What is Sentiment Analysis? Sentiment analysis is the process of determining the sentiment of a piece of text, such as a tweet or a review.
Papers and Code
Mar 07, 2025
Abstract:Finance decision-making often relies on in-depth data analysis across various data sources, including financial tables, news articles, stock prices, etc. In this work, we introduce FinTMMBench, the first comprehensive benchmark for evaluating temporal-aware multi-modal Retrieval-Augmented Generation (RAG) systems in finance. Built from heterologous data of NASDAQ 100 companies, FinTMMBench offers three significant advantages. 1) Multi-modal Corpus: It encompasses a hybrid of financial tables, news articles, daily stock prices, and visual technical charts as the corpus. 2) Temporal-aware Questions: Each question requires the retrieval and interpretation of its relevant data over a specific time period, including daily, weekly, monthly, quarterly, and annual periods. 3) Diverse Financial Analysis Tasks: The questions involve 10 different tasks, including information extraction, trend analysis, sentiment analysis and event detection, etc. We further propose a novel TMMHybridRAG method, which first leverages LLMs to convert data from other modalities (e.g., tabular, visual and time-series data) into textual format and then incorporates temporal information in each node when constructing graphs and dense indexes. Its effectiveness has been validated in extensive experiments, but notable gaps remain, highlighting the challenges presented by our FinTMMBench.
* Under review
Via
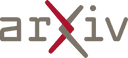
Mar 11, 2025
Abstract:Code-switching, or alternating between languages within a single conversation, presents challenges for multilingual language models on NLP tasks. This research investigates if pre-training Multilingual BERT (mBERT) on code-switched datasets improves the model's performance on critical NLP tasks such as part of speech tagging, sentiment analysis, named entity recognition, and language identification. We use a dataset of Spanglish tweets for pre-training and evaluate the pre-trained model against a baseline model. Our findings show that our pre-trained mBERT model outperforms or matches the baseline model in the given tasks, with the most significant improvements seen for parts of speech tagging. Additionally, our latent analysis uncovers more homogenous English and Spanish embeddings for language identification tasks, providing insights for future modeling work. This research highlights potential for adapting multilingual LMs for code-switched input data in order for advanced utility in globalized and multilingual contexts. Future work includes extending experiments to other language pairs, incorporating multiform data, and exploring methods for better understanding context-dependent code-switches.
Via
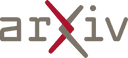
Feb 28, 2025
Abstract:Sentiment Analysis (SA) is instrumental in understanding peoples viewpoints facilitating social media monitoring recognizing products and brands and gauging customer satisfaction. Consequently SA has evolved into an active research domain within Natural Language Processing (NLP). Many approaches outlined in the literature devise intricate frameworks aimed at achieving high accuracy, focusing exclusively on either binary sentiment classification or fine-grained sentiment classification. In this paper our objective is to fine-tune the pre-trained BERT model with Bidirectional LSTM (BiLSTM) to enhance both binary and fine-grained SA specifically for movie reviews. Our approach involves conducting sentiment classification for each review followed by computing the overall sentiment polarity across all reviews. We present our findings on binary classification as well as fine-grained classification utilizing benchmark datasets. Additionally we implement and assess two accuracy improvement techniques Synthetic Minority Oversampling Technique (SMOTE) and NLP Augmenter (NLPAUG) to bolster the models generalization in fine-grained sentiment classification. Finally a heuristic algorithm is employed to calculate the overall polarity of predicted reviews from the BERT+BiLSTM output vector. Our approach performs comparably with state-of-the-art (SOTA) techniques in both classifications. For instance in binary classification we achieve 97.67% accuracy surpassing the leading SOTA model NB-weighted-BON+dv-cosine by 0.27% on the renowned IMDb dataset. Conversely for five-class classification on SST-5 while the top SOTA model RoBERTa+large+Self-explaining attains 55.5% accuracy our model achieves 59.48% accuracy surpassing the BERT-large baseline by 3.6%.
