What is Image Enhancement? Image enhancement is basically improving the interpretability or perception of information in images for human viewers and providing ‘better’ input for other automated image processing techniques. The principal objective of Image Enhancement is to modify attributes of an image to make it more suitable for a given task and a specific observer.
Papers and Code
Apr 21, 2025
Abstract:Cross-Domain Sequential Recommendation (CDSR) predicts user behavior by leveraging historical interactions across multiple domains, focusing on modeling cross-domain preferences through intra- and inter-sequence item relationships. Inspired by human cognitive processes, we propose Hierarchical Attention Fusion of Visual and Textual Representations (HAF-VT), a novel approach integrating visual and textual data to enhance cognitive modeling. Using the frozen CLIP model, we generate image and text embeddings, enriching item representations with multimodal data. A hierarchical attention mechanism jointly learns single-domain and cross-domain preferences, mimicking human information integration. Evaluated on four e-commerce datasets, HAF-VT outperforms existing methods in capturing cross-domain user interests, bridging cognitive principles with computational models and highlighting the role of multimodal data in sequential decision-making.
* Accepted at CogSCI 2025
Via
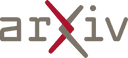
Apr 22, 2025
Abstract:Existing machine unlearning (MU) approaches exhibit significant sensitivity to hyperparameters, requiring meticulous tuning that limits practical deployment. In this work, we first empirically demonstrate the instability and suboptimal performance of existing popular MU methods when deployed in different scenarios. To address this issue, we propose Dual Optimizer (DualOptim), which incorporates adaptive learning rate and decoupled momentum factors. Empirical and theoretical evidence demonstrates that DualOptim contributes to effective and stable unlearning. Through extensive experiments, we show that DualOptim can significantly boost MU efficacy and stability across diverse tasks, including image classification, image generation, and large language models, making it a versatile approach to empower existing MU algorithms.
Via
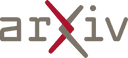
Apr 21, 2025
Abstract:In this work, we build the first benchmark dataset for short-form UGC Image Super-resolution in the wild, termed KwaiSR, intending to advance the research on developing image super-resolution algorithms for short-form UGC platforms. This dataset is collected from the Kwai Platform, which is composed of two parts, i.e., synthetic and wild parts. Among them, the synthetic dataset, including 1,900 image pairs, is produced by simulating the degradation following the distribution of real-world low-quality short-form UGC images, aiming to provide the ground truth for training and objective comparison in the validation/testing. The wild dataset contains low-quality images collected directly from the Kwai Platform, which are filtered using the quality assessment method KVQ from the Kwai Platform. As a result, the KwaiSR dataset contains 1800 synthetic image pairs and 1900 wild images, which are divided into training, validation, and testing parts with a ratio of 8:1:1. Based on the KwaiSR dataset, we organize the NTIRE 2025 challenge on a second short-form UGC Video quality assessment and enhancement, which attracts lots of researchers to develop the algorithm for it. The results of this competition have revealed that our KwaiSR dataset is pretty challenging for existing Image SR methods, which is expected to lead to a new direction in the image super-resolution field. The dataset can be found from https://lixinustc.github.io/NTIRE2025-KVQE-KwaSR-KVQ.github.io/.
* KwaiSR dataset, a new dataset for image super-resolution, used for
CVPR NTIRE 2025 Challenge; CVPR 2025 workshop paper
Via
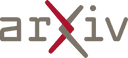
Apr 22, 2025
Abstract:In medical imaging, anomaly detection is a vital element of healthcare diagnostics, especially for neurological conditions which can be life-threatening. Conventional deterministic methods often fall short when it comes to capturing the inherent uncertainty of anomaly detection tasks. This paper introduces a Bayesian Variational Autoencoder (VAE) equipped with multi-head attention mechanisms for detecting anomalies in brain magnetic resonance imaging (MRI). For the purpose of improving anomaly detection performance, we incorporate both epistemic and aleatoric uncertainty estimation through Bayesian inference. The model was tested on the BraTS2020 dataset, and the findings were a 0.83 ROC AUC and a 0.83 PR AUC. The data in our paper suggests that modeling uncertainty is an essential component of anomaly detection, enhancing both performance and interpretability and providing confidence estimates, as well as anomaly predictions, for clinicians to leverage in making medical decisions.
* 16 pages, 6 figures
Via
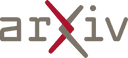
Apr 21, 2025
Abstract:We present a novel framework for generating high-quality, animatable 4D avatar from a single image. While recent advances have shown promising results in 4D avatar creation, existing methods either require extensive multiview data or struggle with shape accuracy and identity consistency. To address these limitations, we propose a comprehensive system that leverages shape, image, and video priors to create full-view, animatable avatars. Our approach first obtains initial coarse shape through 3D-GAN inversion. Then, it enhances multiview textures using depth-guided warping signals for cross-view consistency with the help of the image diffusion model. To handle expression animation, we incorporate a video prior with synchronized driving signals across viewpoints. We further introduce a Consistent-Inconsistent training to effectively handle data inconsistencies during 4D reconstruction. Experimental results demonstrate that our method achieves superior quality compared to the prior art, while maintaining consistency across different viewpoints and expressions.
Via
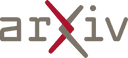
Apr 21, 2025
Abstract:Modern text-to-image generation systems have enabled the creation of remarkably realistic and high-quality visuals, yet they often falter when handling the inherent ambiguities in user prompts. In this work, we present Twin-Co, a framework that leverages synchronized, co-adaptive dialogue to progressively refine image generation. Instead of a static generation process, Twin-Co employs a dynamic, iterative workflow where an intelligent dialogue agent continuously interacts with the user. Initially, a base image is generated from the user's prompt. Then, through a series of synchronized dialogue exchanges, the system adapts and optimizes the image according to evolving user feedback. The co-adaptive process allows the system to progressively narrow down ambiguities and better align with user intent. Experiments demonstrate that Twin-Co not only enhances user experience by reducing trial-and-error iterations but also improves the quality of the generated images, streamlining the creative process across various applications.
