What is Recommendation? Recommendation is the task of providing personalized suggestions to users based on their preferences and behavior.
Papers and Code
May 28, 2025
Abstract:Embedding-based collaborative filtering, often coupled with nearest neighbor search, is widely deployed in large-scale recommender systems for personalized content selection. Modern systems leverage multiple implicit feedback signals (e.g., clicks, add to cart, purchases) to model user preferences comprehensively. However, prevailing approaches adopt a feedback-wise modeling paradigm, which (1) fails to capture the structured progression of user engagement entailed among different feedback and (2) embeds feedback-specific information into disjoint spaces, making representations incommensurable, increasing system complexity, and leading to suboptimal retrieval performance. A promising alternative is Ordinal Logistic Regression (OLR), which explicitly models discrete ordered relations. However, existing OLR-based recommendation models mainly focus on explicit feedback (e.g., movie ratings) and struggle with implicit, correlated feedback, where ordering is vague and non-linear. Moreover, standard OLR lacks flexibility in handling feedback-dependent covariates, resulting in suboptimal performance in real-world systems. To address these limitations, we propose Generalized Neural Ordinal Logistic Regression (GNOLR), which encodes multiple feature-feedback dependencies into a unified, structured embedding space and enforces feedback-specific dependency learning through a nested optimization framework. Thus, GNOLR enhances predictive accuracy, captures the progression of user engagement, and simplifies the retrieval process. We establish a theoretical comparison with existing paradigms, demonstrating how GNOLR avoids disjoint spaces while maintaining effectiveness. Extensive experiments on ten real-world datasets show that GNOLR significantly outperforms state-of-the-art methods in efficiency and adaptability.
Via
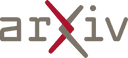
May 28, 2025
Abstract:In online video platforms, accurate watch time prediction has become a fundamental and challenging problem in video recommendation. Previous research has revealed that the accuracy of watch time prediction highly depends on both the transformation of watch-time labels and the decomposition of the estimation process. TPM (Tree based Progressive Regression Model) achieves State-of-the-Art performance with a carefully designed and effective decomposition paradigm. TPM discretizes the watch time into several ordinal intervals and organizes them into a binary decision tree, where each node corresponds to a specific interval. At each non-leaf node, a binary classifier is used to determine the specific interval in which the watch time variable most likely falls, based on the prediction outcome at its parent node. The tree structure serves as the core of TPM, as it defines the decomposition of watch time estimation and determines how the ordinal intervals are discretized. However, in TPM, the tree is predefined as a full binary tree, which may be sub-optimal for the following reasons. First, a full binary tree implies an equal partitioning of the watch time space, which may struggle to capture the complexity of real-world watch time distributions. Second, instead of relying on a globally fixed tree structure, we advocate for a personalized, data-driven tree that can be learned in an end-to-end manner. Therefore, we propose PTPM to enable a highly personalized decomposition of watch estimation with better efficacy and efficiency. Moreover, we reveal that TPM is affected by selection bias due to conditional modeling and devise a simple approach to address it. We conduct extensive experiments on both offline datasets and online environments. PTPM has been fully deployed in core traffic scenarios and serves more than 400 million users per day.
Via
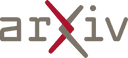
May 28, 2025
Abstract:If AI models can detect when they are being evaluated, the effectiveness of evaluations might be compromised. For example, models could have systematically different behavior during evaluations, leading to less reliable benchmarks for deployment and governance decisions. We investigate whether frontier language models can accurately classify transcripts based on whether they originate from evaluations or real-world deployment, a capability we call evaluation awareness. To achieve this, we construct a diverse benchmark of 1,000 prompts and transcripts from 61 distinct datasets. These span public benchmarks (e.g., MMLU, SWEBench), real-world deployment interactions, and agent trajectories from scaffolding frameworks (e.g., web-browsing agents). Frontier models clearly demonstrate above-random evaluation awareness (Gemini-2.5-Pro reaches an AUC of $0.83$), but do not yet surpass our simple human baseline (AUC of $0.92$). Furthermore, both AI models and humans are better at identifying evaluations in agentic settings compared to chat settings. Additionally, we test whether models can identify the purpose of the evaluation. Under multiple-choice and open-ended questioning, AI models far outperform random chance in identifying what an evaluation is testing for. Our results indicate that frontier models already exhibit a substantial, though not yet superhuman, level of evaluation-awareness. We recommend tracking this capability in future models.
