What is Recommendation? Recommendation is the task of providing personalized suggestions to users based on their preferences and behavior.
Papers and Code
Jun 17, 2025
Abstract:Following recent advancements in large language models (LLMs), LLM-based chatbots have transformed customer support by automating interactions and providing consistent, scalable service. While LLM-based conversational recommender systems (CRSs) have attracted attention for their ability to enhance the quality of recommendations, limited research has addressed the implicit integration of recommendations within customer support interactions. In this work, we introduce ImpReSS, an implicit recommender system designed for customer support conversations. ImpReSS operates alongside existing support chatbots, where users report issues and chatbots provide solutions. Based on a customer support conversation, ImpReSS identifies opportunities to recommend relevant solution product categories (SPCs) that help resolve the issue or prevent its recurrence -- thereby also supporting business growth. Unlike traditional CRSs, ImpReSS functions entirely implicitly and does not rely on any assumption of a user's purchasing intent. Our empirical evaluation of ImpReSS's ability to recommend relevant SPCs that can help address issues raised in support conversations shows promising results, including an MRR@1 (and recall@3) of 0.72 (0.89) for general problem solving, 0.82 (0.83) for information security support, and 0.85 (0.67) for cybersecurity troubleshooting. To support future research, our data and code will be shared upon request.
Via
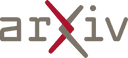
Jun 17, 2025
Abstract:Achieving high synchronization in the synthesis of realistic, speech-driven talking head videos presents a significant challenge. A lifelike talking head requires synchronized coordination of subject identity, lip movements, facial expressions, and head poses. The absence of these synchronizations is a fundamental flaw, leading to unrealistic results. To address the critical issue of synchronization, identified as the ''devil'' in creating realistic talking heads, we introduce SyncTalk++, which features a Dynamic Portrait Renderer with Gaussian Splatting to ensure consistent subject identity preservation and a Face-Sync Controller that aligns lip movements with speech while innovatively using a 3D facial blendshape model to reconstruct accurate facial expressions. To ensure natural head movements, we propose a Head-Sync Stabilizer, which optimizes head poses for greater stability. Additionally, SyncTalk++ enhances robustness to out-of-distribution (OOD) audio by incorporating an Expression Generator and a Torso Restorer, which generate speech-matched facial expressions and seamless torso regions. Our approach maintains consistency and continuity in visual details across frames and significantly improves rendering speed and quality, achieving up to 101 frames per second. Extensive experiments and user studies demonstrate that SyncTalk++ outperforms state-of-the-art methods in synchronization and realism. We recommend watching the supplementary video: https://ziqiaopeng.github.io/synctalk++.
Via
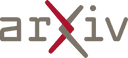
Jun 16, 2025
Abstract:Data scarcity in African agriculture hampers machine learning (ML) model performance, limiting innovations in precision agriculture. The Synthetic Agriculture Data for Africa (SAGDA) library, a Python-based open-source toolkit, addresses this gap by generating, augmenting, and validating synthetic agricultural datasets. We present SAGDA's design and development practices, highlighting its core functions: generate, model, augment, validate, visualize, optimize, and simulate, as well as their roles in applications of ML for agriculture. Two use cases are detailed: yield prediction enhanced via data augmentation, and multi-objective NPK (nitrogen, phosphorus, potassium) fertilizer recommendation. We conclude with future plans for expanding SAGDA's capabilities, underscoring the vital role of open-source, data-driven practices for African agriculture.
