Topic:3D Face Reconstruction
What is 3D Face Reconstruction? 3D face reconstruction is the process of creating a 3D model of a person's face from 2D images or videos.
Papers and Code
May 01, 2025
Abstract:We introduce CLR-Wire, a novel framework for 3D curve-based wireframe generation that integrates geometry and topology into a unified Continuous Latent Representation. Unlike conventional methods that decouple vertices, edges, and faces, CLR-Wire encodes curves as Neural Parametric Curves along with their topological connectivity into a continuous and fixed-length latent space using an attention-driven variational autoencoder (VAE). This unified approach facilitates joint learning and generation of both geometry and topology. To generate wireframes, we employ a flow matching model to progressively map Gaussian noise to these latents, which are subsequently decoded into complete 3D wireframes. Our method provides fine-grained modeling of complex shapes and irregular topologies, and supports both unconditional generation and generation conditioned on point cloud or image inputs. Experimental results demonstrate that, compared with state-of-the-art generative approaches, our method achieves substantial improvements in accuracy, novelty, and diversity, offering an efficient and comprehensive solution for CAD design, geometric reconstruction, and 3D content creation.
* SIGGRAPH 2025 (Patent Protected); Project page:
https://vcc.tech/research/2025/CLRWire
Via
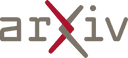
Apr 26, 2025
Abstract:3D face reconstruction (3DFR) algorithms are based on specific assumptions tailored to the limits and characteristics of the different application scenarios. In this study, we investigate how multiple state-of-the-art 3DFR algorithms can be used to generate a better representation of subjects, with the final goal of improving the performance of face recognition systems in challenging uncontrolled scenarios. We also explore how different parametric and non-parametric score-level fusion methods can exploit the unique strengths of multiple 3DFR algorithms to enhance biometric recognition robustness. With this goal, we propose a comprehensive analysis of several face recognition systems across diverse conditions, such as varying distances and camera setups, intra-dataset and cross-dataset, to assess the robustness of the proposed ensemble method. The results demonstrate that the distinct information provided by different 3DFR algorithms can alleviate the problem of generalizing over multiple application scenarios. In addition, the present study highlights the potential of advanced fusion strategies to enhance the reliability of 3DFR-based face recognition systems, providing the research community with key insights to exploit them in real-world applications effectively. Although the experiments are carried out in a specific face verification setup, our proposed fusion-based 3DFR methods may be applied to other tasks around face biometrics that are not strictly related to identity recognition.
Via
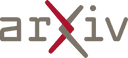
Apr 27, 2025
Abstract:Neural implicit representations have had a significant impact on simultaneous localization and mapping (SLAM) by enabling robots to build continuous, differentiable, and high-fidelity 3D maps from sensor data. However, as the scale and complexity of the environment increase, neural SLAM approaches face renewed challenges in the back-end optimization process to keep up with runtime requirements and maintain global consistency. We introduce MISO, a hierarchical optimization approach that leverages multiresolution submaps to achieve efficient and scalable neural implicit reconstruction. For local SLAM within each submap, we develop a hierarchical optimization scheme with learned initialization that substantially reduces the time needed to optimize the implicit submap features. To correct estimation drift globally, we develop a hierarchical method to align and fuse the multiresolution submaps, leading to substantial acceleration by avoiding the need to decode the full scene geometry. MISO significantly improves computational efficiency and estimation accuracy of neural signed distance function (SDF) SLAM on large-scale real-world benchmarks.
