Topic:Few Shot Object Detection
What is Few Shot Object Detection? Few-shot object detection is a computer-vision task that involves detecting objects in images with limited training data. The goal is to train a model on a few examples of each object class and then use the model to detect objects in new images.
Papers and Code
Jun 17, 2025
Abstract:In this paper, we construct two research objectives: i) explore the learned embedding space of BiomedCLIP, an open-source large vision language model, to analyse meaningful class separations, and ii) quantify the limitations of BiomedCLIP when applied to a highly imbalanced, out-of-distribution multi-label medical dataset. We experiment on IU-xray dataset, which exhibits the aforementioned criteria, and evaluate BiomedCLIP in classifying images (radiographs) in three contexts: zero-shot inference, full finetuning, and linear probing. The results show that the model under zero-shot settings over-predicts all labels, leading to poor precision and inter-class separability. Full fine-tuning improves classification of distinct diseases, while linear probing detects overlapping features. We demonstrate visual understanding of the model using Grad-CAM heatmaps and compare with 15 annotations by a radiologist. We highlight the need for careful adaptations of the models to foster reliability and applicability in a real-world setting. The code for the experiments in this work is available and maintained on GitHub.
Via
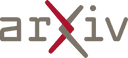
Jun 06, 2025
Abstract:Cross-Domain Few-Shot Object Detection (CD-FSOD) aims to detect novel objects with only a handful of labeled samples from previously unseen domains. While data augmentation and generative methods have shown promise in few-shot learning, their effectiveness for CD-FSOD remains unclear due to the need for both visual realism and domain alignment. Existing strategies, such as copy-paste augmentation and text-to-image generation, often fail to preserve the correct object category or produce backgrounds coherent with the target domain, making them non-trivial to apply directly to CD-FSOD. To address these challenges, we propose Domain-RAG, a training-free, retrieval-guided compositional image generation framework tailored for CD-FSOD. Domain-RAG consists of three stages: domain-aware background retrieval, domain-guided background generation, and foreground-background composition. Specifically, the input image is first decomposed into foreground and background regions. We then retrieve semantically and stylistically similar images to guide a generative model in synthesizing a new background, conditioned on both the original and retrieved contexts. Finally, the preserved foreground is composed with the newly generated domain-aligned background to form the generated image. Without requiring any additional supervision or training, Domain-RAG produces high-quality, domain-consistent samples across diverse tasks, including CD-FSOD, remote sensing FSOD, and camouflaged FSOD. Extensive experiments show consistent improvements over strong baselines and establish new state-of-the-art results. Codes will be released upon acceptance.
Via
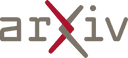
Jun 11, 2025
Abstract:Out-of-context and misattributed imagery is the leading form of media manipulation in today's misinformation and disinformation landscape. The existing methods attempting to detect this practice often only consider whether the semantics of the imagery corresponds to the text narrative, missing manipulation so long as the depicted objects or scenes somewhat correspond to the narrative at hand. To tackle this, we introduce News Media Provenance Dataset, a dataset of news articles with provenance-tagged images. We formulate two tasks on this dataset, location of origin relevance (LOR) and date and time of origin relevance (DTOR), and present baseline results on six large language models (LLMs). We identify that, while the zero-shot performance on LOR is promising, the performance on DTOR hinders, leaving room for specialized architectures and future work.
* Workshop on NLP for Positive Impact @ ACL 2025
Via
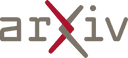
May 26, 2025
Abstract:Recent methods for zero-shot Human-Object Interaction (HOI) detection typically leverage the generalization ability of large Vision-Language Model (VLM), i.e., CLIP, on unseen categories, showing impressive results on various zero-shot settings. However, existing methods struggle to adapt CLIP representations for human-object pairs, as CLIP tends to overlook fine-grained information necessary for distinguishing interactions. To address this issue, we devise, LAIN, a novel zero-shot HOI detection framework enhancing the locality and interaction awareness of CLIP representations. The locality awareness, which involves capturing fine-grained details and the spatial structure of individual objects, is achieved by aggregating the information and spatial priors of adjacent neighborhood patches. The interaction awareness, which involves identifying whether and how a human is interacting with an object, is achieved by capturing the interaction pattern between the human and the object. By infusing locality and interaction awareness into CLIP representation, LAIN captures detailed information about the human-object pairs. Our extensive experiments on existing benchmarks show that LAIN outperforms previous methods on various zero-shot settings, demonstrating the importance of locality and interaction awareness for effective zero-shot HOI detection.