* Proceedings of the ICML 2025 Workshop on Championing Open-source
Development in Machine Learning (CODEML '25)
Via
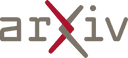
Jun 16, 2025
Abstract:Developing a single foundation model with the capability to excel across diverse tasks has been a long-standing objective in the field of artificial intelligence. As the wave of general-purpose foundation models sweeps across various domains, their influence has significantly extended to the field of recommendation systems. While recent efforts have explored recommendation foundation models for various generative tasks, they often overlook crucial embedding tasks and struggle with the complexities of multi-task learning, including knowledge sharing & conflict resolution, and convergence speed inconsistencies. To address these limitations, we introduce RecFound, a generative representational learning framework for recommendation foundation models. We construct the first comprehensive dataset for recommendation foundation models covering both generative and embedding tasks across diverse scenarios. Based on this dataset, we propose a novel multi-task training scheme featuring a Task-wise Mixture of Low-rank Experts (TMoLE) to handle knowledge sharing & conflict, a Step-wise Convergence-oriented Sample Scheduler (S2Sched) to address inconsistent convergence, and a Model Merge module to balance the performance across tasks. Experiments demonstrate that RecFound achieves state-of-the-art performance across various recommendation tasks, outperforming existing baselines.
Via
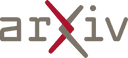
Jun 16, 2025
Abstract:Traditionally, Recommender Systems (RS) have primarily measured performance based on the accuracy and relevance of their recommendations. However, this algorithmic-centric approach overlooks how different types of recommendations impact user engagement and shape the overall quality of experience. In this paper, we shift the focus to the user and address for the first time the challenge of decoding the neural and behavioural variability across distinct recommendation categories, considering more than just relevance. Specifically, we conducted a controlled study using a comprehensive e-commerce dataset containing various recommendation types, and collected Electroencephalography and behavioural data. We analysed both neural and behavioural responses to recommendations that were categorised as Exact, Substitute, Complement, or Irrelevant products within search query results. Our findings offer novel insights into user preferences and decision-making processes, revealing meaningful relationships between behavioural and neural patterns for each category, but also indicate inter-subject variability.
* 11 pages, 7 figures, 5 tables
Via
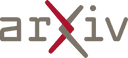
Jun 16, 2025
Abstract:The ongoing evolution of language models has led to the development of large-scale architectures that demonstrate exceptional performance across a wide range of tasks. However, these models come with significant computational and energy demands, as well as potential privacy implications. In this context, Small Reasoning Language Models (SRLMs) with approximately 0.5 billion parameters present a compelling alternative due to their remarkable computational efficiency and cost effectiveness, particularly in resource-constrained environments. Despite these advantages, the limited capacity of 0.5 billion parameter models poses challenges in handling complex tasks such as mathematical reasoning and code generation. This research investigates various training strategies, including supervised fine-tuning (SFT), knowledge distillation (KD), and reinforcement learning (RL), as well as their hybrid implementations, to enhance the performance of 0.5B SRLMs. We analyze effective methodologies to bridge the performance gap between SRLMS and larger models and present insights into optimal training pipelines tailored for these smaller architectures. Through extensive experimental validation and analysis, our work aims to provide actionable recommendations for maximizing the reasoning capabilities of 0.5B models.
Via
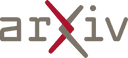
Jun 16, 2025
Abstract:In Sequential Recommendation Systems (SRSs), Transformer models show remarkable performance but face computation cost challenges when modeling long-term user behavior sequences due to the quadratic complexity of the dot-product attention mechanism. By approximating the dot-product attention, linear attention provides an efficient option with linear complexity. However, existing linear attention methods face two limitations: 1) they often use learnable position encodings, which incur extra computational costs in long-term sequence scenarios, and 2) they may not consider the user's fine-grained local preferences and confuse these with the actual change of long-term interests. To remedy these drawbacks, we propose a long-term sequential Recommendation model with Gated Rotary Enhanced Linear Attention (RecGRELA). Specifically, we first propose a Rotary-Enhanced Linear Attention (RELA) module to model long-range dependency within the user's historical information using rotary position encodings. We then introduce a local short operation to incorporate local preferences and demonstrate the theoretical insight. We further introduce a SiLU-based Gated mechanism for RELA (GRELA) to help the model determine whether a user's behavior indicates local interest or a genuine shift in long-term preferences. Experimental results on four public datasets demonstrate that our RecGRELA achieves state-of-the-art performance compared to existing SRSs while maintaining low memory overhead.
