What is music generation? Music generation is the task of generating music or music-like sounds from a model or algorithm.
Papers and Code
Feb 11, 2025
Abstract:Expressive music performance rendering involves interpreting symbolic scores with variations in timing, dynamics, articulation, and instrument-specific techniques, resulting in performances that capture musical can emotional intent. We introduce RenderBox, a unified framework for text-and-score controlled audio performance generation across multiple instruments, applying coarse-level controls through natural language descriptions and granular-level controls using music scores. Based on a diffusion transformer architecture and cross-attention joint conditioning, we propose a curriculum-based paradigm that trains from plain synthesis to expressive performance, gradually incorporating controllable factors such as speed, mistakes, and style diversity. RenderBox achieves high performance compared to baseline models across key metrics such as FAD and CLAP, and also tempo and pitch accuracy under different prompting tasks. Subjective evaluation further demonstrates that RenderBox is able to generate controllable expressive performances that sound natural and musically engaging, aligning well with prompts and intent.
Via
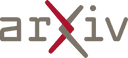
Jan 30, 2025
Abstract:Image animation has become a promising area in multimodal research, with a focus on generating videos from reference images. While prior work has largely emphasized generic video generation guided by text, music-driven dance video generation remains underexplored. In this paper, we introduce MuseDance, an innovative end-to-end model that animates reference images using both music and text inputs. This dual input enables MuseDance to generate personalized videos that follow text descriptions and synchronize character movements with the music. Unlike existing approaches, MuseDance eliminates the need for complex motion guidance inputs, such as pose or depth sequences, making flexible and creative video generation accessible to users of all expertise levels. To advance research in this field, we present a new multimodal dataset comprising 2,904 dance videos with corresponding background music and text descriptions. Our approach leverages diffusion-based methods to achieve robust generalization, precise control, and temporal consistency, setting a new baseline for the music-driven image animation task.
Via
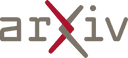
Feb 18, 2025
Abstract:While neural vocoders have made significant progress in high-fidelity speech synthesis, their application on polyphonic music has remained underexplored. In this work, we propose DisCoder, a neural vocoder that leverages a generative adversarial encoder-decoder architecture informed by a neural audio codec to reconstruct high-fidelity 44.1 kHz audio from mel spectrograms. Our approach first transforms the mel spectrogram into a lower-dimensional representation aligned with the Descript Audio Codec (DAC) latent space before reconstructing it to an audio signal using a fine-tuned DAC decoder. DisCoder achieves state-of-the-art performance in music synthesis on several objective metrics and in a MUSHRA listening study. Our approach also shows competitive performance in speech synthesis, highlighting its potential as a universal vocoder.
* Accepted at ICASSP 2025
Via
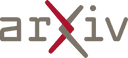
Feb 18, 2025
Abstract:Text-to-song generation, the task of creating vocals and accompaniment from textual inputs, poses significant challenges due to domain complexity and data scarcity. Existing approaches often employ multi-stage generation procedures, resulting in cumbersome training and inference pipelines. In this paper, we propose SongGen, a fully open-source, single-stage auto-regressive transformer designed for controllable song generation. The proposed model facilitates fine-grained control over diverse musical attributes, including lyrics and textual descriptions of instrumentation, genre, mood, and timbre, while also offering an optional three-second reference clip for voice cloning. Within a unified auto-regressive framework, SongGen supports two output modes: mixed mode, which generates a mixture of vocals and accompaniment directly, and dual-track mode, which synthesizes them separately for greater flexibility in downstream applications. We explore diverse token pattern strategies for each mode, leading to notable improvements and valuable insights. Furthermore, we design an automated data preprocessing pipeline with effective quality control. To foster community engagement and future research, we will release our model weights, training code, annotated data, and preprocessing pipeline. The generated samples are showcased on our project page at https://liuzh-19.github.io/SongGen/ , and the code will be available at https://github.com/LiuZH-19/SongGen .
Via
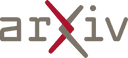
Dec 31, 2024
Abstract:Music generation has been established as a prominent topic in artificial intelligence and machine learning over recent years. In most recent works on RNN-based neural network methods have been applied for sequence generation. In contrast, generative adversarial networks (GANs) and their counterparts have been explored by very few researchersfor music generation. In this paper, a classical system was employed alongside a new system to generate creative music. Both systems were designed based on adversarial networks to generate music by learning from examples. The classical system was trained to learn a set of music pieces without differentiating between classes, whereas the new system was trained to learn the different composers and their styles to generate a creative music piece by deviating from the learned composers' styles. The base structure utilized was generative adversarial networks (GANs), which are capable of generating novel outputs given a set of inputs to learn from and mimic their distribution. It has been shown in previous work that GANs are limited in their original design with respect to creative outputs. Building on the Creative Adversarial Networks (CAN) , this work applied them in the music domain rather than the visual art domain. Additionally, unrolled CAN was introduced to prevent mode collapse. Experiments were conducted on both GAN and CAN for generating music, and their capabilities were measured in terms of deviation from the input set.
