Jul 03, 2025
Abstract:Recently, the remarkable success of large language models (LLMs) has achieved a profound impact on the field of artificial intelligence. Numerous advanced works based on LLMs have been proposed and applied in various scenarios. Among them, video language models (VidLMs) are particularly widely used. However, existing works primarily focus on terrestrial scenarios, overlooking the highly demanding application needs of underwater observation. To overcome this gap, we introduce UVLM, an under water observation benchmark which is build through a collaborative approach combining human expertise and AI models. To ensure data quality, we have conducted in-depth considerations from multiple perspectives. First, to address the unique challenges of underwater environments, we selected videos that represent typical underwater challenges including light variations, water turbidity, and diverse viewing angles to construct the dataset. Second, to ensure data diversity, the dataset covers a wide range of frame rates, resolutions, 419 classes of marine animals, and various static plants and terrains. Next, for task diversity, we adopted a structured design where observation targets are categorized into two major classes: biological and environmental. Each category includes content observation and change/action observation, totaling 20 distinct task types. Finally, we designed several challenging evaluation metrics to enable quantitative comparison and analysis of different methods. Experiments on two representative VidLMs demonstrate that fine-tuning VidLMs on UVLM significantly improves underwater world understanding while also showing potential for slight improvements on existing in-air VidLM benchmarks, such as VideoMME and Perception text. The dataset and prompt engineering will be released publicly.
* 13 pages, 4 figures, 3 tables
Via
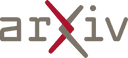