What is Object Detection? Object detection is a computer vision task in which the goal is to detect and locate objects of interest in an image or video. The task involves identifying the position and boundaries of objects in an image, and classifying the objects into different categories. It forms a crucial part of vision recognition, alongside image classification and retrieval.
Papers and Code
Jun 09, 2025
Abstract:Collaborative perception plays a crucial role in enhancing environmental understanding by expanding the perceptual range and improving robustness against sensor failures, which primarily involves collaborative 3D detection and tracking tasks. The former focuses on object recognition in individual frames, while the latter captures continuous instance tracklets over time. However, existing works in both areas predominantly focus on the vehicle superclass, lacking effective solutions for both multi-class collaborative detection and tracking. This limitation hinders their applicability in real-world scenarios, which involve diverse object classes with varying appearances and motion patterns. To overcome these limitations, we propose a multi-class collaborative detection and tracking framework tailored for diverse road users. We first present a detector with a global spatial attention fusion (GSAF) module, enhancing multi-scale feature learning for objects of varying sizes. Next, we introduce a tracklet RE-IDentification (REID) module that leverages visual semantics with a vision foundation model to effectively reduce ID SWitch (IDSW) errors, in cases of erroneous mismatches involving small objects like pedestrians. We further design a velocity-based adaptive tracklet management (VATM) module that adjusts the tracking interval dynamically based on object motion. Extensive experiments on the V2X-Real and OPV2V datasets show that our approach significantly outperforms existing state-of-the-art methods in both detection and tracking accuracy.
Via
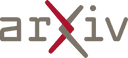
Jun 09, 2025
Abstract:Automated pollen recognition is vital to paleoclimatology, biodiversity monitoring, and public health, yet conventional methods are hampered by inefficiency and subjectivity. Existing deep learning models often struggle to achieve the requisite localization accuracy for microscopic targets like pollen, which are characterized by their minute size, indistinct edges, and complex backgrounds. To overcome this limitation, we introduce HieraEdgeNet, a multi-scale edge-enhancement framework. The framework's core innovation is the introduction of three synergistic modules: the Hierarchical Edge Module (HEM), which explicitly extracts a multi-scale pyramid of edge features that corresponds to the semantic hierarchy at early network stages; the Synergistic Edge Fusion (SEF) module, for deeply fusing these edge priors with semantic information at each respective scale; and the Cross Stage Partial Omni-Kernel Module (CSPOKM), which maximally refines the most detail-rich feature layers using an Omni-Kernel operator - comprising anisotropic large-kernel convolutions and mixed-domain attention - all within a computationally efficient Cross-Stage Partial (CSP) framework. On a large-scale dataset comprising 120 pollen classes, HieraEdgeNet achieves a mean Average Precision (mAP@.5) of 0.9501, significantly outperforming state-of-the-art baseline models such as YOLOv12n and RT-DETR. Furthermore, qualitative analysis confirms that our approach generates feature representations that are more precisely focused on object boundaries. By systematically integrating edge information, HieraEdgeNet provides a robust and powerful solution for high-precision, high-efficiency automated detection of microscopic objects.
Via
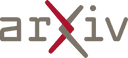
Jun 05, 2025
Abstract:Recently, Large Language Models (LLMs) and Vision Large Language Models (VLLMs) have demonstrated impressive performance as agents across various tasks while data scarcity and label noise remain significant challenges in computer vision tasks, such as object detection and instance segmentation. A common solution for resolving these issues is to generate synthetic data. However, current synthetic data generation methods struggle with issues, such as multiple objects per mask, inaccurate segmentation, and incorrect category labels, limiting their effectiveness. To address these issues, we introduce Gen-n-Val, a novel agentic data generation framework that leverages Layer Diffusion (LD), LLMs, and VLLMs to produce high-quality, single-object masks and diverse backgrounds. Gen-n-Val consists of two agents: (1) The LD prompt agent, an LLM, optimizes prompts for LD to generate high-quality foreground instance images and segmentation masks. These optimized prompts ensure the generation of single-object synthetic data with precise instance masks and clean backgrounds. (2) The data validation agent, a VLLM, which filters out low-quality synthetic instance images. The system prompts for both agents are refined through TextGrad. Additionally, we use image harmonization to combine multiple instances within scenes. Compared to state-of-the-art synthetic data approaches like MosaicFusion, our approach reduces invalid synthetic data from 50% to 7% and improves performance by 1% mAP on rare classes in COCO instance segmentation with YOLOv9c and YOLO11m. Furthermore, Gen-n-Val shows significant improvements (7. 1% mAP) over YOLO-Worldv2-M in open-vocabulary object detection benchmarks with YOLO11m. Moreover, Gen-n-Val improves the performance of YOLOv9 and YOLO11 families in instance segmentation and object detection.
