Topic:Image To Image Translation
What is Image To Image Translation? Image-to-image translation is the process of converting an image from one domain to another using deep learning techniques.
Papers and Code
Jun 24, 2025
Abstract:With access to large-scale, unlabeled medical datasets, researchers are confronted with two questions: Should they attempt to pretrain a custom foundation model on this medical data, or use transfer-learning from an existing generalist model? And, if a custom model is pretrained, are novel methods required? In this paper we explore these questions by conducting a case-study, in which we train a foundation model on a large regional fetal ultrasound dataset of 2M images. By selecting the well-established DINOv2 method for pretraining, we achieve state-of-the-art results on three fetal ultrasound datasets, covering data from different countries, classification, segmentation, and few-shot tasks. We compare against a series of models pretrained on natural images, ultrasound images, and supervised baselines. Our results demonstrate two key insights: (i) Pretraining on custom data is worth it, even if smaller models are trained on less data, as scaling in natural image pretraining does not translate to ultrasound performance. (ii) Well-tuned methods from computer vision are making it feasible to train custom foundation models for a given medical domain, requiring no hyperparameter tuning and little methodological adaptation. Given these findings, we argue that a bias towards methodological innovation should be avoided when developing domain specific foundation models under common computational resource constraints.
* Submitted version of paper accepted at MICCAI 2025
Via
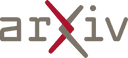
Jun 24, 2025
Abstract:Vascular diseases pose a significant threat to human health, with X-ray angiography established as the gold standard for diagnosis, allowing for detailed observation of blood vessels. However, angiographic X-rays expose personnel and patients to higher radiation levels than non-angiographic X-rays, which are unwanted. Thus, modality translation from non-angiographic to angiographic X-rays is desirable. Data-driven deep approaches are hindered by the lack of paired large-scale X-ray angiography datasets. While making high-quality vascular angiography synthesis crucial, it remains challenging. We find that current medical image synthesis primarily operates at pixel level and struggles to adapt to the complex geometric structure of blood vessels, resulting in unsatisfactory quality of blood vessel image synthesis, such as disconnections or unnatural curvatures. To overcome this issue, we propose a self-supervised method via diffusion models to transform non-angiographic X-rays into angiographic X-rays, mitigating data shortages for data-driven approaches. Our model comprises a diffusion model that learns the distribution of vascular data from diffusion latent, a generator for vessel synthesis, and a mask-based adversarial module. To enhance geometric accuracy, we propose a parametric vascular model to fit the shape and distribution of blood vessels. The proposed method contributes a pipeline and a synthetic dataset for X-ray angiography. We conducted extensive comparative and ablation experiments to evaluate the Angio-Diff. The results demonstrate that our method achieves state-of-the-art performance in synthetic angiography image quality and more accurately synthesizes the geometric structure of blood vessels. The code is available at https://github.com/zfw-cv/AngioDiff.
Via
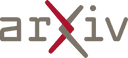
Jun 17, 2025
Abstract:We introduce a model named DreamLight for universal image relighting in this work, which can seamlessly composite subjects into a new background while maintaining aesthetic uniformity in terms of lighting and color tone. The background can be specified by natural images (image-based relighting) or generated from unlimited text prompts (text-based relighting). Existing studies primarily focus on image-based relighting, while with scant exploration into text-based scenarios. Some works employ intricate disentanglement pipeline designs relying on environment maps to provide relevant information, which grapples with the expensive data cost required for intrinsic decomposition and light source. Other methods take this task as an image translation problem and perform pixel-level transformation with autoencoder architecture. While these methods have achieved decent harmonization effects, they struggle to generate realistic and natural light interaction effects between the foreground and background. To alleviate these challenges, we reorganize the input data into a unified format and leverage the semantic prior provided by the pretrained diffusion model to facilitate the generation of natural results. Moreover, we propose a Position-Guided Light Adapter (PGLA) that condenses light information from different directions in the background into designed light query embeddings, and modulates the foreground with direction-biased masked attention. In addition, we present a post-processing module named Spectral Foreground Fixer (SFF) to adaptively reorganize different frequency components of subject and relighted background, which helps enhance the consistency of foreground appearance. Extensive comparisons and user study demonstrate that our DreamLight achieves remarkable relighting performance.