Via
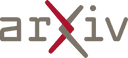
May 28, 2025
Abstract:We present the Star-Based Automated Single-locus and Epistasis analysis tool - Genetic Programming (StarBASE-GP), an automated framework for discovering meaningful genetic variants associated with phenotypic variation in large-scale genomic datasets. StarBASE-GP uses a genetic programming-based multi-objective optimization strategy to evolve machine learning pipelines that simultaneously maximize explanatory power (r2) and minimize pipeline complexity. Biological domain knowledge is integrated at multiple stages, including the use of nine inheritance encoding strategies to model deviations from additivity, a custom linkage disequilibrium pruning node that minimizes redundancy among features, and a dynamic variant recommendation system that prioritizes informative candidates for pipeline inclusion. We evaluate StarBASE-GP on a cohort of Rattus norvegicus (brown rat) to identify variants associated with body mass index, benchmarking its performance against a random baseline and a biologically naive version of the tool. StarBASE-GP consistently evolves Pareto fronts with superior performance, yielding higher accuracy in identifying both ground truth and novel quantitative trait loci, highlighting relevant targets for future validation. By incorporating evolutionary search and relevant biological theory into a flexible automated machine learning framework, StarBASE-GP demonstrates robust potential for advancing variant discovery in complex traits.
Via
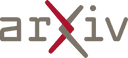
May 28, 2025
Abstract:User-item interaction histories are pivotal for sequential recommendation systems but often include noise, such as unintended clicks or actions that fail to reflect genuine user preferences. To address this issue, we propose the User-Consistent Preference-based Sequential Recommendation System (ConsRec), designed to capture stable user preferences and filter noisy items from interaction histories. Specifically, ConsRec constructs a user-interacted item graph, learns item similarities from their text representations, and then extracts the maximum connected subgraph from the user-interacted item graph for denoising items. Experimental results on the Yelp and Amazon Product datasets illustrate that ConsRec achieves a 13% improvement over baseline recommendation models, showing its effectiveness in denoising user-interacted items. Further analysis reveals that the denoised interaction histories form semantically tighter clusters of user-preferred items, leading to higher relevance scores for ground-truth targets and more accurate recommendations. All codes are available at https://github.com/NEUIR/ConsRec.
Via
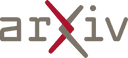
May 27, 2025
Abstract:Personalized programming tutoring, such as exercise recommendation, can enhance learners' efficiency, motivation, and outcomes, which is increasingly important in modern digital education. However, the lack of sufficient and high-quality programming data, combined with the mismatch between offline evaluation and real-world learning, hinders the practical deployment of such systems. To address this challenge, many approaches attempt to simulate learner practice data, yet they often overlook the fine-grained, iterative nature of programming learning, resulting in a lack of interpretability and granularity. To fill this gap, we propose a LLM-based agent, CoderAgent, to simulate students' programming processes in a fine-grained manner without relying on real data. Specifically, we equip each human learner with an intelligent agent, the core of which lies in capturing the cognitive states of the human programming practice process. Inspired by ACT-R, a cognitive architecture framework, we design the structure of CoderAgent to align with human cognitive architecture by focusing on the mastery of programming knowledge and the application of coding ability. Recognizing the inherent patterns in multi-layered cognitive reasoning, we introduce the Programming Tree of Thought (PTOT), which breaks down the process into four steps: why, how, where, and what. This approach enables a detailed analysis of iterative problem-solving strategies. Finally, experimental evaluations on real-world datasets demonstrate that CoderAgent provides interpretable insights into learning trajectories and achieves accurate simulations, paving the way for personalized programming education.
