What is Image Matting? Image matting is the process of extracting the foreground object from an image and creating a transparent background.
Papers and Code
May 20, 2025
Abstract:A key trend in Large Reasoning Models (e.g., OpenAI's o3) is the native agentic ability to use external tools such as web browsers for searching and writing/executing code for image manipulation to think with images. In the open-source research community, while significant progress has been made in language-only agentic abilities such as function calling and tool integration, the development of multi-modal agentic capabilities that involve truly thinking with images, and their corresponding benchmarks, are still less explored. This work highlights the effectiveness of Visual Agentic Reinforcement Fine-Tuning (Visual-ARFT) for enabling flexible and adaptive reasoning abilities for Large Vision-Language Models (LVLMs). With Visual-ARFT, open-source LVLMs gain the ability to browse websites for real-time information updates and write code to manipulate and analyze input images through cropping, rotation, and other image processing techniques. We also present a Multi-modal Agentic Tool Bench (MAT) with two settings (MAT-Search and MAT-Coding) designed to evaluate LVLMs' agentic search and coding abilities. Our experimental results demonstrate that Visual-ARFT outperforms its baseline by +18.6% F1 / +13.0% EM on MAT-Coding and +10.3% F1 / +8.7% EM on MAT-Search, ultimately surpassing GPT-4o. Visual-ARFT also achieves +29.3 F1% / +25.9% EM gains on existing multi-hop QA benchmarks such as 2Wiki and HotpotQA, demonstrating strong generalization capabilities. Our findings suggest that Visual-ARFT offers a promising path toward building robust and generalizable multimodal agents.
Via
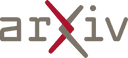
Apr 20, 2025
Abstract:Human instance matting aims to estimate an alpha matte for each human instance in an image, which is challenging as it easily fails in complex cases requiring disentangling mingled pixels belonging to multiple instances along hairy and thin boundary structures. In this work, we address this by introducing MP-Mat, a novel 3D-and-instance-aware matting framework with multiplane representation, where the multiplane concept is designed from two different perspectives: scene geometry level and instance level. Specifically, we first build feature-level multiplane representations to split the scene into multiple planes based on depth differences. This approach makes the scene representation 3D-aware, and can serve as an effective clue for splitting instances in different 3D positions, thereby improving interpretability and boundary handling ability especially in occlusion areas. Then, we introduce another multiplane representation that splits the scene in an instance-level perspective, and represents each instance with both matte and color. We also treat background as a special instance, which is often overlooked by existing methods. Such an instance-level representation facilitates both foreground and background content awareness, and is useful for other down-stream tasks like image editing. Once built, the representation can be reused to realize controllable instance-level image editing with high efficiency. Extensive experiments validate the clear advantage of MP-Mat in matting task. We also demonstrate its superiority in image editing tasks, an area under-explored by existing matting-focused methods, where our approach under zero-shot inference even outperforms trained specialized image editing techniques by large margins. Code is open-sourced at https://github.com/JiaoSiyi/MPMat.git}.
* Accepted by ICLR 2025
Via
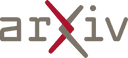
Mar 05, 2025
Abstract:In this paper, we explore a novel image matting task aimed at achieving efficient inference under various computational cost constraints, specifically FLOP limitations, using a single matting network. Existing matting methods which have not explored scalable architectures or path-learning strategies, fail to tackle this challenge. To overcome these limitations, we introduce Path-Adaptive Matting (PAM), a framework that dynamically adjusts network paths based on image contexts and computational cost constraints. We formulate the training of the computational cost-constrained matting network as a bilevel optimization problem, jointly optimizing the matting network and the path estimator. Building on this formalization, we design a path-adaptive matting architecture by incorporating path selection layers and learnable connect layers to estimate optimal paths and perform efficient inference within a unified network. Furthermore, we propose a performance-aware path-learning strategy to generate path labels online by evaluating a few paths sampled from the prior distribution of optimal paths and network estimations, enabling robust and efficient online path learning. Experiments on five image matting datasets demonstrate that the proposed PAM framework achieves competitive performance across a range of computational cost constraints.