* To appear at RSS 2025 (15 pages, 11 figures)
Via
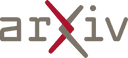
Apr 23, 2025
Abstract:Robust GNSS positioning in urban environments is still plagued by multipath effects, particularly due to the complex signal propagation induced by ubiquitous surfaces with varied radio frequency reflectivities. Current 3D Mapping Aided (3DMA) GNSS techniques show great potentials in mitigating multipath but face a critical trade-off between computational efficiency and modeling accuracy. Most approaches often rely on offline outdated or oversimplified 3D maps, while real-time LiDAR-based reconstruction boasts high accuracy, it is problematic in low laser reflectivity conditions; camera 3DMA is a good candidate to balance accuracy and efficiency but current methods suffer from extremely low reconstruction speed, a far cry from real-time multipath-mitigated navigation. This paper proposes an accelerated framework incorporating camera multi-view stereo (MVS) reconstruction and ray tracing. By hypothesizing on surface textures, an orthogonal visual feature fusion framework is proposed, which robustly addresses both texture-rich and texture-poor surfaces, lifting off the reflectivity challenges in visual reconstruction. A polygonal surface modeling scheme is further integrated to accurately delineate complex building boundaries, enhancing the reconstruction granularity. To avoid excessively accurate reconstruction, reprojected point cloud multi-plane fitting and two complexity control strategies are proposed, thus improving upon multipath estimation speed. Experiments were conducted in Lujiazui, Shanghai, a typical multipath-prone district. The results show that the method achieves an average reconstruction accuracy of 2.4 meters in dense urban environments featuring glass curtain wall structures, a traditionally tough case for reconstruction, and achieves a ray-tracing-based multipath correction rate of 30 image frames per second, 10 times faster than the contemporary benchmarks.
Via
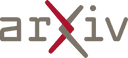
Apr 21, 2025
Abstract:Digital modeling and reconstruction of human faces serve various applications. However, its availability is often hindered by the requirements of data capturing devices, manual labor, and suitable actors. This situation restricts the diversity, expressiveness, and control over the resulting models. This work aims to demonstrate that a semantically controllable generative network can provide enhanced control over the digital face modeling process. To enhance diversity beyond the limited human faces scanned in a controlled setting, we introduce a novel data generation pipeline that creates a high-quality 3D face database using a pre-trained diffusion model. Our proposed normalization module converts synthesized data from the diffusion model into high-quality scanned data. Using the 44,000 face models we obtained, we further developed an efficient GAN-based generator. This generator accepts semantic attributes as input, and generates geometry and albedo. It also allows continuous post-editing of attributes in the latent space. Our asset refinement component subsequently creates physically-based facial assets. We introduce a comprehensive system designed for creating and editing high-quality face assets. Our proposed model has undergone extensive experiment, comparison and evaluation. We also integrate everything into a web-based interactive tool. We aim to make this tool publicly available with the release of the paper.
Via
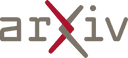
Apr 17, 2025
Abstract:Although 3D Gaussian Splatting (3DGS) has revolutionized 3D reconstruction, it still faces challenges such as aliasing, projection artifacts, and view inconsistencies, primarily due to the simplification of treating splats as 2D entities. We argue that incorporating full 3D evaluation of Gaussians throughout the 3DGS pipeline can effectively address these issues while preserving rasterization efficiency. Specifically, we introduce an adaptive 3D smoothing filter to mitigate aliasing and present a stable view-space bounding method that eliminates popping artifacts when Gaussians extend beyond the view frustum. Furthermore, we promote tile-based culling to 3D with screen-space planes, accelerating rendering and reducing sorting costs for hierarchical rasterization. Our method achieves state-of-the-art quality on in-distribution evaluation sets and significantly outperforms other approaches for out-of-distribution views. Our qualitative evaluations further demonstrate the effective removal of aliasing, distortions, and popping artifacts, ensuring real-time, artifact-free rendering.
Via
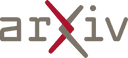
Apr 17, 2025
Abstract:Visuomotor policies learned from teleoperated demonstrations face challenges such as lengthy data collection, high costs, and limited data diversity. Existing approaches address these issues by augmenting image observations in RGB space or employing Real-to-Sim-to-Real pipelines based on physical simulators. However, the former is constrained to 2D data augmentation, while the latter suffers from imprecise physical simulation caused by inaccurate geometric reconstruction. This paper introduces RoboSplat, a novel method that generates diverse, visually realistic demonstrations by directly manipulating 3D Gaussians. Specifically, we reconstruct the scene through 3D Gaussian Splatting (3DGS), directly edit the reconstructed scene, and augment data across six types of generalization with five techniques: 3D Gaussian replacement for varying object types, scene appearance, and robot embodiments; equivariant transformations for different object poses; visual attribute editing for various lighting conditions; novel view synthesis for new camera perspectives; and 3D content generation for diverse object types. Comprehensive real-world experiments demonstrate that RoboSplat significantly enhances the generalization of visuomotor policies under diverse disturbances. Notably, while policies trained on hundreds of real-world demonstrations with additional 2D data augmentation achieve an average success rate of 57.2%, RoboSplat attains 87.8% in one-shot settings across six types of generalization in the real world.