* 14 pages, 5 figures, published in International Journal On Advances
in Systems and Measurements, volume 16, numbers 3 and 4, 2023
Via
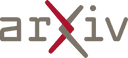
Feb 21, 2025
Abstract:As more and more internet users post images online to express their daily emotions, image sentiment analysis has attracted increasing attention. Recently, researchers generally tend to design different neural networks to extract visual features from images for sentiment analysis. Despite the significant progress, metadata, the data (e.g., text descriptions and keyword tags) for describing the image, has not been sufficiently explored in this task. In this paper, we propose a novel Metadata Enhanced Transformer for sentiment analysis (SentiFormer) to fuse multiple metadata and the corresponding image into a unified framework. Specifically, we first obtain multiple metadata of the image and unify the representations of diverse data. To adaptively learn the appropriate weights for each metadata, we then design an adaptive relevance learning module to highlight more effective information while suppressing weaker ones. Moreover, we further develop a cross-modal fusion module to fuse the adaptively learned representations and make the final prediction. Extensive experiments on three publicly available datasets demonstrate the superiority and rationality of our proposed method.
Via
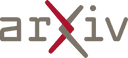
Mar 09, 2025
Abstract:This paper focuses on multimodal alignment within the realm of Artificial Intelligence, particularly in text and image modalities. The semantic gap between the textual and visual modality poses a discrepancy problem towards the effectiveness of multi-modalities fusion. Therefore, we introduce Text-Image Joint Embedding Predictive Architecture (TI-JEPA), an innovative pre-training strategy that leverages energy-based model (EBM) framework to capture complex cross-modal relationships. TI-JEPA combines the flexibility of EBM in self-supervised learning to facilitate the compatibility between textual and visual elements. Through extensive experiments across multiple benchmarks, we demonstrate that TI-JEPA achieves state-of-the-art performance on multimodal sentiment analysis task (and potentially on a wide range of multimodal-based tasks, such as Visual Question Answering), outperforming existing pre-training methodologies. Our findings highlight the potential of using energy-based framework in advancing multimodal fusion and suggest significant improvements for downstream applications.
Via
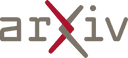
Mar 25, 2025
Abstract:Understanding how emotions are expressed across languages is vital for building culturally-aware and inclusive NLP systems. However, emotion expression in African languages is understudied, limiting the development of effective emotion detection tools in these languages. In this work, we present a cross-linguistic analysis of emotion expression in 15 African languages. We examine four key dimensions of emotion representation: text length, sentiment polarity, emotion co-occurrence, and intensity variations. Our findings reveal diverse language-specific patterns in emotional expression -- with Somali texts typically longer, while others like IsiZulu and Algerian Arabic show more concise emotional expression. We observe a higher prevalence of negative sentiment in several Nigerian languages compared to lower negativity in languages like IsiXhosa. Further, emotion co-occurrence analysis demonstrates strong cross-linguistic associations between specific emotion pairs (anger-disgust, sadness-fear), suggesting universal psychological connections. Intensity distributions show multimodal patterns with significant variations between language families; Bantu languages display similar yet distinct profiles, while Afroasiatic languages and Nigerian Pidgin demonstrate wider intensity ranges. These findings highlight the need for language-specific approaches to emotion detection while identifying opportunities for transfer learning across related languages.
Via
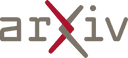
Feb 24, 2025
Abstract:A sentiment analysis system powered by machine learning was created in this study to improve real-time social network public opinion monitoring. For sophisticated sentiment identification, the suggested approach combines cutting-edge transformer-based architectures (DistilBERT, RoBERTa) with traditional machine learning models (Logistic Regression, SVM, Naive Bayes). The system achieved an accuracy of up to 80-85% using transformer models in real-world scenarios after being tested using both deep learning techniques and standard machine learning processes on annotated social media datasets. According to experimental results, deep learning models perform noticeably better than lexicon-based and conventional rule-based classifiers, lowering misclassification rates and enhancing the ability to recognize nuances like sarcasm. According to feature importance analysis, context tokens, sentiment-bearing keywords, and part-of-speech structure are essential for precise categorization. The findings confirm that AI-driven sentiment frameworks can provide a more adaptive and efficient approach to modern sentiment challenges. Despite the system's impressive performance, issues with computing overhead, data quality, and domain-specific terminology still exist. In order to monitor opinions on a broad scale, future research will investigate improving computing performance, extending coverage to various languages, and integrating real-time streaming APIs. The results demonstrate that governments, corporations, and social researchers looking for more in-depth understanding of public mood on digital platforms can find a reliable and adaptable answer in AI-powered sentiment analysis.
