May 26, 2025
Abstract:Recent advancements in radiance field rendering, exemplified by Neural Radiance Fields (NeRF) and 3D Gaussian Splatting (3DGS), have significantly progressed 3D modeling and reconstruction. The use of multiple 360-degree omnidirectional images for these tasks is increasingly favored due to advantages in data acquisition and comprehensive scene capture. However, the inherent geometric distortions in common omnidirectional representations, such as equirectangular projection (particularly severe in polar regions and varying with latitude), pose substantial challenges to achieving high-fidelity 3D reconstructions. Current datasets, while valuable, often lack the specific focus, scene composition, and ground truth granularity required to systematically benchmark and drive progress in overcoming these omnidirectional-specific challenges. To address this critical gap, we introduce Omnidirectional Blender 3D (OB3D), a new synthetic dataset curated for advancing 3D reconstruction from multiple omnidirectional images. OB3D features diverse and complex 3D scenes generated from Blender 3D projects, with a deliberate emphasis on challenging scenarios. The dataset provides comprehensive ground truth, including omnidirectional RGB images, precise omnidirectional camera parameters, and pixel-aligned equirectangular maps for depth and normals, alongside evaluation metrics. By offering a controlled yet challenging environment, OB3Daims to facilitate the rigorous evaluation of existing methods and prompt the development of new techniques to enhance the accuracy and reliability of 3D reconstruction from omnidirectional images.
Via
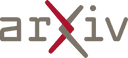