* Published at Robotics: Science and Systems (RSS) 2025
Via
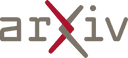
Apr 16, 2025
Abstract:Accurate, real-time 3D reconstruction of human heads from monocular images and videos underlies numerous visual applications. As 3D ground truth data is hard to come by at scale, previous methods have sought to learn from abundant 2D videos in a self-supervised manner. Typically, this involves the use of differentiable mesh rendering, which is effective but faces limitations. To improve on this, we propose SHeaP (Self-supervised Head Geometry Predictor Learned via 2D Gaussians). Given a source image, we predict a 3DMM mesh and a set of Gaussians that are rigged to this mesh. We then reanimate this rigged head avatar to match a target frame, and backpropagate photometric losses to both the 3DMM and Gaussian prediction networks. We find that using Gaussians for rendering substantially improves the effectiveness of this self-supervised approach. Training solely on 2D data, our method surpasses existing self-supervised approaches in geometric evaluations on the NoW benchmark for neutral faces and a new benchmark for non-neutral expressions. Our method also produces highly expressive meshes, outperforming state-of-the-art in emotion classification.
* For video demonstrations and additional materials please see
https://nlml.github.io/sheap/
Via
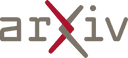
Apr 17, 2025
Abstract:Photorealistic avatars have become essential for immersive applications in virtual reality (VR) and augmented reality (AR), enabling lifelike interactions in areas such as training simulations, telemedicine, and virtual collaboration. These avatars bridge the gap between the physical and digital worlds, improving the user experience through realistic human representation. However, existing avatar creation techniques face significant challenges, including high costs, long creation times, and limited utility in virtual applications. Manual methods, such as MetaHuman, require extensive time and expertise, while automatic approaches, such as NeRF-based pipelines often lack efficiency, detailed facial expression fidelity, and are unable to be rendered at a speed sufficent for real-time applications. By involving several cutting-edge modern techniques, we introduce an end-to-end 3D Gaussian Splatting (3DGS) avatar creation pipeline that leverages monocular video input to create a scalable and efficient photorealistic avatar directly compatible with the Unity game engine. Our pipeline incorporates a novel Gaussian splatting technique with customized preprocessing that enables the user of "in the wild" monocular video capture, detailed facial expression reconstruction and embedding within a fully rigged avatar model. Additionally, we present a Unity-integrated Gaussian Splatting Avatar Editor, offering a user-friendly environment for VR/AR application development. Experimental results validate the effectiveness of our preprocessing pipeline in standardizing custom data for 3DGS training and demonstrate the versatility of Gaussian avatars in Unity, highlighting the scalability and practicality of our approach.
Via
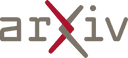
Apr 12, 2025
Abstract:Recently, reconstructing scenes from a single panoramic image using advanced 3D Gaussian Splatting (3DGS) techniques has attracted growing interest. Panoramic images offer a 360$\times$ 180 field of view (FoV), capturing the entire scene in a single shot. However, panoramic images introduce severe distortion, making it challenging to render 3D Gaussians into 2D distorted equirectangular space directly. Converting equirectangular images to cubemap projections partially alleviates this problem but introduces new challenges, such as projection distortion and discontinuities across cube-face boundaries. To address these limitations, we present a novel framework, named TPGS, to bridge continuous panoramic 3D scene reconstruction with perspective Gaussian splatting. Firstly, we introduce a Transition Plane between adjacent cube faces to enable smoother transitions in splatting directions and mitigate optimization ambiguity in the boundary region. Moreover, an intra-to-inter face optimization strategy is proposed to enhance local details and restore visual consistency across cube-face boundaries. Specifically, we optimize 3D Gaussians within individual cube faces and then fine-tune them in the stitched panoramic space. Additionally, we introduce a spherical sampling technique to eliminate visible stitching seams. Extensive experiments on indoor and outdoor, egocentric, and roaming benchmark datasets demonstrate that our approach outperforms existing state-of-the-art methods. Code and models will be available at https://github.com/zhijieshen-bjtu/TPGS.
Via
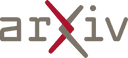