Jun 14, 2025
Abstract:A new class of audio deepfakes-codecfakes (CFs)-has recently caught attention, synthesized by Audio Language Models that leverage neural audio codecs (NACs) in the backend. In response, the community has introduced dedicated benchmarks and tailored detection strategies. As the field advances, efforts have moved beyond binary detection toward source attribution, including open-set attribution, which aims to identify the NAC responsible for generation and flag novel, unseen ones during inference. This shift toward source attribution improves forensic interpretability and accountability. However, open-set attribution remains fundamentally limited: while it can detect that a NAC is unfamiliar, it cannot characterize or identify individual unseen codecs. It treats such inputs as generic ``unknowns'', lacking insight into their internal configuration. This leads to major shortcomings: limited generalization to new NACs and inability to resolve fine-grained variations within NAC families. To address these gaps, we propose Neural Audio Codec Source Parsing (NACSP) - a paradigm shift that reframes source attribution for CFs as structured regression over generative NAC parameters such as quantizers, bandwidth, and sampling rate. We formulate NACSP as a multi-task regression task for predicting these NAC parameters and establish the first comprehensive benchmark using various state-of-the-art speech pre-trained models (PTMs). To this end, we propose HYDRA, a novel framework that leverages hyperbolic geometry to disentangle complex latent properties from PTM representations. By employing task-specific attention over multiple curvature-aware hyperbolic subspaces, HYDRA enables superior multi-task generalization. Our extensive experiments show HYDRA achieves top results on benchmark CFs datasets compared to baselines operating in Euclidean space.
Via
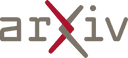