May 01, 2025
Abstract:Diffusion-based visuomotor policies generate robot motions by learning to denoise action-space trajectories conditioned on observations. These observations are commonly streams of RGB images, whose high dimensionality includes substantial task-irrelevant information, requiring large models to extract relevant patterns. In contrast, using more structured observations, such as the spatial poses (positions and orientations) of key objects over time, enables training more compact policies that can recognize relevant patterns with fewer parameters. However, obtaining accurate object poses in open-set, real-world environments remains challenging. For instance, it is impractical to assume that all relevant objects are equipped with markers, and recent learning-based 6D pose estimation and tracking methods often depend on pre-scanned object meshes, requiring manual reconstruction. In this work, we propose PRISM-DP, an approach that leverages segmentation, mesh generation, pose estimation, and pose tracking models to enable compact diffusion policy learning directly from the spatial poses of task-relevant objects. Crucially, because PRISM-DP uses a mesh generation model, it eliminates the need for manual mesh processing or creation, improving scalability and usability in open-set, real-world environments. Experiments across a range of tasks in both simulation and real-world settings show that PRISM-DP outperforms high-dimensional image-based diffusion policies and achieves performance comparable to policies trained with ground-truth state information. We conclude with a discussion of the broader implications and limitations of our approach.
Via
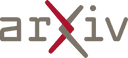