Via
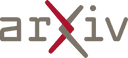
Jan 26, 2025
Abstract:Amphion is an open-source toolkit for Audio, Music, and Speech Generation, designed to lower the entry barrier for junior researchers and engineers in these fields. It provides a versatile framework that supports a variety of generation tasks and models. In this report, we introduce Amphion v0.2, the second major release developed in 2024. This release features a 100K-hour open-source multilingual dataset, a robust data preparation pipeline, and novel models for tasks such as text-to-speech, audio coding, and voice conversion. Furthermore, the report includes multiple tutorials that guide users through the functionalities and usage of the newly released models.
Via
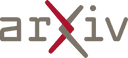
Jan 10, 2025
Abstract:The streaming service Deezer heavily relies on the search to help users navigate through its extensive music catalog. Nonetheless, it is primarily designed to find specific items and does not lead directly to a smooth listening experience. We present Text2Playlist, a stand-alone tool that addresses these limitations. Text2Playlist leverages generative AI, music information retrieval and recommendation systems to generate query-specific and personalized playlists, successfully deployed at scale.
Via
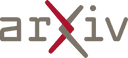
Jan 29, 2025
Abstract:Efficiently compressing high-dimensional audio signals into a compact and informative latent space is crucial for various tasks, including generative modeling and music information retrieval (MIR). Existing audio autoencoders, however, often struggle to achieve high compression ratios while preserving audio fidelity and facilitating efficient downstream applications. We introduce Music2Latent2, a novel audio autoencoder that addresses these limitations by leveraging consistency models and a novel approach to representation learning based on unordered latent embeddings, which we call summary embeddings. Unlike conventional methods that encode local audio features into ordered sequences, Music2Latent2 compresses audio signals into sets of summary embeddings, where each embedding can capture distinct global features of the input sample. This enables to achieve higher reconstruction quality at the same compression ratio. To handle arbitrary audio lengths, Music2Latent2 employs an autoregressive consistency model trained on two consecutive audio chunks with causal masking, ensuring coherent reconstruction across segment boundaries. Additionally, we propose a novel two-step decoding procedure that leverages the denoising capabilities of consistency models to further refine the generated audio at no additional cost. Our experiments demonstrate that Music2Latent2 outperforms existing continuous audio autoencoders regarding audio quality and performance on downstream tasks. Music2Latent2 paves the way for new possibilities in audio compression.
* Accepted to ICASSP 2025
Via
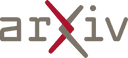
Jan 05, 2025
Abstract:In recent years, there has been a notable increase in research on machine learning models for music retrieval and generation systems that are capable of taking natural language sentences as inputs. However, there is a scarcity of large-scale publicly available datasets, consisting of music data and their corresponding natural language descriptions known as music captions. In particular, non-musical information such as suitable situations for listening to a track and the emotions elicited upon listening is crucial for describing music. This type of information is underrepresented in existing music caption datasets due to the challenges associated with extracting it directly from music data. To address this issue, we propose a method for generating music caption data that incorporates non-musical aspects inferred from music thumbnail images, and validated the effectiveness of our approach through human evaluations. Additionally, we created a dataset with approximately 360,000 captions containing non-musical aspects. Leveraging this dataset, we trained a music retrieval model and demonstrated its effectiveness in music retrieval tasks through evaluation.
* Accepted at NLP4MusA 2024
Via
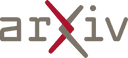
Dec 12, 2024
Abstract:Multimodal music generation aims to produce music from diverse input modalities, including text, videos, and images. Existing methods use a common embedding space for multimodal fusion. Despite their effectiveness in other modalities, their application in multimodal music generation faces challenges of data scarcity, weak cross-modal alignment, and limited controllability. This paper addresses these issues by using explicit bridges of text and music for multimodal alignment. We introduce a novel method named Visuals Music Bridge (VMB). Specifically, a Multimodal Music Description Model converts visual inputs into detailed textual descriptions to provide the text bridge; a Dual-track Music Retrieval module that combines broad and targeted retrieval strategies to provide the music bridge and enable user control. Finally, we design an Explicitly Conditioned Music Generation framework to generate music based on the two bridges. We conduct experiments on video-to-music, image-to-music, text-to-music, and controllable music generation tasks, along with experiments on controllability. The results demonstrate that VMB significantly enhances music quality, modality, and customization alignment compared to previous methods. VMB sets a new standard for interpretable and expressive multimodal music generation with applications in various multimedia fields. Demos and code are available at https://github.com/wbs2788/VMB.
Via
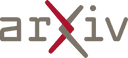