Via
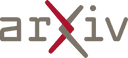
Jun 24, 2025
Abstract:Accessibility remains a critical concern in today's society, as many technologies are not developed to support the full range of user needs. Existing multi-agent systems (MAS) often cannot provide comprehensive assistance for users in need due to the lack of customization stemming from closed-source designs. Consequently, individuals with disabilities frequently encounter significant barriers when attempting to interact with digital environments. We introduce MATE, a multimodal accessibility MAS, which performs the modality conversions based on the user's needs. The system is useful for assisting people with disabilities by ensuring that data will be converted to an understandable format. For instance, if the user cannot see well and receives an image, the system converts this image to its audio description. MATE can be applied to a wide range of domains, industries, and areas, such as healthcare, and can become a useful assistant for various groups of users. The system supports multiple types of models, ranging from LLM API calling to using custom machine learning (ML) classifiers. This flexibility ensures that the system can be adapted to various needs and is compatible with a wide variety of hardware. Since the system is expected to run locally, it ensures the privacy and security of sensitive information. In addition, the framework can be effectively integrated with institutional technologies (e.g., digital healthcare service) for real-time user assistance. Furthermore, we introduce ModCon-Task-Identifier, a model that is capable of extracting the precise modality conversion task from the user input. Numerous experiments show that ModCon-Task-Identifier consistently outperforms other LLMs and statistical models on our custom data. Our code and data are publicly available at https://github.com/AlgazinovAleksandr/Multi-Agent-MATE.
Via
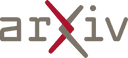
Jun 18, 2025
Abstract:Recent Multimodal Large Language Models (MLLMs) excel on benchmark vision-language tasks, yet little is known about how input visual quality shapes their responses. Does higher perceptual quality of images already translate to better MLLM understanding? We conduct the first systematic study spanning leading MLLMs and a suite of vision-language benchmarks, applying controlled degradations and stylistic shifts to each image. Surprisingly, we uncover a visual-quality paradox: model, task, and even individual-instance performance can improve when images deviate from human-perceived fidelity. Off-the-shelf restoration pipelines fail to reconcile these idiosyncratic preferences. To close the gap, we introduce Visual-Quality Test-Time Tuning (VQ-TTT)-a lightweight adaptation module that: (1) inserts a learnable, low-rank kernel before the frozen vision encoder to modulate frequency content; and (2) fine-tunes only shallow vision-encoder layers via LoRA. VQ-TTT dynamically adjusts each input image in a single forward pass, aligning it with task-specific model preferences. Across the evaluated MLLMs and all datasets, VQ-TTT lifts significant average accuracy, with no external models, cached features, or extra training data. These findings redefine ``better'' visual inputs for MLLMs and highlight the need for adaptive, rather than universally ``clean'', imagery, in the new era of AI being the main data customer.
* 18 pages
Via
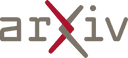
Jun 17, 2025
Abstract:Generative AI, specifically text-to-image models, have revolutionized interior architectural design by enabling the rapid translation of conceptual ideas into visual representations from simple text prompts. While generative AI can produce visually appealing images they often lack actionable data for designers In this work, we propose a novel pipeline that integrates DALL-E 3 with a materials dataset to enrich AI-generated designs with sustainability metrics and material usage insights. After the model generates an interior design image, a post-processing module identifies the top ten materials present and pairs them with carbon dioxide equivalent (CO2e) values from a general materials dictionary. This approach allows designers to immediately evaluate environmental impacts and refine prompts accordingly. We evaluate the system through three user tests: (1) no mention of sustainability to the user prior to the prompting process with generative AI, (2) sustainability goals communicated to the user before prompting, and (3) sustainability goals communicated along with quantitative CO2e data included in the generative AI outputs. Our qualitative and quantitative analyses reveal that the introduction of sustainability metrics in the third test leads to more informed design decisions, however, it can also trigger decision fatigue and lower overall satisfaction. Nevertheless, the majority of participants reported incorporating sustainability principles into their workflows in the third test, underscoring the potential of integrated metrics to guide more ecologically responsible practices. Our findings showcase the importance of balancing design freedom with practical constraints, offering a clear path toward holistic, data-driven solutions in AI-assisted architectural design.
