What is autonomous cars? Autonomous cars are self-driving vehicles that use artificial intelligence (AI) and sensors to navigate and operate without human intervention, using high-resolution cameras and lidars that detect what happens in the car's immediate surroundings. They have the potential to revolutionize transportation by improving safety, efficiency, and accessibility.
Papers and Code
Oct 10, 2024
Abstract:The generation of high-quality 3D car assets is essential for various applications, including video games, autonomous driving, and virtual reality. Current 3D generation methods utilizing NeRF or 3D-GS as representations for 3D objects, generate a Lambertian object under fixed lighting and lack separated modelings for material and global illumination. As a result, the generated assets are unsuitable for relighting under varying lighting conditions, limiting their applicability in downstream tasks. To address this challenge, we propose a novel relightable 3D object generative framework that automates the creation of 3D car assets, enabling the swift and accurate reconstruction of a vehicle's geometry, texture, and material properties from a single input image. Our approach begins with introducing a large-scale synthetic car dataset comprising over 1,000 high-precision 3D vehicle models. We represent 3D objects using global illumination and relightable 3D Gaussian primitives integrating with BRDF parameters. Building on this representation, we introduce a feed-forward model that takes images as input and outputs both relightable 3D Gaussians and global illumination parameters. Experimental results demonstrate that our method produces photorealistic 3D car assets that can be seamlessly integrated into road scenes with different illuminations, which offers substantial practical benefits for industrial applications.
Via
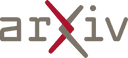
Oct 30, 2024
Abstract:Accurate vehicle detection is essential for the development of intelligent transportation systems, autonomous driving, and traffic monitoring. This paper presents a detailed analysis of YOLO11, the latest advancement in the YOLO series of deep learning models, focusing exclusively on vehicle detection tasks. Building upon the success of its predecessors, YOLO11 introduces architectural improvements designed to enhance detection speed, accuracy, and robustness in complex environments. Using a comprehensive dataset comprising multiple vehicle types-cars, trucks, buses, motorcycles, and bicycles we evaluate YOLO11's performance using metrics such as precision, recall, F1 score, and mean average precision (mAP). Our findings demonstrate that YOLO11 surpasses previous versions (YOLOv8 and YOLOv10) in detecting smaller and more occluded vehicles while maintaining a competitive inference time, making it well-suited for real-time applications. Comparative analysis shows significant improvements in the detection of complex vehicle geometries, further contributing to the development of efficient and scalable vehicle detection systems. This research highlights YOLO11's potential to enhance autonomous vehicle performance and traffic monitoring systems, offering insights for future developments in the field.
* 16 pages
Via
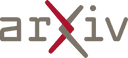
Nov 01, 2024
Abstract:This paper presents an autonomous method to address challenges arising from severe lighting conditions in machine vision applications that use event cameras. To manage these conditions, the research explores the built in potential of these cameras to adjust pixel functionality, named bias settings. As cars are driven at various times and locations, shifts in lighting conditions are unavoidable. Consequently, this paper utilizes the neuromorphic YOLO-based face tracking module of a driver monitoring system as the event-based application to study. The proposed method uses numerical metrics to continuously monitor the performance of the event-based application in real-time. When the application malfunctions, the system detects this through a drop in the metrics and automatically adjusts the event cameras bias values. The Nelder-Mead simplex algorithm is employed to optimize this adjustment, with finetuning continuing until performance returns to a satisfactory level. The advantage of bias optimization lies in its ability to handle conditions such as flickering or darkness without requiring additional hardware or software. To demonstrate the capabilities of the proposed system, it was tested under conditions where detecting human faces with default bias values was impossible. These severe conditions were simulated using dim ambient light and various flickering frequencies. Following the automatic and dynamic process of bias modification, the metrics for face detection significantly improved under all conditions. Autobiasing resulted in an increase in the YOLO confidence indicators by more than 33 percent for object detection and 37 percent for face detection highlighting the effectiveness of the proposed method.
* ECCV 2024 NeVi Workshop
Via
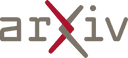
Sep 28, 2024
Abstract:Autonomous racing has rapidly gained research attention. Traditionally, racing cars rely on 2D LiDAR as their primary visual system. In this work, we explore the integration of an event camera with the existing system to provide enhanced temporal information. Our goal is to fuse the 2D LiDAR data with event data in an end-to-end learning framework for steering prediction, which is crucial for autonomous racing. To the best of our knowledge, this is the first study addressing this challenging research topic. We start by creating a multisensor dataset specifically for steering prediction. Using this dataset, we establish a benchmark by evaluating various SOTA fusion methods. Our observations reveal that existing methods often incur substantial computational costs. To address this, we apply low-rank techniques to propose a novel, efficient, and effective fusion design. We introduce a new fusion learning policy to guide the fusion process, enhancing robustness against misalignment. Our fusion architecture provides better steering prediction than LiDAR alone, significantly reducing the RMSE from 7.72 to 1.28. Compared to the second-best fusion method, our work represents only 11% of the learnable parameters while achieving better accuracy. The source code, dataset, and benchmark will be released to promote future research.
Via
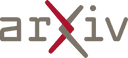
Oct 24, 2024
Abstract:This work presents the experiments and solution outline for our teams winning submission in the Learn To Race Autonomous Racing Virtual Challenge 2022 hosted by AIcrowd. The objective of the Learn-to-Race competition is to push the boundary of autonomous technology, with a focus on achieving the safety benefits of autonomous driving. In the description the competition is framed as a reinforcement learning (RL) challenge. We focused our initial efforts on implementation of Soft Actor Critic (SAC) variants. Our goal was to learn non-trivial control of the race car exclusively from visual and geometric features, directly mapping pixels to control actions. We made suitable modifications to the default reward policy aiming to promote smooth steering and acceleration control. The framework for the competition provided real time simulation, meaning a single episode (learning experience) is measured in minutes. Instead of pursuing parallelisation of episodes we opted to explore a more traditional approach in which the visual perception was processed (via learned operators) and fed into rule-based controllers. Such a system, while not as academically "attractive" as a pixels-to-actions approach, results in a system that requires less training, is more explainable, generalises better and is easily tuned and ultimately out-performed all other agents in the competition by a large margin.