* 10 pages, 5 figures, 2 tables. Preprint submitted for community
feedback
Via
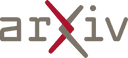
Mar 07, 2025
Abstract:In recent years, the application of behavioral testing in Natural Language Processing (NLP) model evaluation has experienced a remarkable and substantial growth. However, the existing methods continue to be restricted by the requirements for manual labor and the limited scope of capability assessment. To address these limitations, we introduce AutoTestForge, an automated and multidimensional testing framework for NLP models in this paper. Within AutoTestForge, through the utilization of Large Language Models (LLMs) to automatically generate test templates and instantiate them, manual involvement is significantly reduced. Additionally, a mechanism for the validation of test case labels based on differential testing is implemented which makes use of a multi-model voting system to guarantee the quality of test cases. The framework also extends the test suite across three dimensions, taxonomy, fairness, and robustness, offering a comprehensive evaluation of the capabilities of NLP models. This expansion enables a more in-depth and thorough assessment of the models, providing valuable insights into their strengths and weaknesses. A comprehensive evaluation across sentiment analysis (SA) and semantic textual similarity (STS) tasks demonstrates that AutoTestForge consistently outperforms existing datasets and testing tools, achieving higher error detection rates (an average of $30.89\%$ for SA and $34.58\%$ for STS). Moreover, different generation strategies exhibit stable effectiveness, with error detection rates ranging from $29.03\% - 36.82\%$.
* 15 pages, 4 figures, Under review
Via
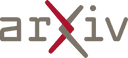
Mar 04, 2025
Abstract:This paper investigates the challenges of affect control in large language models (LLMs), focusing on their ability to express appropriate emotional states during extended dialogues. We evaluated state-of-the-art open-weight LLMs to assess their affective expressive range in terms of arousal and valence. Our study employs a novel methodology combining LLM-based sentiment analysis with multiturn dialogue simulations between LLMs. We quantify the models' capacity to express a wide spectrum of emotions and how they fluctuate during interactions. Our findings reveal significant variations among LLMs in their ability to maintain consistent affect, with some models demonstrating more stable emotional trajectories than others. Furthermore, we identify key challenges in affect control, including difficulties in producing and maintaining extreme emotional states and limitations in adapting affect to changing conversational contexts. These findings have important implications for the development of more emotionally intelligent AI systems and highlight the need for improved affect modelling in LLMs.
Via
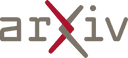
Feb 17, 2025
Abstract:Aspect-based sentiment analysis (ABSA) is a crucial task in information extraction and sentiment analysis, aiming to identify aspects with associated sentiment elements in text. However, existing ABSA datasets are predominantly English-centric, limiting the scope for multilingual evaluation and research. To bridge this gap, we present M-ABSA, a comprehensive dataset spanning 7 domains and 21 languages, making it the most extensive multilingual parallel dataset for ABSA to date. Our primary focus is on triplet extraction, which involves identifying aspect terms, aspect categories, and sentiment polarities. The dataset is constructed through an automatic translation process with human review to ensure quality. We perform extensive experiments using various baselines to assess performance and compatibility on M-ABSA. Our empirical findings highlight that the dataset enables diverse evaluation tasks, such as multilingual and multi-domain transfer learning, and large language model evaluation, underscoring its inclusivity and its potential to drive advancements in multilingual ABSA research.
Via
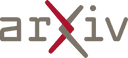