Topic:Image To Image Translation
What is Image To Image Translation? Image-to-image translation is the process of converting an image from one domain to another using deep learning techniques.
Papers and Code
Jul 19, 2024
Abstract:Sketches reflect the drawing style of individual artists; therefore, it is important to consider their unique styles when extracting sketches from color images for various applications. Unfortunately, most existing sketch extraction methods are designed to extract sketches of a single style. Although there have been some attempts to generate various style sketches, the methods generally suffer from two limitations: low quality results and difficulty in training the model due to the requirement of a paired dataset. In this paper, we propose a novel multi-modal sketch extraction method that can imitate the style of a given reference sketch with unpaired data training in a semi-supervised manner. Our method outperforms state-of-the-art sketch extraction methods and unpaired image translation methods in both quantitative and qualitative evaluations.
* ACM Transactions on Graphics (TOG) 2023, Volume 42, Issue 4
Article No.: 56, Pages 1 - 12
* Main paper 1-12 page, Supplementary 13-34 page
Via
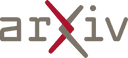
Jul 18, 2024
Abstract:Current multimodal machine translation (MMT) systems rely on fully supervised data (i.e models are trained on sentences with their translations and accompanying images). However, this type of data is costly to collect, limiting the extension of MMT to other language pairs for which such data does not exist. In this work, we propose a method to bypass the need for fully supervised data to train MMT systems, using multimodal English data only. Our method, called ZeroMMT, consists in adapting a strong text-only machine translation (MT) model by training it on a mixture of two objectives: visually conditioned masked language modelling and the Kullback-Leibler divergence between the original and new MMT outputs. We evaluate on standard MMT benchmarks and the recently released CoMMuTE, a contrastive benchmark aiming to evaluate how well models use images to disambiguate English sentences. We obtain disambiguation performance close to state-of-the-art MMT models trained additionally on fully supervised examples. To prove that our method generalizes to languages with no fully supervised training data available, we extend the CoMMuTE evaluation dataset to three new languages: Arabic, Russian and Chinese. We further show that we can control the trade-off between disambiguation capabilities and translation fidelity at inference time using classifier-free guidance and without any additional data. Our code, data and trained models are publicly accessible.
* Preprint. Under review
Via
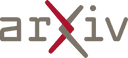
Jul 19, 2024
Abstract:This study addresses the nuanced challenge of estimating head translations within the context of six-degrees-of-freedom (6DoF) head pose estimation, placing emphasis on this aspect over the more commonly studied head rotations. Identifying a gap in existing methodologies, we recognized the underutilized potential synergy between facial geometry and head translation. To bridge this gap, we propose a novel approach called the head Translation, Rotation, and face Geometry network (TRG), which stands out for its explicit bidirectional interaction structure. This structure has been carefully designed to leverage the complementary relationship between face geometry and head translation, marking a significant advancement in the field of head pose estimation. Our contributions also include the development of a strategy for estimating bounding box correction parameters and a technique for aligning landmarks to image. Both of these innovations demonstrate superior performance in 6DoF head pose estimation tasks. Extensive experiments conducted on ARKitFace and BIWI datasets confirm that the proposed method outperforms current state-of-the-art techniques. Codes are released at https://github.com/asw91666/TRG-Release.
Via
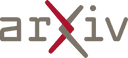
Jul 16, 2024
Abstract:Federated Learning (FL) offers a privacy-preserving approach to train models on decentralized data. Its potential in healthcare is significant, but challenges arise due to cross-client variations in medical image data, exacerbated by limited annotations. This paper introduces Cross-Client Variations Adaptive Federated Learning (CCVA-FL) to address these issues. CCVA-FL aims to minimize cross-client variations by transforming images into a common feature space. It involves expert annotation of a subset of images from each client, followed by the selection of a client with the least data complexity as the target. Synthetic medical images are then generated using Scalable Diffusion Models with Transformers (DiT) based on the target client's annotated images. These synthetic images, capturing diversity and representing the original data, are shared with other clients. Each client then translates its local images into the target image space using image-to-image translation. The translated images are subsequently used in a federated learning setting to develop a server model. Our results demonstrate that CCVA-FL outperforms Vanilla Federated Averaging by effectively addressing data distribution differences across clients without compromising privacy.
* 10 pages, 6 figures
Via
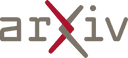
Jul 16, 2024
Abstract:Good weight initialization serves as an effective measure to reduce the training cost of a deep neural network (DNN) model. The choice of how to initialize parameters is challenging and may require manual tuning, which can be time-consuming and prone to human error. To overcome such limitations, this work takes a novel step towards building a weight generator to synthesize the neural weights for initialization. We use the image-to-image translation task with generative adversarial networks (GANs) as an example due to the ease of collecting model weights spanning a wide range. Specifically, we first collect a dataset with various image editing concepts and their corresponding trained weights, which are later used for the training of the weight generator. To address the different characteristics among layers and the substantial number of weights to be predicted, we divide the weights into equal-sized blocks and assign each block an index. Subsequently, a diffusion model is trained with such a dataset using both text conditions of the concept and the block indexes. By initializing the image translation model with the denoised weights predicted by our diffusion model, the training requires only 43.3 seconds. Compared to training from scratch (i.e., Pix2pix), we achieve a 15x training time acceleration for a new concept while obtaining even better image generation quality.
