Apr 17, 2025
Abstract:Computer vision is transforming fashion through Virtual Try-On (VTON) and Virtual Try-Off (VTOFF). VTON generates images of a person in a specified garment using a target photo and a standardized garment image, while a more challenging variant, Person-to-Person Virtual Try-On (p2p-VTON), uses a photo of another person wearing the garment. VTOFF, on the other hand, extracts standardized garment images from clothed individuals. We introduce TryOffDiff, a diffusion-based VTOFF model. Built on a latent diffusion framework with SigLIP image conditioning, it effectively captures garment properties like texture, shape, and patterns. TryOffDiff achieves state-of-the-art results on VITON-HD and strong performance on DressCode dataset, covering upper-body, lower-body, and dresses. Enhanced with class-specific embeddings, it pioneers multi-garment VTOFF, the first of its kind. When paired with VTON models, it improves p2p-VTON by minimizing unwanted attribute transfer, such as skin color. Code is available at: https://rizavelioglu.github.io/tryoffdiff/
Via
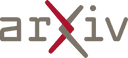