Via
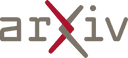
Jun 04, 2025
Abstract:Existing LiDAR 3D object detection methods predominantely rely on sparse convolutions and/or transformers, which can be challenging to run on resource-constrained edge devices, due to irregular memory access patterns and high computational costs. In this paper, we propose FALO, a hardware-friendly approach to LiDAR 3D detection, which offers both state-of-the-art (SOTA) detection accuracy and fast inference speed. More specifically, given the 3D point cloud and after voxelization, FALO first arranges sparse 3D voxels into a 1D sequence based on their coordinates and proximity. The sequence is then processed by our proposed ConvDotMix blocks, consisting of large-kernel convolutions, Hadamard products, and linear layers. ConvDotMix provides sufficient mixing capability in both spatial and embedding dimensions, and introduces higher-order nonlinear interaction among spatial features. Furthermore, when going through the ConvDotMix layers, we introduce implicit grouping, which balances the tensor dimensions for more efficient inference and takes into account the growing receptive field. All these operations are friendly to run on resource-constrained platforms and proposed FALO can readily deploy on compact, embedded devices. Our extensive evaluation on LiDAR 3D detection benchmarks such as nuScenes and Waymo shows that FALO achieves competitive performance. Meanwhile, FALO is 1.6~9.8x faster than the latest SOTA on mobile Graphics Processing Unit (GPU) and mobile Neural Processing Unit (NPU).
Via
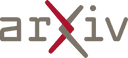
Jun 12, 2025
Abstract:Quality management in semiconductor manufacturing often relies on template matching with known golden standards. For Indium-Phosphide (InP) multi-project wafer manufacturing, low production scale and high design variability lead to such golden standards being typically unavailable. Defect detection, in turn, is manual and labor-intensive. This work addresses this challenge by proposing a methodology to generate a synthetic golden standard using Deep Neural Networks, trained to simulate photo-realistic InP wafer images from CAD data. We evaluate various training objectives and assess the quality of the simulated images on both synthetic data and InP wafer photographs. Our deep-learning-based method outperforms a baseline decision-tree-based approach, enabling the use of a 'simulated golden die' from CAD plans in any user-defined region of a wafer for more efficient defect detection. We apply our method to a template matching procedure, to demonstrate its practical utility in surface defect detection.
Via
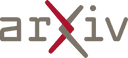
Jun 13, 2025
Abstract:Background: Coronary artery disease (CAD) remains one of the leading causes of mortality worldwide. Precise segmentation of coronary arteries from invasive coronary angiography (ICA) is critical for effective clinical decision-making. Objective: This study aims to propose a novel deep learning model based on frequency-domain analysis to enhance the accuracy of coronary artery segmentation and stenosis detection in ICA, thereby offering robust support for the stenosis detection and treatment of CAD. Methods: We propose the Frequency-Domain Attention-Guided Diffusion Network (FAD-Net), which integrates a frequency-domain-based attention mechanism and a cascading diffusion strategy to fully exploit frequency-domain information for improved segmentation accuracy. Specifically, FAD-Net employs a Multi-Level Self-Attention (MLSA) mechanism in the frequency domain, computing the similarity between queries and keys across high- and low-frequency components in ICAs. Furthermore, a Low-Frequency Diffusion Module (LFDM) is incorporated to decompose ICAs into low- and high-frequency components via multi-level wavelet transformation. Subsequently, it refines fine-grained arterial branches and edges by reintegrating high-frequency details via inverse fusion, enabling continuous enhancement of anatomical precision. Results and Conclusions: Extensive experiments demonstrate that FAD-Net achieves a mean Dice coefficient of 0.8717 in coronary artery segmentation, outperforming existing state-of-the-art methods. In addition, it attains a true positive rate of 0.6140 and a positive predictive value of 0.6398 in stenosis detection, underscoring its clinical applicability. These findings suggest that FAD-Net holds significant potential to assist in the accurate diagnosis and treatment planning of CAD.
