Jun 14, 2025
Abstract:Numerous studies have proposed computational methods to detect hate speech online, yet most focus on the English language and emphasize model development. In this study, we evaluate the counterfactual fairness of hate speech detection models in the Dutch language, specifically examining the performance and fairness of transformer-based models. We make the following key contributions. First, we curate a list of Dutch Social Group Terms that reflect social context. Second, we generate counterfactual data for Dutch hate speech using LLMs and established strategies like Manual Group Substitution (MGS) and Sentence Log-Likelihood (SLL). Through qualitative evaluation, we highlight the challenges of generating realistic counterfactuals, particularly with Dutch grammar and contextual coherence. Third, we fine-tune baseline transformer-based models with counterfactual data and evaluate their performance in detecting hate speech. Fourth, we assess the fairness of these models using Counterfactual Token Fairness (CTF) and group fairness metrics, including equality of odds and demographic parity. Our analysis shows that models perform better in terms of hate speech detection, average counterfactual fairness and group fairness. This work addresses a significant gap in the literature on counterfactual fairness for hate speech detection in Dutch and provides practical insights and recommendations for improving both model performance and fairness.
* Accepted for publication at the 9th Workshop on Online Abuse and
Harms (WOAH) held in conjunction with ACL 2025
Via
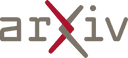