Topic:Photoplethysmography
What is Photoplethysmography? Photoplethysmography (PPG) is a non-invasive optical technique used to measure blood volume changes in the microvascular bed of tissue.
Papers and Code
Jun 11, 2025
Abstract:Remote photoplethysmography (rPPG) enables non-contact, continuous monitoring of physiological signals and offers a practical alternative to traditional health sensing methods. Although rPPG is promising for daily health monitoring, its application in long-term personal care scenarios, such as mirror-facing routines in high-altitude environments, remains challenging due to ambient lighting variations, frequent occlusions from hand movements, and dynamic facial postures. To address these challenges, we present LADH (Long-term Altitude Daily Health), the first long-term rPPG dataset containing 240 synchronized RGB and infrared (IR) facial videos from 21 participants across five common personal care scenarios, along with ground-truth PPG, respiration, and blood oxygen signals. Our experiments demonstrate that combining RGB and IR video inputs improves the accuracy and robustness of non-contact physiological monitoring, achieving a mean absolute error (MAE) of 4.99 BPM in heart rate estimation. Furthermore, we find that multi-task learning enhances performance across multiple physiological indicators simultaneously. Dataset and code are open at https://github.com/McJackTang/FusionVitals.
Via
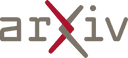
May 28, 2025
Abstract:Cardiovascular signals such as photoplethysmography (PPG), electrocardiography (ECG), and blood pressure (BP) are inherently correlated and complementary, together reflecting the health of cardiovascular system. However, their joint utilization in real-time monitoring is severely limited by diverse acquisition challenges from noisy wearable recordings to burdened invasive procedures. Here we propose UniCardio, a multi-modal diffusion transformer that reconstructs low-quality signals and synthesizes unrecorded signals in a unified generative framework. Its key innovations include a specialized model architecture to manage the signal modalities involved in generation tasks and a continual learning paradigm to incorporate varying modality combinations. By exploiting the complementary nature of cardiovascular signals, UniCardio clearly outperforms recent task-specific baselines in signal denoising, imputation, and translation. The generated signals match the performance of ground-truth signals in detecting abnormal health conditions and estimating vital signs, even in unseen domains, while ensuring interpretability for human experts. These advantages position UniCardio as a promising avenue for advancing AI-assisted healthcare.
Via
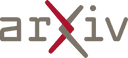
May 19, 2025
Abstract:Cardiovascular diseases remain a leading cause of mortality and disability. The convenient measurement of cardiovascular health using smart systems is therefore a key enabler to foster accurate and early detection and diagnosis of cardiovascular diseases and it require accessing a correct pulse morphology similar to arterial pressure wave. This paper investigates the comparison between different sensor modalities, such as mmWave and photoplethysmography from the same physiological site and reference continuous non-invasive blood pressure devide. We have developed a hardware prototype and established an experiment consist of 23 test participants. Both mmWave and PPG are capable of detecting inter-beat intervals. mmWave is providing more accurate arterial pulse waveform than green photoplethysmography.
Via
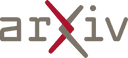
May 16, 2025
Abstract:Photoplethysmography (PPG) signals encode information about relative changes in blood volume that can be used to assess various aspects of cardiac health non-invasively, e.g.\ to detect atrial fibrillation (AF) or predict blood pressure (BP). Deep networks are well-equipped to handle the large quantities of data acquired from wearable measurement devices. However, they lack interpretability and are prone to overfitting, leaving considerable risk for poor performance on unseen data and misdiagnosis. Here, we describe the use of two scalable uncertainty quantification techniques: Monte Carlo Dropout and the recently proposed Improved Variational Online Newton. These techniques are used to assess the trustworthiness of models trained to perform AF classification and BP regression from raw PPG time series. We find that the choice of hyperparameters has a considerable effect on the predictive performance of the models and on the quality and composition of predicted uncertainties. E.g. the stochasticity of the model parameter sampling determines the proportion of the total uncertainty that is aleatoric, and has varying effects on predictive performance and calibration quality dependent on the chosen uncertainty quantification technique and the chosen expression of uncertainty. We find significant discrepancy in the quality of uncertainties over the predicted classes, emphasising the need for a thorough evaluation protocol that assesses local and adaptive calibration. This work suggests that the choice of hyperparameters must be carefully tuned to balance predictive performance and calibration quality, and that the optimal parameterisation may vary depending on the chosen expression of uncertainty.