* 24 pages,9 figures
Via
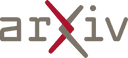
Jun 16, 2025
Abstract:Text-attributed graphs (TAGs) integrate textual data with graph structures, providing valuable insights in applications such as social network analysis and recommendation systems. Graph Neural Networks (GNNs) effectively capture both topological structure and textual information in TAGs but are vulnerable to adversarial attacks. Existing graph injection attack (GIA) methods assume that attackers can directly manipulate the embedding layer, producing non-explainable node embeddings. Furthermore, the effectiveness of these attacks often relies on surrogate models with high training costs. Thus, this paper introduces ATAG-LLM, a novel black-box GIA framework tailored for TAGs. Our approach leverages large language models (LLMs) to generate interpretable text-level node attributes directly, ensuring attacks remain feasible in real-world scenarios. We design strategies for LLM prompting that balance exploration and reliability to guide text generation, and propose a similarity assessment method to evaluate attack text effectiveness in disrupting graph homophily. This method efficiently perturbs the target node with minimal training costs in a strict black-box setting, ensuring a text-level graph injection attack for TAGs. Experiments on real-world TAG datasets validate the superior performance of ATAG-LLM compared to state-of-the-art embedding-level and text-level attack methods.
Via
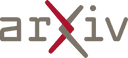
Jun 16, 2025
Abstract:Sequential recommender systems aim to model users' evolving preferences by capturing patterns in their historical interactions. Recent advances in this area have leveraged deep neural networks and attention mechanisms to effectively represent sequential behaviors and time-sensitive interests. In this work, we propose C-TLSAN (Content-Enhanced Time-Aware Long- and Short-Term Attention Network), an extension of the TLSAN architecture that jointly models long- and short-term user preferences while incorporating semantic content associated with items, such as product descriptions. C-TLSAN enriches the recommendation pipeline by embedding textual content linked to users' historical interactions directly into both long-term and short-term attention layers. This allows the model to learn from both behavioral patterns and rich item content, enhancing user and item representations across temporal dimensions. By fusing sequential signals with textual semantics, our approach improves the expressiveness and personalization capacity of recommendation systems. We conduct extensive experiments on large-scale Amazon datasets, benchmarking C-TLSAN against state-of-the-art baselines, including recent sequential recommenders based on Large Language Models (LLMs), which represent interaction history and predictions in text form. Empirical results demonstrate that C-TLSAN consistently outperforms strong baselines in next-item prediction tasks. Notably, it improves AUC by 1.66%, Recall@10 by 93.99%, and Precision@10 by 94.80% on average over the best-performing baseline (TLSAN) across 10 Amazon product categories. These results highlight the value of integrating content-aware enhancements into temporal modeling frameworks for sequential recommendation. Our code is available at https://github.com/booml247/cTLSAN.
Via
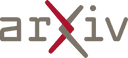
Jun 16, 2025
Abstract:Large Language Models (LLMs) have shown strong potential for recommendation by framing item prediction as a token-by-token language generation task. However, existing methods treat all item tokens equally, simply pursuing likelihood maximization during both optimization and decoding. This overlooks crucial token-level differences in decisiveness-many tokens contribute little to item discrimination yet can dominate optimization or decoding. To quantify token decisiveness, we propose a novel perspective that models item generation as a decision process, measuring token decisiveness by the Information Gain (IG) each token provides in reducing uncertainty about the generated item. Our empirical analysis reveals that most tokens have low IG but often correspond to high logits, disproportionately influencing training loss and decoding, which may impair model performance. Building on these insights, we introduce an Information Gain-based Decisiveness-aware Token handling (IGD) strategy that integrates token decisiveness into both tuning and decoding. Specifically, IGD downweights low-IG tokens during tuning and rebalances decoding to emphasize tokens with high IG. In this way, IGD moves beyond pure likelihood maximization, effectively prioritizing high-decisiveness tokens. Extensive experiments on four benchmark datasets with two LLM backbones demonstrate that IGD consistently improves recommendation accuracy, achieving significant gains on widely used ranking metrics compared to strong baselines.
Via
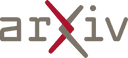