Via
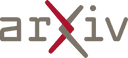
Apr 22, 2025
Abstract:The continuous improvement in weather forecast skill over the past several decades is largely due to the increasing quantity of available satellite observations and their assimilation into operational forecast systems. Assimilating these observations requires observation operators in the form of radiative transfer models. Significant efforts have been dedicated to enhancing the computational efficiency of these models. Computational cost remains a bottleneck, and a large fraction of available data goes unused for assimilation. To address this, we used machine learning to build an efficient neural network based probabilistic emulator of the Community Radiative Transfer Model (CRTM), applied to the GOES Advanced Baseline Imager. The trained NN emulator predicts brightness temperatures output by CRTM and the corresponding error with respect to CRTM. RMSE of the predicted brightness temperature is 0.3 K averaged across all channels. For clear sky conditions, the RMSE is less than 0.1 K for 9 out of 10 infrared channels. The error predictions are generally reliable across a wide range of conditions. Explainable AI methods demonstrate that the trained emulator reproduces the relevant physics, increasing confidence that the model will perform well when presented with new data.
* 26 pages, 9 figures, 1 table
Via
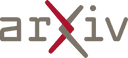
Apr 21, 2025
Abstract:Entity linking (EL) aligns textual mentions with their corresponding entities in a knowledge base, facilitating various applications such as semantic search and question answering. Recent advances in multimodal entity linking (MEL) have shown that combining text and images can reduce ambiguity and improve alignment accuracy. However, most existing MEL methods overlook the rich structural information available in the form of knowledge-graph (KG) triples. In this paper, we propose KGMEL, a novel framework that leverages KG triples to enhance MEL. Specifically, it operates in three stages: (1) Generation: Produces high-quality triples for each mention by employing vision-language models based on its text and images. (2) Retrieval: Learns joint mention-entity representations, via contrastive learning, that integrate text, images, and (generated or KG) triples to retrieve candidate entities for each mention. (3) Reranking: Refines the KG triples of the candidate entities and employs large language models to identify the best-matching entity for the mention. Extensive experiments on benchmark datasets demonstrate that KGMEL outperforms existing methods. Our code and datasets are available at: https://github.com/juyeonnn/KGMEL.
* SIGIR 2025 (Short)
Via
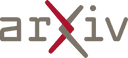
Apr 22, 2025
Abstract:Multimodal aspect-based sentiment classification (MASC) is an emerging task due to an increase in user-generated multimodal content on social platforms, aimed at predicting sentiment polarity toward specific aspect targets (i.e., entities or attributes explicitly mentioned in text-image pairs). Despite extensive efforts and significant achievements in existing MASC, substantial gaps remain in understanding fine-grained visual content and the cognitive rationales derived from semantic content and impressions (cognitive interpretations of emotions evoked by image content). In this study, we present Chimera: a cognitive and aesthetic sentiment causality understanding framework to derive fine-grained holistic features of aspects and infer the fundamental drivers of sentiment expression from both semantic perspectives and affective-cognitive resonance (the synergistic effect between emotional responses and cognitive interpretations). Specifically, this framework first incorporates visual patch features for patch-word alignment. Meanwhile, it extracts coarse-grained visual features (e.g., overall image representation) and fine-grained visual regions (e.g., aspect-related regions) and translates them into corresponding textual descriptions (e.g., facial, aesthetic). Finally, we leverage the sentimental causes and impressions generated by a large language model (LLM) to enhance the model's awareness of sentimental cues evoked by semantic content and affective-cognitive resonance. Experimental results on standard MASC datasets demonstrate the effectiveness of the proposed model, which also exhibits greater flexibility to MASC compared to LLMs such as GPT-4o. We have publicly released the complete implementation and dataset at https://github.com/Xillv/Chimera
* Accepted by TAFFC 2025
Via
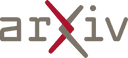
Apr 22, 2025
Abstract:Domain-adaptive thermal object detection plays a key role in facilitating visible (RGB)-to-thermal (IR) adaptation by reducing the need for co-registered image pairs and minimizing reliance on large annotated IR datasets. However, inherent limitations of IR images, such as the lack of color and texture cues, pose challenges for RGB-trained models, leading to increased false positives and poor-quality pseudo-labels. To address this, we propose Semantic-Aware Gray color Augmentation (SAGA), a novel strategy for mitigating color bias and bridging the domain gap by extracting object-level features relevant to IR images. Additionally, to validate the proposed SAGA for drone imagery, we introduce the IndraEye, a multi-sensor (RGB-IR) dataset designed for diverse applications. The dataset contains 5,612 images with 145,666 instances, captured from diverse angles, altitudes, backgrounds, and times of day, offering valuable opportunities for multimodal learning, domain adaptation for object detection and segmentation, and exploration of sensor-specific strengths and weaknesses. IndraEye aims to enhance the development of more robust and accurate aerial perception systems, especially in challenging environments. Experimental results show that SAGA significantly improves RGB-to-IR adaptation for autonomous driving and IndraEye dataset, achieving consistent performance gains of +0.4% to +7.6% (mAP) when integrated with state-of-the-art domain adaptation techniques. The dataset and codes are available at https://github.com/airliisc/IndraEye.
* Accepted at CVPR-W PBVS 2025
Via
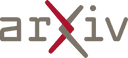