* Accepted to CVPR2025; Code is available at:
https://github.com/OreoChocolate/LAIN
Via
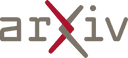
Jun 06, 2025
Abstract:Understanding relationships between objects is central to visual intelligence, with applications in embodied AI, assistive systems, and scene understanding. Yet, most visual relationship detection (VRD) models rely on a fixed predicate set, limiting their generalization to novel interactions. A key challenge is the inability to visually ground semantically plausible, but unannotated, relationships hypothesized from external knowledge. This work introduces an iterative visual grounding framework that leverages large language models (LLMs) as structured relational priors. Inspired by expectation-maximization (EM), our method alternates between generating candidate scene graphs from detected objects using an LLM (expectation) and training a visual model to align these hypotheses with perceptual evidence (maximization). This process bootstraps relational understanding beyond annotated data and enables generalization to unseen predicates. Additionally, we introduce a new benchmark for open-world VRD on Visual Genome with 21 held-out predicates and evaluate under three settings: seen, unseen, and mixed. Our model outperforms LLM-only, few-shot, and debiased baselines, achieving mean recall (mR@50) of 15.9, 13.1, and 11.7 on predicate classification on these three sets. These results highlight the promise of grounded LLM priors for scalable open-world visual understanding.
* 22 pages, 9 figures, 5 tables
Via
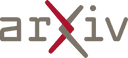
May 20, 2025
Abstract:This paper focus on few-shot object detection~(FSOD) and instance segmentation~(FSIS), which requires a model to quickly adapt to novel classes with a few labeled instances. The existing methods severely suffer from bias classification because of the missing label issue which naturally exists in an instance-level few-shot scenario and is first formally proposed by us. Our analysis suggests that the standard classification head of most FSOD or FSIS models needs to be decoupled to mitigate the bias classification. Therefore, we propose an embarrassingly simple but effective method that decouples the standard classifier into two heads. Then, these two individual heads are capable of independently addressing clear positive samples and noisy negative samples which are caused by the missing label. In this way, the model can effectively learn novel classes while mitigating the effects of noisy negative samples. Without bells and whistles, our model without any additional computation cost and parameters consistently outperforms its baseline and state-of-the-art by a large margin on PASCAL VOC and MS-COCO benchmarks for FSOD and FSIS tasks. The Code is available at https://csgaobb.github.io/Projects/DCFS.