Via
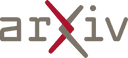
Aug 27, 2024
Abstract:This work aims to present a three-dimensional vehicle dynamics state estimation under varying signal quality. Few researchers have investigated the impact of three-dimensional road geometries on the state estimation and, thus, neglect road inclination and banking. Especially considering high velocities and accelerations, the literature does not address these effects. Therefore, we compare two- and three-dimensional state estimation schemes to outline the impact of road geometries. We use an Extended Kalman Filter with a point-mass motion model and extend it by an additional formulation of reference angles. Furthermore, virtual velocity measurements significantly improve the estimation of road angles and the vehicle's side slip angle. We highlight the importance of steady estimations for vehicle motion control algorithms and demonstrate the challenges of degraded signal quality and Global Navigation Satellite System dropouts. The proposed adaptive covariance facilitates a smooth estimation and enables stable controller behavior. The developed state estimation has been deployed on a high-speed autonomous race car at various racetracks. Our findings indicate that our approach outperforms state-of-the-art vehicle dynamics state estimators and an industry-grade Inertial Navigation System. Further studies are needed to investigate the performance under varying track conditions and on other vehicle types.
* This paper has been accepted at IROS 2024
Via
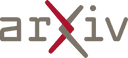
Jun 13, 2024
Abstract:This paper proposes a mini autonomous car to be used by the team UruBots for the 2024 FIRA Autonomous Cars Race Challenge. The vehicle is proposed focusing on a low cost and light weight setup. Powered by a Raspberry PI4 and with a total weight of 1.15 Kilograms, we show that our vehicle manages to race a track of approximately 13 meters in 11 seconds at the best evaluation that was carried out, with an average speed of 1.2m/s in average. That performance was achieved after training a convolutional neural network with 1500 samples for a total amount of 60 epochs. Overall, we believe that our vehicle are suited to perform at the FIRA Autonomous Cars Race Challenge 2024, helping the development of the field of study and the category in the competition.
* Team Description Paper for the FIRA RoboWorld Cup 2024
Via
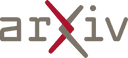
Nov 01, 2024
Abstract:Autonomous cooperative planning (ACP) is a promising technique to improve the efficiency and safety of multi-vehicle interactions for future intelligent transportation systems. However, realizing robust ACP is a challenge due to the aggregation of perception, motion, and communication uncertainties. This paper proposes a novel multi-uncertainty aware ACP (MUACP) framework that simultaneously accounts for multiple types of uncertainties via regularized cooperative model predictive control (RC-MPC). The regularizers and constraints for perception, motion, and communication are constructed according to the confidence levels, weather conditions, and outage probabilities, respectively. The effectiveness of the proposed method is evaluated in the Car Learning to Act (CARLA) simulation platform. Results demonstrate that the proposed MUACP efficiently performs cooperative formation in real time and outperforms other benchmark approaches in various scenarios under imperfect knowledge of the environment.
Via
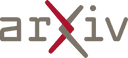
Jul 22, 2024
Abstract:Robots should personalize how they perform tasks to match the needs of individual human users. Today's robot achieve this personalization by asking for the human's feedback in the task space. For example, an autonomous car might show the human two different ways to decelerate at stoplights, and ask the human which of these motions they prefer. This current approach to personalization is indirect: based on the behaviors the human selects (e.g., decelerating slowly), the robot tries to infer their underlying preference (e.g., defensive driving). By contrast, our paper develops a learning and interface-based approach that enables humans to directly indicate their desired style. We do this by learning an abstract, low-dimensional, and continuous canonical space from human demonstration data. Each point in the canonical space corresponds to a different style (e.g., defensive or aggressive driving), and users can directly personalize the robot's behavior by simply clicking on a point. Given the human's selection, the robot then decodes this canonical style across each task in the dataset -- e.g., if the human selects a defensive style, the autonomous car personalizes its behavior to drive defensively when decelerating, passing other cars, or merging onto highways. We refer to our resulting approach as PECAN: Personalizing Robot Behaviors through a Learned Canonical Space. Our simulations and user studies suggest that humans prefer using PECAN to directly personalize robot behavior (particularly when those users become familiar with PECAN), and that users find the learned canonical space to be intuitive and consistent. See videos here: https://youtu.be/wRJpyr23PKI
* 22 pages, 7 figures
Via
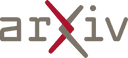
Jun 13, 2024
Abstract:This document presents the design of an autonomous car developed by the UruBots team for the 2024 FIRA Autonomous Cars Race Challenge. The project involves creating an RC-car sized electric vehicle capable of navigating race tracks with in an autonomous manner. It integrates mechanical and electronic systems alongside artificial intelligence based algorithms for the navigation and real-time decision-making. The core of our project include the utilization of an AI-based algorithm to learn information from a camera and act in the robot to perform the navigation. We show that by creating a dataset with more than five thousand samples and a five-layered CNN we managed to achieve promissing performance we our proposed hardware setup. Overall, this paper aims to demonstrate the autonomous capabilities of our car, highlighting its readiness for the 2024 FIRA challenge, helping to contribute to the field of autonomous vehicle research.
* Team Description Paper for the FIRA RoboWorld Cup 2024
Via
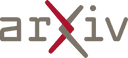