* Accepted to AAAI 2025
Via
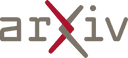
Feb 24, 2025
Abstract:Real-world image matting is essential for applications in content creation and augmented reality. However, it remains challenging due to the complex nature of scenes and the scarcity of high-quality datasets. To address these limitations, we introduce Mask2Alpha, an iterative refinement framework designed to enhance semantic comprehension, instance awareness, and fine-detail recovery in image matting. Our framework leverages self-supervised Vision Transformer features as semantic priors, strengthening contextual understanding in complex scenarios. To further improve instance differentiation, we implement a mask-guided feature selection module, enabling precise targeting of objects in multi-instance settings. Additionally, a sparse convolution-based optimization scheme allows Mask2Alpha to recover high-resolution details through progressive refinement,from low-resolution semantic passes to high-resolution sparse reconstructions. Benchmarking across various real-world datasets, Mask2Alpha consistently achieves state-of-the-art results, showcasing its effectiveness in accurate and efficient image matting.
* 9pages
Via
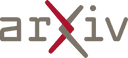
Jan 27, 2025
Abstract:Learning effective deep portrait matting models requires training data of both high quality and large quantity. Neither quality nor quantity can be easily met for portrait matting, however. Since the most accurate ground-truth portrait mattes are acquired in front of the green screen, it is almost impossible to harvest a large-scale portrait matting dataset in reality. This work shows that one can leverage text prompts and the recent Layer Diffusion model to generate high-quality portrait foregrounds and extract latent portrait mattes. However, the portrait mattes cannot be readily in use due to significant generation artifacts. Inspired by the connectivity priors observed in portrait images, that is, the border of portrait foregrounds always appears connected, a connectivity-aware approach is introduced to refine portrait mattes. Building on this, a large-scale portrait matting dataset is created, termed LD-Portrait-20K, with $20,051$ portrait foregrounds and high-quality alpha mattes. Extensive experiments demonstrated the value of the LD-Portrait-20K dataset, with models trained on it significantly outperforming those trained on other datasets. In addition, comparisons with the chroma keying algorithm and an ablation study on dataset capacity further confirmed the effectiveness of the proposed matte creation approach. Further, the dataset also contributes to state-of-the-art video portrait matting, implemented by simple video segmentation and a trimap-based image matting model trained on this dataset.
Via
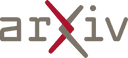
Jan 08, 2025
Abstract:Current approaches to dichotomous image segmentation (DIS) treat image matting and object segmentation as fundamentally different tasks. As improvements in image segmentation become increasingly challenging to achieve, combining image matting and grayscale segmentation techniques offers promising new directions for architectural innovation. Inspired by the possibility of aligning these two model tasks, we propose a new architectural approach for DIS called Confidence-Guided Matting (CGM). We created the first CGM model called Background Erase Network (BEN). BEN is comprised of two components: BEN Base for initial segmentation and BEN Refiner for confidence refinement. Our approach achieves substantial improvements over current state-of-the-art methods on the DIS5K validation dataset, demonstrating that matting-based refinement can significantly enhance segmentation quality. This work opens new possibilities for cross-pollination between matting and segmentation techniques in computer vision.
* 13 pages, 2 figures, 2 tables, and 2 algorithms
Via
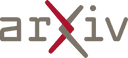
Dec 30, 2024
Abstract:Transparent objects are ubiquitous in daily life, making their perception and robotics manipulation important. However, they present a major challenge due to their distinct refractive and reflective properties when it comes to accurately estimating the 6D pose. To solve this, we present ReFlow6D, a novel method for transparent object 6D pose estimation that harnesses the refractive-intermediate representation. Unlike conventional approaches, our method leverages a feature space impervious to changes in RGB image space and independent of depth information. Drawing inspiration from image matting, we model the deformation of the light path through transparent objects, yielding a unique object-specific intermediate representation guided by light refraction that is independent of the environment in which objects are observed. By integrating these intermediate features into the pose estimation network, we show that ReFlow6D achieves precise 6D pose estimation of transparent objects, using only RGB images as input. Our method further introduces a novel transparent object compositing loss, fostering the generation of superior refractive-intermediate features. Empirical evaluations show that our approach significantly outperforms state-of-the-art methods on TOD and Trans32K-6D datasets. Robot grasping experiments further demonstrate that ReFlow6D's pose estimation accuracy effectively translates to real-world robotics task. The source code is available at: https://github.com/StoicGilgamesh/ReFlow6D and https://github.com/StoicGilgamesh/matting_rendering.
