Dec 16, 2024
Abstract:Real-world design tasks - such as picture book creation, film storyboard development using character sets, photo retouching, visual effects, and font transfer - are highly diverse and complex, requiring deep interpretation and extraction of various elements from instructions, descriptions, and reference images. The resulting images often implicitly capture key features from references or user inputs, making it challenging to develop models that can effectively address such varied tasks. While existing visual generative models can produce high-quality images based on prompts, they face significant limitations in professional design scenarios that involve varied forms and multiple inputs and outputs, even when enhanced with adapters like ControlNets and LoRAs. To address this, we introduce IDEA-Bench, a comprehensive benchmark encompassing 100 real-world design tasks, including rendering, visual effects, storyboarding, picture books, fonts, style-based, and identity-preserving generation, with 275 test cases to thoroughly evaluate a model's general-purpose generation capabilities. Notably, even the best-performing model only achieves 22.48 on IDEA-Bench, while the best general-purpose model only achieves 6.81. We provide a detailed analysis of these results, highlighting the inherent challenges and providing actionable directions for improvement. Additionally, we provide a subset of 18 representative tasks equipped with multimodal large language model (MLLM)-based auto-evaluation techniques to facilitate rapid model development and comparison. We releases the benchmark data, evaluation toolkits, and an online leaderboard at https://github.com/ali-vilab/IDEA-Bench, aiming to drive the advancement of generative models toward more versatile and applicable intelligent design systems.
Via
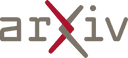