increasingly impractical due to novel interfaces and limited operational data. This study proposes a cognitive-mechanistic framework (COGMIF) that enhances the IDHEAS-ECA methodology by integrating an ACT-R-based human digital twin (HDT) with TimeGAN-augmented simulation. The ACT-R model simulates operator cognition, including memory retrieval, goal-directed procedural reasoning, and perceptual-motor execution, under high-fidelity scenarios derived from a high-temperature gas-cooled reactor (HTGR) simulator. To overcome the resource constraints of large-scale cognitive modeling, TimeGAN is trained on ACT-R-generated time-series data to produce high-fidelity synthetic operator behavior datasets. These simulations are then used to drive IDHEAS-ECA assessments, enabling scalable, mechanism-informed estimation of human error probabilities (HEPs). Comparative analyses with SPAR-H and sensitivity assessments demonstrate the robustness and practical advantages of the proposed COGMIF. Finally, procedural features are mapped onto a Bayesian network to quantify the influence of contributing factors, revealing key drivers of operational risk. This work offers a credible and computationally efficient pathway to integrate cognitive theory into industrial HRA practices.
Via
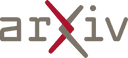
Mar 17, 2025
Abstract:Climate change has increased the vulnerability of forests to insect-related damage, resulting in widespread forest loss in Central Europe and highlighting the need for effective, continuous monitoring systems. Remote sensing based forest health monitoring, oftentimes, relies on supervised machine learning algorithms that require labeled training data. Monitoring temporal patterns through time series analysis offers a potential alternative for earlier detection of disturbance but requires substantial storage resources. This study investigates the potential of a Deep Learning algorithm based on a Long Short Term Memory (LSTM) Autoencoder for the detection of anomalies in forest health (e.g. bark beetle outbreaks), utilizing Sentinel-2 time series data. This approach is an alternative to supervised machine learning methods, avoiding the necessity for labeled training data. Furthermore, it is more memory-efficient than other time series analysis approaches, as a robust model can be created using only a 26-week-long time series as input. In this study, we monitored pure stands of spruce in Thuringia, Germany, over a 7-year period from 2018 to the end of 2024. Our best model achieved a detection accuracy of 87% on test data and was able to detect 61% of all anomalies at a very early stage (more than a month before visible signs of forest degradation). Compared to another widely used time series break detection algorithm - BFAST (Breaks For Additive Season and Trend), our approach consistently detected higher percentage of anomalies at an earlier stage. These findings suggest that LSTM-based Autoencoders could provide a promising, resource-efficient approach to forest health monitoring, enabling more timely responses to emerging threats.
* 24 pages, 18 figures, submitted to IEEE: Journal of Selected Topics
in Applied Earth Observations and Remote Sensing
Via
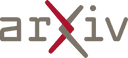