* Accepted by IJCAI2025
Via
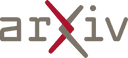
May 27, 2025
Abstract:This study explores the integration of eXtreme Programming (XP) and the Cross-Industry Standard Process for Data Mining (CRISP-DM) in agile Data Science projects. We conducted a case study at the e-commerce company Elo7 to answer the research question: How can the agility of the XP method be integrated with CRISP-DM in Data Science projects? Data was collected through interviews and questionnaires with a Data Science team consisting of data scientists, ML engineers, and data product managers. The results show that 86% of the team frequently or always applies CRISP-DM, while 71% adopt XP practices in their projects. Furthermore, the study demonstrates that it is possible to combine CRISP-DM with XP in Data Science projects, providing a structured and collaborative approach. Finally, the study generated improvement recommendations for the company.
Via
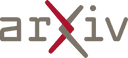
May 27, 2025
Abstract:Traditional photography composition approaches are dominated by 2D cropping-based methods. However, these methods fall short when scenes contain poorly arranged subjects. Professional photographers often employ perspective adjustment as a form of 3D recomposition, modifying the projected 2D relationships between subjects while maintaining their actual spatial positions to achieve better compositional balance. Inspired by this artistic practice, we propose photography perspective composition (PPC), extending beyond traditional cropping-based methods. However, implementing the PPC faces significant challenges: the scarcity of perspective transformation datasets and undefined assessment criteria for perspective quality. To address these challenges, we present three key contributions: (1) An automated framework for building PPC datasets through expert photographs. (2) A video generation approach that demonstrates the transformation process from suboptimal to optimal perspectives. (3) A perspective quality assessment (PQA) model constructed based on human performance. Our approach is concise and requires no additional prompt instructions or camera trajectories, helping and guiding ordinary users to enhance their composition skills.
Via
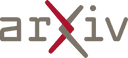
May 27, 2025
Abstract:Sequential recommendation is a popular paradigm in modern recommender systems. In particular, one challenging problem in this space is cross-domain sequential recommendation (CDSR), which aims to predict future behaviors given user interactions across multiple domains. Existing CDSR frameworks are mostly built on the self-attention transformer and seek to improve by explicitly injecting additional domain-specific components (e.g. domain-aware module blocks). While these additional components help, we argue they overlook the core self-attention module already present in the transformer, a naturally powerful tool to learn correlations among behaviors. In this work, we aim to improve the CDSR performance for simple models from a novel perspective of enhancing the self-attention. Specifically, we introduce a Pareto-optimal self-attention and formulate the cross-domain learning as a multi-objective problem, where we optimize the recommendation task while dynamically minimizing the cross-domain attention scores. Our approach automates knowledge transfer in CDSR (dubbed as AutoCDSR) -- it not only mitigates negative transfer but also encourages complementary knowledge exchange among auxiliary domains. Based on the idea, we further introduce AutoCDSR+, a more performant variant with slight additional cost. Our proposal is easy to implement and works as a plug-and-play module that can be incorporated into existing transformer-based recommenders. Besides flexibility, it is practical to deploy because it brings little extra computational overheads without heavy hyper-parameter tuning. AutoCDSR on average improves Recall@10 for SASRec and Bert4Rec by 9.8% and 16.0% and NDCG@10 by 12.0% and 16.7%, respectively. Code is available at https://github.com/snap-research/AutoCDSR.
* Accepted to KDD'25
Via
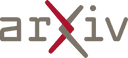
May 27, 2025
Abstract:As generative AI systems become widely adopted, they enable unprecedented creation levels of synthetic data across text, images, audio, and video modalities. While research has addressed the energy consumption of model training and inference, a critical sustainability challenge remains understudied: digital waste. This term refers to stored data that consumes resources without serving a specific (and/or immediate) purpose. This paper presents this terminology in the AI context and introduces digital waste as an ethical imperative within (generative) AI development, positioning environmental sustainability as core for responsible innovation. Drawing from established digital resource management approaches, we examine how other disciplines manage digital waste and identify transferable approaches for the AI community. We propose specific recommendations encompassing re-search directions, technical interventions, and cultural shifts to mitigate the environmental consequences of in-definite data storage. By expanding AI ethics beyond immediate concerns like bias and privacy to include inter-generational environmental justice, this work contributes to a more comprehensive ethical framework that considers the complete lifecycle impact of generative AI systems.
* 8 pages, submitted to AAAI/ACM Conference on AI, Ethics and Society
Via
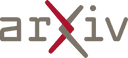