What is speech recognition? Speech recognition is the task of identifying words spoken aloud, analyzing the voice and language, and accurately transcribing the words.
Papers and Code
May 26, 2025
Abstract:The development of high-performing, robust, and reliable speech technologies depends on large, high-quality datasets. However, African languages -- including our focus, Igbo, Hausa, and Yoruba -- remain under-represented due to insufficient data. Popular voice-enabled technologies do not support any of the 2000+ African languages, limiting accessibility for circa one billion people. While previous dataset efforts exist for the target languages, they lack the scale and diversity needed for robust speech models. To bridge this gap, we introduce the NaijaVoices dataset, a 1,800-hour speech-text dataset with 5,000+ speakers. We outline our unique data collection approach, analyze its acoustic diversity, and demonstrate its impact through finetuning experiments on automatic speech recognition, averagely achieving 75.86% (Whisper), 52.06% (MMS), and 42.33% (XLSR) WER improvements. These results highlight NaijaVoices' potential to advance multilingual speech processing for African languages.
* Accepted for publication at Interspeech 2025
Via
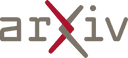
May 20, 2025
Abstract:Automatic speech recognition (ASR) for dysarthric speech remains challenging due to data scarcity, particularly in non-English languages. To address this, we fine-tune a voice conversion model on English dysarthric speech (UASpeech) to encode both speaker characteristics and prosodic distortions, then apply it to convert healthy non-English speech (FLEURS) into non-English dysarthric-like speech. The generated data is then used to fine-tune a multilingual ASR model, Massively Multilingual Speech (MMS), for improved dysarthric speech recognition. Evaluation on PC-GITA (Spanish), EasyCall (Italian), and SSNCE (Tamil) demonstrates that VC with both speaker and prosody conversion significantly outperforms the off-the-shelf MMS performance and conventional augmentation techniques such as speed and tempo perturbation. Objective and subjective analyses of the generated data further confirm that the generated speech simulates dysarthric characteristics.
* 5 pages, 1 figure, Accepted to Interspeech 2025
Via
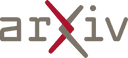
May 23, 2025
Abstract:Speech emotion recognition (SER) in naturalistic settings remains a challenge due to the intrinsic variability, diverse recording conditions, and class imbalance. As participants in the Interspeech Naturalistic SER Challenge which focused on these complexities, we present Abhinaya, a system integrating speech-based, text-based, and speech-text models. Our approach fine-tunes self-supervised and speech large language models (SLLM) for speech representations, leverages large language models (LLM) for textual context, and employs speech-text modeling with an SLLM to capture nuanced emotional cues. To combat class imbalance, we apply tailored loss functions and generate categorical decisions through majority voting. Despite one model not being fully trained, the Abhinaya system ranked 4th among 166 submissions. Upon completion of training, it achieved state-of-the-art performance among published results, demonstrating the effectiveness of our approach for SER in real-world conditions.
* 5 pages, 2 figures, 4 tables, accepted at Interspeech 2025
Via
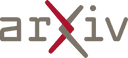
May 26, 2025
Abstract:Cross-lingual alignment in pretrained language models (LMs) has enabled efficient transfer in text-based LMs. Such an alignment has also been observed in speech foundation models. However, it remains an open question whether findings and methods from text-based cross-lingual alignment apply to speech. Building on prior work on spoken translation retrieval, we perform pronunciation-controlled experiments to observe if cross-lingual alignment can indeed occur in such models on a semantic basis, instead of relying on phonetic similarities. Our findings indicate that even in the absence of phonetic cues, spoken translation retrieval accuracy remains relatively stable. We follow up with a controlled experiment on a word-level dataset of cross-lingual synonyms and near-homophones, confirming the existence of both phonetic and semantic knowledge in the encoder. Finally, we qualitatively examine the transcriptions produced by early exiting the encoder, where we observe that speech translation produces semantic errors that are characterized by phonetic similarities to corresponding words in the source language. We apply this insight from early exiting to speech recognition in seven low-resource languages unsupported by the Whisper model, and achieve improved accuracy in all languages examined, particularly for languages with transparent orthographies.
Via
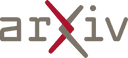
May 26, 2025
Abstract:This paper presents KIT's submissions to the IWSLT 2025 low-resource track. We develop both cascaded systems, consisting of Automatic Speech Recognition (ASR) and Machine Translation (MT) models, and end-to-end (E2E) Speech Translation (ST) systems for three language pairs: Bemba, North Levantine Arabic, and Tunisian Arabic into English. Building upon pre-trained models, we fine-tune our systems with different strategies to utilize resources efficiently. This study further explores system enhancement with synthetic data and model regularization. Specifically, we investigate MT-augmented ST by generating translations from ASR data using MT models. For North Levantine, which lacks parallel ST training data, a system trained solely on synthetic data slightly surpasses the cascaded system trained on real data. We also explore augmentation using text-to-speech models by generating synthetic speech from MT data, demonstrating the benefits of synthetic data in improving both ASR and ST performance for Bemba. Additionally, we apply intra-distillation to enhance model performance. Our experiments show that this approach consistently improves results across ASR, MT, and ST tasks, as well as across different pre-trained models. Finally, we apply Minimum Bayes Risk decoding to combine the cascaded and end-to-end systems, achieving an improvement of approximately 1.5 BLEU points.