* 15 Pages, 6 figures, CAAD Futures 2025
Via
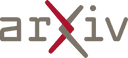
Jun 12, 2025
Abstract:Fluorodeoxyglucose (FDG) PET to evaluate patients with epilepsy is one of the most common applications for simultaneous PET/MRI, given the need to image both brain structure and metabolism, but is suboptimal due to the radiation dose in this young population. Little work has been done synthesizing diagnostic quality PET images from MRI data or MRI data with ultralow-dose PET using advanced generative AI methods, such as diffusion models, with attention to clinical evaluations tailored for the epilepsy population. Here we compared the performance of diffusion- and non-diffusion-based deep learning models for the MRI-to-PET image translation task for epilepsy imaging using simultaneous PET/MRI in 52 subjects (40 train/2 validate/10 hold-out test). We tested three different models: 2 score-based generative diffusion models (SGM-Karras Diffusion [SGM-KD] and SGM-variance preserving [SGM-VP]) and a Transformer-Unet. We report results on standard image processing metrics as well as clinically relevant metrics, including congruency measures (Congruence Index and Congruency Mean Absolute Error) that assess hemispheric metabolic asymmetry, which is a key part of the clinical analysis of these images. The SGM-KD produced the best qualitative and quantitative results when synthesizing PET purely from T1w and T2 FLAIR images with the least mean absolute error in whole-brain specific uptake value ratio (SUVR) and highest intraclass correlation coefficient. When 1% low-dose PET images are included in the inputs, all models improve significantly and are interchangeable for quantitative performance and visual quality. In summary, SGMs hold great potential for pure MRI-to-PET translation, while all 3 model types can synthesize full-dose FDG-PET accurately using MRI and ultralow-dose PET.
Via
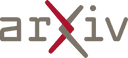
Jun 13, 2025
Abstract:Vision-Language Translation (VLT) is a challenging task that requires accurately recognizing multilingual text embedded in images and translating it into the target language with the support of visual context. While recent Large Vision-Language Models (LVLMs) have demonstrated strong multilingual and visual understanding capabilities, there is a lack of systematic evaluation and understanding of their performance on VLT. In this work, we present a comprehensive study of VLT from three key perspectives: data quality, model architecture, and evaluation metrics. (1) We identify critical limitations in existing datasets, particularly in semantic and cultural fidelity, and introduce AibTrans -- a multilingual, parallel, human-verified dataset with OCR-corrected annotations. (2) We benchmark 11 commercial LVLMs/LLMs and 6 state-of-the-art open-source models across end-to-end and cascaded architectures, revealing their OCR dependency and contrasting generation versus reasoning behaviors. (3) We propose Density-Aware Evaluation to address metric reliability issues under varying contextual complexity, introducing the DA Score as a more robust measure of translation quality. Building upon these findings, we establish a new evaluation benchmark for VLT. Notably, we observe that fine-tuning LVLMs on high-resource language pairs degrades cross-lingual performance, and we propose a balanced multilingual fine-tuning strategy that effectively adapts LVLMs to VLT without sacrificing their generalization ability.
Via
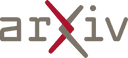
Jun 08, 2025
Abstract:Segmentation of nuclei regions from histological images enables morphometric analysis of nuclei structures, which in turn helps in the detection and diagnosis of diseases under consideration. To develop a nuclei segmentation algorithm, applicable to different types of target domain representations, image-to-image translation networks can be considered as they are invariant to target domain image representations. One of the important issues with image-to-image translation models is that they fail miserably when the information content between two image domains are asymmetric in nature. In this regard, the paper introduces a new deep generative model for segmenting nuclei structures from histological images. The proposed model considers an embedding space for handling information-disparity between information-rich histological image space and information-poor segmentation map domain. Integrating judiciously the concepts of optimal transport and measure theory, the model develops an invertible generator, which provides an efficient optimization framework with lower network complexity. The concept of invertible generator automatically eliminates the need of any explicit cycle-consistency loss. The proposed model also introduces a spatially-constrained squeeze operation within the framework of invertible generator to maintain spatial continuity within the image patches. The model provides a better trade-off between network complexity and model performance compared to other existing models having complex network architectures. The performance of the proposed deep generative model, along with a comparison with state-of-the-art nuclei segmentation methods, is demonstrated on publicly available histological image data sets.
* 13 pages, 8 figures
Via
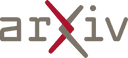
Jun 15, 2025
Abstract:Generative models based on deep learning have shown significant potential in medical imaging, particularly for modality transformation and multimodal fusion in MRI-based brain imaging. This study introduces GM-LDM, a novel framework that leverages the latent diffusion model (LDM) to enhance the efficiency and precision of MRI generation tasks. GM-LDM integrates a 3D autoencoder, pre-trained on the large-scale ABCD MRI dataset, achieving statistical consistency through KL divergence loss. We employ a Vision Transformer (ViT)-based encoder-decoder as the denoising network to optimize generation quality. The framework flexibly incorporates conditional data, such as functional network connectivity (FNC) data, enabling personalized brain imaging, biomarker identification, and functional-to-structural information translation for brain diseases like schizophrenia.
Via
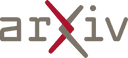