Via
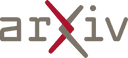
May 11, 2025
Abstract:Remote physiological sensing using camera-based technologies offers transformative potential for non-invasive vital sign monitoring across healthcare and human-computer interaction domains. Although deep learning approaches have advanced the extraction of physiological signals from video data, existing methods have not been sufficiently assessed for their robustness to domain shifts. These shifts in remote physiological sensing include variations in ambient conditions, camera specifications, head movements, facial poses, and physiological states which often impact real-world performance significantly. Cross-dataset evaluation provides an objective measure to assess generalization capabilities across these domain shifts. We introduce Target Signal Constrained Factorization module (TSFM), a novel multidimensional attention mechanism that explicitly incorporates physiological signal characteristics as factorization constraints, allowing more precise feature extraction. Building on this innovation, we present MMRPhys, an efficient dual-branch 3D-CNN architecture designed for simultaneous multitask estimation of photoplethysmography (rPPG) and respiratory (rRSP) signals from multimodal RGB and thermal video inputs. Through comprehensive cross-dataset evaluation on five benchmark datasets, we demonstrate that MMRPhys with TSFM significantly outperforms state-of-the-art methods in generalization across domain shifts for rPPG and rRSP estimation, while maintaining a minimal inference latency suitable for real-time applications. Our approach establishes new benchmarks for robust multitask and multimodal physiological sensing and offers a computationally efficient framework for practical deployment in unconstrained environments. The web browser-based application featuring on-device real-time inference of MMRPhys model is available at https://physiologicailab.github.io/mmrphys-live
* 25 pages, 6 figures
Via
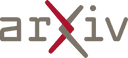
May 08, 2025
Abstract:Smart rings offer a convenient way to continuously and unobtrusively monitor cardiovascular physiological signals. However, a gap remains between the ring hardware and reliable methods for estimating cardiovascular parameters, partly due to the lack of publicly available datasets and standardized analysis tools. In this work, we present $\tau$-Ring, the first open-source ring-based dataset designed for cardiovascular physiological sensing. The dataset comprises photoplethysmography signals (infrared and red channels) and 3-axis accelerometer data collected from two rings (reflective and transmissive optical paths), with 28.21 hours of raw data from 34 subjects across seven activities. $\tau$-Ring encompasses both stationary and motion scenarios, as well as stimulus-evoked abnormal physiological states, annotated with four ground-truth labels: heart rate, respiratory rate, oxygen saturation, and blood pressure. Using our proposed RingTool toolkit, we evaluated three widely-used physics-based methods and four cutting-edge deep learning approaches. Our results show superior performance compared to commercial rings, achieving best MAE values of 5.18 BPM for heart rate, 2.98 BPM for respiratory rate, 3.22\% for oxygen saturation, and 13.33/7.56 mmHg for systolic/diastolic blood pressure estimation. The open-sourced dataset and toolkit aim to foster further research and community-driven advances in ring-based cardiovascular health sensing.
Via
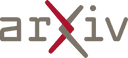
May 06, 2025
Abstract:Remote photoplethysmography (rPPG) enables non-contact physiological measurement but remains highly susceptible to illumination changes, motion artifacts, and limited temporal modeling. Large Language Models (LLMs) excel at capturing long-range dependencies, offering a potential solution but struggle with the continuous, noise-sensitive nature of rPPG signals due to their text-centric design. To bridge this gap, we introduce PhysLLM, a collaborative optimization framework that synergizes LLMs with domain-specific rPPG components. Specifically, the Text Prototype Guidance (TPG) strategy is proposed to establish cross-modal alignment by projecting hemodynamic features into LLM-interpretable semantic space, effectively bridging the representational gap between physiological signals and linguistic tokens. Besides, a novel Dual-Domain Stationary (DDS) Algorithm is proposed for resolving signal instability through adaptive time-frequency domain feature re-weighting. Finally, rPPG task-specific cues systematically inject physiological priors through physiological statistics, environmental contextual answering, and task description, leveraging cross-modal learning to integrate both visual and textual information, enabling dynamic adaptation to challenging scenarios like variable illumination and subject movements. Evaluation on four benchmark datasets, PhysLLM achieves state-of-the-art accuracy and robustness, demonstrating superior generalization across lighting variations and motion scenarios.
