Jun 26, 2025
Abstract:Grokking, i.e., test performance keeps improving long after training loss converged, has been recently witnessed in neural network training, making the mechanism of generalization and other emerging capabilities such as reasoning mysterious. While prior studies usually train small models on a few toy or highly-specific tasks for thousands of epochs, we conduct the first study of grokking on checkpoints during one-pass pretraining of a 7B large language model (LLM), i.e., OLMoE. We compute the training loss and evaluate generalization on diverse benchmark tasks, including math reasoning, code generation, and commonsense/domain-specific knowledge retrieval tasks. Our study, for the first time, verifies that grokking still happens in the pretraining of large-scale foundation models, though different data may enter grokking stages asynchronously. We further demystify grokking's "emergence of generalization" by investigating LLM internal dynamics. Specifically, we find that training samples' pathways (i.e., expert choices across layers) evolve from random, instance-specific to more structured and shareable between samples during grokking. Also, the complexity of a sample's pathway reduces despite the converged loss. These indicate a memorization-to-generalization conversion, providing a mechanistic explanation of delayed generalization. In the study, we develop two novel metrics to quantify pathway distance and the complexity of a single pathway. We show their ability to predict the generalization improvement on diverse downstream tasks. They are efficient, simple to compute and solely dependent on training data. Hence, they have practical value for pretraining, enabling us to monitor the generalization performance without finetuning and test. Theoretically, we show that more structured pathways reduce model complexity and improve the generalization bound.
Via
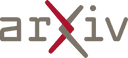