Nov 04, 2024
Abstract:Localization is one of the most crucial tasks for Unmanned Aerial Vehicle systems (UAVs) directly impacting overall performance, which can be achieved with various sensors and applied to numerous tasks related to search and rescue operations, object tracking, construction, etc. However, due to the negative effects of challenging environments, UAVs may lose signals for localization. In this paper, we present an effective path-planning system leveraging semantic segmentation information to navigate around texture-less and problematic areas like lakes, oceans, and high-rise buildings using a monocular camera. We introduce a real-time semantic segmentation architecture and a novel keyframe decision pipeline to optimize image inputs based on pixel distribution, reducing processing time. A hierarchical planner based on the Dynamic Window Approach (DWA) algorithm, integrated with a cost map, is designed to facilitate efficient path planning. The system is implemented in a photo-realistic simulation environment using Unity, aligning with segmentation model parameters. Comprehensive qualitative and quantitative evaluations validate the effectiveness of our approach, showing significant improvements in the reliability and efficiency of UAV localization in challenging environments.
* In The 24th International Conference on Control, Automation, and
Systems (ICCAS 2024), Jeju, Korea
Via
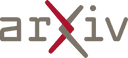