Via
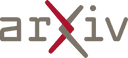
May 20, 2025
Abstract:Sign Language Recognition (SLR) systems primarily focus on manual gestures, but non-manual features such as mouth movements, specifically mouthing, provide valuable linguistic information. This work directly classifies mouthing instances to their corresponding words in the spoken language while exploring the potential of transfer learning from Visual Speech Recognition (VSR) to mouthing recognition in German Sign Language. We leverage three VSR datasets: one in English, one in German with unrelated words and one in German containing the same target words as the mouthing dataset, to investigate the impact of task similarity in this setting. Our results demonstrate that multi-task learning improves both mouthing recognition and VSR accuracy as well as model robustness, suggesting that mouthing recognition should be treated as a distinct but related task to VSR. This research contributes to the field of SLR by proposing knowledge transfer from VSR to SLR datasets with limited mouthing annotations.
* Accepted at 19th IEEE International Conference on Automatic Face and
Gesture Recognition 2025
Via
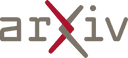
May 24, 2025
Abstract:Automatic Speech Recognition (ASR) systems struggle with child speech due to its distinct acoustic and linguistic variability and limited availability of child speech datasets, leading to high transcription error rates. While ASR error correction (AEC) methods have improved adult speech transcription, their effectiveness on child speech remains largely unexplored. To address this, we introduce CHSER, a Generative Speech Error Correction (GenSEC) dataset for child speech, comprising 200K hypothesis-transcription pairs spanning diverse age groups and speaking styles. Results demonstrate that fine-tuning on the CHSER dataset achieves up to a 28.5% relative WER reduction in a zero-shot setting and a 13.3% reduction when applied to fine-tuned ASR systems. Additionally, our error analysis reveals that while GenSEC improves substitution and deletion errors, it struggles with insertions and child-specific disfluencies. These findings highlight the potential of GenSEC for improving child ASR.
* Accepted in Interspeech 2025
Via
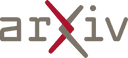
May 23, 2025
Abstract:In our prior works, we introduced a scalable streaming speech synthesis model, CosyVoice 2, which integrates a large language model (LLM) and a chunk-aware flow matching (FM) model, and achieves low-latency bi-streaming speech synthesis and human-parity quality. Despite these advancements, CosyVoice 2 exhibits limitations in language coverage, domain diversity, data volume, text formats, and post-training techniques. In this paper, we present CosyVoice 3, an improved model designed for zero-shot multilingual speech synthesis in the wild, surpassing its predecessor in content consistency, speaker similarity, and prosody naturalness. Key features of CosyVoice 3 include: 1) A novel speech tokenizer to improve prosody naturalness, developed via supervised multi-task training, including automatic speech recognition, speech emotion recognition, language identification, audio event detection, and speaker analysis. 2) A new differentiable reward model for post-training applicable not only to CosyVoice 3 but also to other LLM-based speech synthesis models. 3) Dataset Size Scaling: Training data is expanded from ten thousand hours to one million hours, encompassing 9 languages and 18 Chinese dialects across various domains and text formats. 4) Model Size Scaling: Model parameters are increased from 0.5 billion to 1.5 billion, resulting in enhanced performance on our multilingual benchmark due to the larger model capacity. These advancements contribute significantly to the progress of speech synthesis in the wild. We encourage readers to listen to the demo at https://funaudiollm.github.io/cosyvoice3.
* Preprint, work in progress
Via
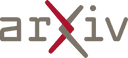
May 21, 2025
Abstract:We introduce a data-driven approach for enabling word-level timestamp prediction in the Canary model. Accurate timestamp information is crucial for a variety of downstream tasks such as speech content retrieval and timed subtitles. While traditional hybrid systems and end-to-end (E2E) models may employ external modules for timestamp prediction, our approach eliminates the need for separate alignment mechanisms. By leveraging the NeMo Forced Aligner (NFA) as a teacher model, we generate word-level timestamps and train the Canary model to predict timestamps directly. We introduce a new <|timestamp|> token, enabling the Canary model to predict start and end timestamps for each word. Our method demonstrates precision and recall rates between 80% and 90%, with timestamp prediction errors ranging from 20 to 120 ms across four languages, with minimal WER degradation. Additionally, we extend our system to automatic speech translation (AST) tasks, achieving timestamp prediction errors around 200 milliseconds.
* Accepted to Interspeech 2025
Via
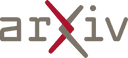
May 24, 2025
Abstract:In this paper, we introduce the first publicly available English-Kpelle dataset for machine translation, comprising over 2000 sentence pairs drawn from everyday communication, religious texts, and educational materials. By fine-tuning Meta's No Language Left Behind(NLLB) model on two versions of the dataset, we achieved BLEU scores of up to 30 in the Kpelle-to-English direction, demonstrating the benefits of data augmentation. Our findings align with NLLB-200 benchmarks on other African languages, underscoring Kpelle's potential for competitive performance despite its low-resource status. Beyond machine translation, this dataset enables broader NLP tasks, including speech recognition and language modelling. We conclude with a roadmap for future dataset expansion, emphasizing orthographic consistency, community-driven validation, and interdisciplinary collaboration to advance inclusive language technology development for Kpelle and other low-resourced Mande languages.
Via
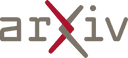