Via
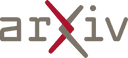
Jul 15, 2024
Abstract:Microscopy images acquired by multiple camera lenses or sensors in biological experiments offer a comprehensive understanding of the objects from diverse aspects. However, setups for multiple microscopes raise the possibility of misalignment of identical target features through different modalities. Thus, multimodal image registration is essential. In this work, we employed previous successes in biological image translation (XAcGAN) and mono-modal image registration (RoTIR) and created a deep-learning-based model, Dual-Domain RoTIR (DD_RoTIR), to address the challenges. However, it is believed that GAN-based translation models are inadequate for multimodal image registration. We facilitated the registration utilizing the feature-matching algorithm based on Transformers and rotation equivariant networks. Furthermore, hierarchical feature-matching was employed as multimodal image registration is more challenging. Results show the DD_RoTIR model presents good applicability and robustness in multiple microscopy image datasets.
* 30 pages including supporting information; 15 figures for main
context, 5 figures for supporting information; 5 tables; 5 equations in main,
12 in supporting imformation
Via
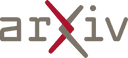
Jul 16, 2024
Abstract:Investigating the solar magnetic field is crucial to understand the physical processes in the solar interior as well as their effects on the interplanetary environment. We introduce a novel method to predict the evolution of the solar line-of-sight (LoS) magnetogram using image-to-image translation with Denoising Diffusion Probabilistic Models (DDPMs). Our approach combines "computer science metrics" for image quality and "physics metrics" for physical accuracy to evaluate model performance. The results indicate that DDPMs are effective in maintaining the structural integrity, the dynamic range of solar magnetic fields, the magnetic flux and other physical features such as the size of the active regions, surpassing traditional persistence models, also in flaring situation. We aim to use deep learning not only for visualisation but as an integrative and interactive tool for telescopes, enhancing our understanding of unexpected physical events like solar flares. Future studies will aim to integrate more diverse solar data to refine the accuracy and applicability of our generative model.
* Conference paper accepted for an oral presentation to the ESA SPAICE
CONFERENCE 17 19 September 2024
Via
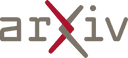
Jul 16, 2024
Abstract:This paper introduces a novel approach to image denoising that leverages the advantages of Generative Adversarial Networks (GANs). Specifically, we propose a model that combines elements of the Pix2Pix model and the Wasserstein GAN (WGAN) with Gradient Penalty (WGAN-GP). This hybrid framework seeks to capitalize on the denoising capabilities of conditional GANs, as demonstrated in the Pix2Pix model, while mitigating the need for an exhaustive search for optimal hyperparameters that could potentially ruin the stability of the learning process. In the proposed method, the GAN's generator is employed to produce denoised images, harnessing the power of a conditional GAN for noise reduction. Simultaneously, the implementation of the Lipschitz continuity constraint during updates, as featured in WGAN-GP, aids in reducing susceptibility to mode collapse. This innovative design allows the proposed model to benefit from the strong points of both Pix2Pix and WGAN-GP, generating superior denoising results while ensuring training stability. Drawing on previous work on image-to-image translation and GAN stabilization techniques, the proposed research highlights the potential of GANs as a general-purpose solution for denoising. The paper details the development and testing of this model, showcasing its effectiveness through numerical experiments. The dataset was created by adding synthetic noise to clean images. Numerical results based on real-world dataset validation underscore the efficacy of this approach in image-denoising tasks, exhibiting significant enhancements over traditional techniques. Notably, the proposed model demonstrates strong generalization capabilities, performing effectively even when trained with synthetic noise.
* Systems and Soft Computing
Via
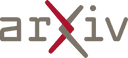
Jul 19, 2024
Abstract:Deep learning has significantly advanced the field of gastrointestinal vision, enhancing disease diagnosis capabilities. One major challenge in automating diagnosis within gastrointestinal settings is the detection of abnormal cases in endoscopic images. Due to the sparsity of data, this process of distinguishing normal from abnormal cases has faced significant challenges, particularly with rare and unseen conditions. To address this issue, we frame abnormality detection as an out-of-distribution (OOD) detection problem. In this setup, a model trained on In-Distribution (ID) data, which represents a healthy GI tract, can accurately identify healthy cases, while abnormalities are detected as OOD, regardless of their class. We introduce a test-time augmentation segment into the OOD detection pipeline, which enhances the distinction between ID and OOD examples, thereby improving the effectiveness of existing OOD methods with the same model. This augmentation shifts the pixel space, which translates into a more distinct semantic representation for OOD examples compared to ID examples. We evaluated our method against existing state-of-the-art OOD scores, showing improvements with test-time augmentation over the baseline approach.
Via
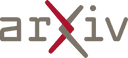
Jul 15, 2024
Abstract:In recent years, methods for producing highly realistic synthetic images have significantly advanced, allowing the creation of high-quality images from text prompts that describe the desired content. Even more impressively, Stable Diffusion (SD) models now provide users with the option of creating synthetic images in an image-to-image translation fashion, modifying images in the latent space of advanced autoencoders. This striking evolution, however, brings an alarming consequence: it is possible to pass an image through SD autoencoders to reproduce a synthetic copy of the image with high realism and almost no visual artifacts. This process, known as SD image laundering, can transform real images into lookalike synthetic ones and risks complicating forensic analysis for content authenticity verification. Our paper investigates the forensic implications of image laundering, revealing a serious potential to obscure traces of real content, including sensitive and harmful materials that could be mistakenly classified as synthetic, thereby undermining the protection of individuals depicted. To address this issue, we propose a two-stage detection pipeline that effectively differentiates between pristine, laundered, and fully synthetic images (those generated from text prompts), showing robustness across various conditions. Finally, we highlight another alarming property of image laundering, which appears to mask the unique artifacts exploited by forensic detectors to solve the camera model identification task, strongly undermining their performance. Our experimental code is available at https://github.com/polimi-ispl/synthetic-image-detection.
Via
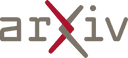