* IEEE Robotics and Automation Letters, vol. 9, no. 11, pp.
9438-9445, Nov. 2024
Via
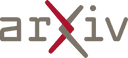
Dec 17, 2024
Abstract:Transformer-based models have recently achieved outstanding performance in image matting. However, their application to high-resolution images remains challenging due to the quadratic complexity of global self-attention. To address this issue, we propose MEMatte, a \textbf{m}emory-\textbf{e}fficient \textbf{m}atting framework for processing high-resolution images. MEMatte incorporates a router before each global attention block, directing informative tokens to the global attention while routing other tokens to a Lightweight Token Refinement Module (LTRM). Specifically, the router employs a local-global strategy to predict the routing probability of each token, and the LTRM utilizes efficient modules to simulate global attention. Additionally, we introduce a Batch-constrained Adaptive Token Routing (BATR) mechanism, which allows each router to dynamically route tokens based on image content and the stages of attention block in the network. Furthermore, we construct an ultra high-resolution image matting dataset, UHR-395, comprising 35,500 training images and 1,000 test images, with an average resolution of $4872\times6017$. This dataset is created by compositing 395 different alpha mattes across 11 categories onto various backgrounds, all with high-quality manual annotation. Extensive experiments demonstrate that MEMatte outperforms existing methods on both high-resolution and real-world datasets, significantly reducing memory usage by approximately 88% and latency by 50% on the Composition-1K benchmark. Our code is available at https://github.com/linyiheng123/MEMatte.
Via
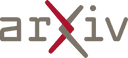
Nov 15, 2024
Abstract:Vision Transformers (ViTs) and Convolutional Neural Networks (CNNs) face inherent challenges in image matting, particularly in preserving fine structural details. ViTs, with their global receptive field enabled by the self-attention mechanism, often lose local details such as hair strands. Conversely, CNNs, constrained by their local receptive field, rely on deeper layers to approximate global context but struggle to retain fine structures at greater depths. To overcome these limitations, we propose a novel Morpho-Aware Global Attention (MAGA) mechanism, designed to effectively capture the morphology of fine structures. MAGA employs Tetris-like convolutional patterns to align the local shapes of fine structures, ensuring optimal local correspondence while maintaining sensitivity to morphological details. The extracted local morphology information is used as query embeddings, which are projected onto global key embeddings to emphasize local details in a broader context. Subsequently, by projecting onto value embeddings, MAGA seamlessly integrates these emphasized morphological details into a unified global structure. This approach enables MAGA to simultaneously focus on local morphology and unify these details into a coherent whole, effectively preserving fine structures. Extensive experiments show that our MAGA-based ViT achieves significant performance gains, outperforming state-of-the-art methods across two benchmarks with average improvements of 4.3% in SAD and 39.5% in MSE.
Via
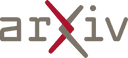
Dec 28, 2024
Abstract:Multimodal large language models (MLLMs) hold significant potential in the medical field, but their capabilities are often limited by insufficient data in certain medical domains, highlighting the need for understanding what kinds of images can be used by MLLMs for generalization. Current research suggests that multi-task training outperforms single-task as different tasks can benefit each other, but they often overlook the internal relationships within these tasks, providing limited guidance on selecting datasets to enhance specific tasks. To analyze this phenomenon, we attempted to employ compositional generalization (CG)-the ability of models to understand novel combinations by recombining learned elements-as a guiding framework. Since medical images can be precisely defined by Modality, Anatomical area, and Task, naturally providing an environment for exploring CG. Therefore, we assembled 106 medical datasets to create Med-MAT for comprehensive experiments. The experiments confirmed that MLLMs can use CG to understand unseen medical images and identified CG as one of the main drivers of the generalization observed in multi-task training. Additionally, further studies demonstrated that CG effectively supports datasets with limited data and delivers consistent performance across different backbones, highlighting its versatility and broad applicability. Med-MAT is publicly available at https://github.com/FreedomIntelligence/Med-MAT.
Via
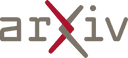