* 35 pages, 12 figures
Via
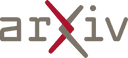
Jun 13, 2025
Abstract:Diabetes has emerged as a significant global health issue, especially with the increasing number of cases in many countries. This trend Underlines the need for a greater emphasis on early detection and proactive management to avert or mitigate the severe health complications of this disease. Over recent years, machine learning algorithms have shown promising potential in predicting diabetes risk and are beneficial for practitioners. Objective: This study highlights the prediction capabilities of statistical and non-statistical machine learning methods over Diabetes risk classification in 768 samples from the Pima Indians Diabetes Database. It consists of the significant demographic and clinical features of age, body mass index (BMI) and blood glucose levels that greatly depend on the vulnerability against Diabetes. The experimentation assesses the various types of machine learning algorithms in terms of accuracy and effectiveness regarding diabetes prediction. These algorithms include Logistic Regression, Decision Tree, Random Forest, K-Nearest Neighbors, Naive Bayes, Support Vector Machine, Gradient Boosting and Neural Network Models. The results show that the Neural Network algorithm gained the highest predictive accuracy with 78,57 %, and then the Random Forest algorithm had the second position with 76,30 % accuracy. These findings show that machine learning techniques are not just highly effective. Still, they also can potentially act as early screening tools in predicting Diabetes within a data-driven fashion with valuable information on who is more likely to get affected. In addition, this study can help to realize the potential of machine learning for timely intervention over the longer term, which is a step towards reducing health outcomes and disease burden attributable to Diabetes on healthcare systems
* Data and Metadata [Internet]. 2025 Feb. 18 [cited 2025 Jun.
11];4:545
Via
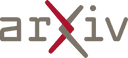
Jun 14, 2025
Abstract:Children's emotional development fundamentally relies on secure attachment relationships, yet current AI companions lack the theoretical foundation to provide developmentally appropriate emotional support. We introduce DinoCompanion, the first attachment-theory-grounded multimodal robot for emotionally responsive child-AI interaction. We address three critical challenges in child-AI systems: the absence of developmentally-informed AI architectures, the need to balance engagement with safety, and the lack of standardized evaluation frameworks for attachment-based capabilities. Our contributions include: (i) a multimodal dataset of 128 caregiver-child dyads containing 125,382 annotated clips with paired preference-risk labels, (ii) CARPO (Child-Aware Risk-calibrated Preference Optimization), a novel training objective that maximizes engagement while applying epistemic-uncertainty-weighted risk penalties, and (iii) AttachSecure-Bench, a comprehensive evaluation benchmark covering ten attachment-centric competencies with strong expert consensus (\k{appa}=0.81). DinoCompanion achieves state-of-the-art performance (57.15%), outperforming GPT-4o (50.29%) and Claude-3.7-Sonnet (53.43%), with exceptional secure base behaviors (72.99%, approaching human expert levels of 78.4%) and superior attachment risk detection (69.73%). Ablations validate the critical importance of multimodal fusion, uncertainty-aware risk modeling, and hierarchical memory for coherent, emotionally attuned interactions.
Via
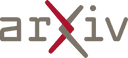
Jun 06, 2025
Abstract:Spiking Neural Networks (SNNs) are noted for their brain-like computation and energy efficiency, but their performance lags behind Artificial Neural Networks (ANNs) in tasks like image classification and object detection due to the limited representational capacity. To address this, we propose a novel spiking neuron, Integer Binary-Range Alignment Leaky Integrate-and-Fire to exponentially expand the information expression capacity of spiking neurons with only a slight energy increase. This is achieved through Integer Binary Leaky Integrate-and-Fire and range alignment strategy. The Integer Binary Leaky Integrate-and-Fire allows integer value activation during training and maintains spike-driven dynamics with binary conversion expands virtual timesteps during inference. The range alignment strategy is designed to solve the spike activation limitation problem where neurons fail to activate high integer values. Experiments show our method outperforms previous SNNs, achieving 74.19% accuracy on ImageNet and 66.2% mAP@50 and 49.1% mAP@50:95 on COCO, surpassing previous bests with the same architecture by +3.45% and +1.6% and +1.8%, respectively. Notably, our SNNs match or exceed ANNs' performance with the same architecture, and the energy efficiency is improved by 6.3${\times}$.
* 11 pages
Via
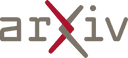
Jun 06, 2025
Abstract:Vision transformers have been widely explored in various vision tasks. Due to heavy computational cost, much interest has aroused for compressing vision transformer dynamically in the aspect of tokens. Current methods mainly pay attention to token pruning or merging to reduce token numbers, in which tokens are compressed exclusively, causing great information loss and therefore post-training is inevitably required to recover the performance. In this paper, we rethink token reduction and unify the process as an explicit form of token matrix transformation, in which all existing methods are constructing special forms of matrices within the framework. Furthermore, we propose a many-to-many Token Transforming framework that serves as a generalization of all existing methods and reserves the most information, even enabling training-free acceleration. We conduct extensive experiments to validate our framework. Specifically, we reduce 40% FLOPs and accelerate DeiT-S by $\times$1.5 with marginal 0.1% accuracy drop. Furthermore, we extend the method to dense prediction tasks including segmentation, object detection, depth estimation, and language model generation. Results demonstrate that the proposed method consistently achieves substantial improvements, offering a better computation-performance trade-off, impressive budget reduction and inference acceleration.
Via
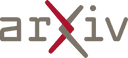