* Accepted by NeurIPS 2022
Via
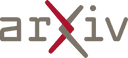
May 27, 2025
Abstract:Vision-language models (VLMs) trained on internet-scale data achieve remarkable zero-shot detection performance on common objects like car, truck, and pedestrian. However, state-of-the-art models still struggle to generalize to out-of-distribution classes, tasks and imaging modalities not typically found in their pre-training. Rather than simply re-training VLMs on more visual data, we argue that one should align VLMs to new concepts with annotation instructions containing a few visual examples and rich textual descriptions. To this end, we introduce Roboflow100-VL, a large-scale collection of 100 multi-modal object detection datasets with diverse concepts not commonly found in VLM pre-training. We evaluate state-of-the-art models on our benchmark in zero-shot, few-shot, semi-supervised, and fully-supervised settings, allowing for comparison across data regimes. Notably, we find that VLMs like GroundingDINO and Qwen2.5-VL achieve less than 2% zero-shot accuracy on challenging medical imaging datasets within Roboflow100-VL, demonstrating the need for few-shot concept alignment. Our code and dataset are available at https://github.com/roboflow/rf100-vl/ and https://universe.roboflow.com/rf100-vl/
* The first two authors contributed equally
Via
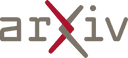
Jun 05, 2025
Abstract:Integrated sensing and communication (ISAC) has been envisioned to play a more important role in future wireless networks. However, the design of ISAC networks is challenging, especially when there are multiple communication and sensing (C\&S) nodes and multiple sensing targets. We investigate a multi-base station (BS) ISAC network in which multiple BSs equipped with multiple antennas simultaneously provide C\&S services for multiple ground communication users (CUs) and targets. To enhance the overall performance of C\&S, we formulate a joint user association (UA) and multi-BS transmit beamforming optimization problem with the objective of maximizing the total sum rate of all CUs while ensuring both the minimum target detection and parameter estimation requirements. To efficiently solve the highly non-convex mixed integer nonlinear programming (MINLP) optimization problem, we propose an alternating optimization (AO)-based algorithm that decomposes the problem into two sub-problems, i.e., UA optimization and multi-BS transmit beamforming optimization. Inspired by large language models (LLMs) for prediction and inference, we propose a unified framework integrating LLMs with convex-based optimization methods. First, we propose a comprehensive design of prompt engineering, including few-shot, chain of thought, and self-reflection techniques to guide LLMs in solving the binary integer programming UA optimization problem. Second, we utilize convex-based optimization methods to handle the non-convex beamforming optimization problem based on fractional programming (FP), majorization minimization (MM), and the alternating direction method of multipliers (ADMM) with an optimized UA from LLMs. Numerical results demonstrate that our proposed LLM-enabled AO-based algorithm achieves fast convergence and near upper-bound performance with the GPT-o1 model, outperforming various benchmark schemes.
Via
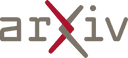
May 30, 2025
Abstract:We present a simple yet effective training-free approach for zero-shot 3D symmetry detection that leverages visual features from foundation vision models such as DINOv2. Our method extracts features from rendered views of 3D objects and backprojects them onto the original geometry. We demonstrate the symmetric invariance of these features and use them to identify reflection-symmetry planes through a proposed algorithm. Experiments on a subset of ShapeNet demonstrate that our approach outperforms both traditional geometric methods and learning-based approaches without requiring any training data. Our work demonstrates how foundation vision models can help in solving complex 3D geometric problems such as symmetry detection.
Via
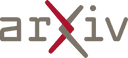
May 26, 2025
Abstract:The global challenge of sustainable recycling demands automated, fast, and accurate, state-of-the-art (SOTA) material detection systems that act as a bedrock for a circular economy. Democratizing access to these cutting-edge solutions that enable real-time waste analysis is essential for scaling up recycling efforts and fostering the Green Deal. In response, we introduce \textbf{Electrolyzers-HSI}, a novel multimodal benchmark dataset designed to accelerate the recovery of critical raw materials through accurate electrolyzer materials classification. The dataset comprises 55 co-registered high-resolution RGB images and hyperspectral imaging (HSI) data cubes spanning the 400--2500 nm spectral range, yielding over 4.2 million pixel vectors and 424,169 labeled ones. This enables non-invasive spectral analysis of shredded electrolyzer samples, supporting quantitative and qualitative material classification and spectral properties investigation. We evaluate a suite of baseline machine learning (ML) methods alongside SOTA transformer-based deep learning (DL) architectures, including Vision Transformer, SpectralFormer, and the Multimodal Fusion Transformer, to investigate architectural bottlenecks for further efficiency optimisation when deploying transformers in material identification. We implement zero-shot detection techniques and majority voting across pixel-level predictions to establish object-level classification robustness. In adherence to the FAIR data principles, the electrolyzers-HSI dataset and accompanying codebase are openly available at https://github.com/hifexplo/Electrolyzers-HSI and https://rodare.hzdr.de/record/3668, supporting reproducible research and facilitating the broader adoption of smart and sustainable e-waste recycling solutions.
Via
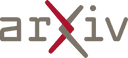