Via
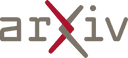
May 06, 2025
Abstract:3D mask presentation attack detection is crucial for protecting face recognition systems against the rising threat of 3D mask attacks. While most existing methods utilize multimodal features or remote photoplethysmography (rPPG) signals to distinguish between real faces and 3D masks, they face significant challenges, such as the high costs associated with multimodal sensors and limited generalization ability. Detection-related text descriptions offer concise, universal information and are cost-effective to obtain. However, the potential of vision-language multimodal features for 3D mask presentation attack detection remains unexplored. In this paper, we propose a novel knowledge-based prompt learning framework to explore the strong generalization capability of vision-language models for 3D mask presentation attack detection. Specifically, our approach incorporates entities and triples from knowledge graphs into the prompt learning process, generating fine-grained, task-specific explicit prompts that effectively harness the knowledge embedded in pre-trained vision-language models. Furthermore, considering different input images may emphasize distinct knowledge graph elements, we introduce a visual-specific knowledge filter based on an attention mechanism to refine relevant elements according to the visual context. Additionally, we leverage causal graph theory insights into the prompt learning process to further enhance the generalization ability of our method. During training, a spurious correlation elimination paradigm is employed, which removes category-irrelevant local image patches using guidance from knowledge-based text features, fostering the learning of generalized causal prompts that align with category-relevant local patches. Experimental results demonstrate that the proposed method achieves state-of-the-art intra- and cross-scenario detection performance on benchmark datasets.
Via
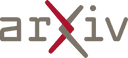
May 02, 2025
Abstract:Camera-based monitoring of Pulse Rate (PR) enables continuous and unobtrusive assessment of driver's state, allowing estimation of fatigue or stress that could impact traffic safety. Commonly used wearable Photoplethysmography (PPG) sensors, while effective, suffer from motion artifacts and user discomfort. This study explores the feasibility of non-contact PR assessment using facial video recordings captured by a Red, Green, and Blue (RGB) camera in a driving simulation environment. The proposed approach detects subtle skin color variations due to blood flow and compares extracted PR values against reference measurements from a wearable wristband Empatica E4. We evaluate the impact of Eulerian Video Magnification (EVM) on signal quality and assess statistical differences in PR between age groups. Data obtained from 80 recordings from 64 healthy subjects covering a PR range of 45-160 bpm are analyzed, and signal extraction accuracy is quantified using metrics, such as Mean Absolute Error (MAE) and Root Mean Square Error (RMSE). Results show that EVM slightly improves PR estimation accuracy, reducing MAE from 6.48 bpm to 5.04 bpm and RMSE from 7.84 bpm to 6.38 bpm. A statistically significant difference is found between older and younger groups with both video-based and ground truth evaluation procedures. Additionally, we discuss Empatica E4 bias and its potential impact on the overall assessment of contact measurements. Altogether the findings demonstrate the feasibility of camera-based PR monitoring in dynamic environments and its potential integration into driving simulators for real-time physiological assessment.
* 6 figures and one table
Via
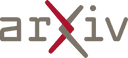
Apr 29, 2025
Abstract:Artefacts compromise clinical decision-making in the use of medical time series. Pulsatile waveforms offer probabilities for accurate artefact detection, yet most approaches rely on supervised manners and overlook patient-level distribution shifts. To address these issues, we introduce a generalised label-free framework, GenClean, for real-time artefact cleaning and leverage an in-house dataset of 180,000 ten-second arterial blood pressure (ABP) samples for training. We first investigate patient-level generalisation, demonstrating robust performances under both intra- and inter-patient distribution shifts. We further validate its effectiveness through challenging cross-disease cohort experiments on the MIMIC-III database. Additionally, we extend our method to photoplethysmography (PPG), highlighting its applicability to diverse medical pulsatile signals. Finally, its integration into ICM+, a clinical research monitoring software, confirms the real-time feasibility of our framework, emphasising its practical utility in continuous physiological monitoring. This work provides a foundational step toward precision medicine in improving the reliability of high-resolution medical time series analysis
Via
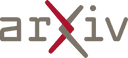