What is music generation? Music generation is the task of generating music or music-like sounds from a model or algorithm.
Papers and Code
Feb 12, 2025
Abstract:The advent of Music-Language Models has greatly enhanced the automatic music generation capability of AI systems, but they are also limited in their coverage of the musical genres and cultures of the world. We present a study of the datasets and research papers for music generation and quantify the bias and under-representation of genres. We find that only 5.7% of the total hours of existing music datasets come from non-Western genres, which naturally leads to disparate performance of the models across genres. We then investigate the efficacy of Parameter-Efficient Fine-Tuning (PEFT) techniques in mitigating this bias. Our experiments with two popular models -- MusicGen and Mustango, for two underrepresented non-Western music traditions -- Hindustani Classical and Turkish Makam music, highlight the promises as well as the non-triviality of cross-genre adaptation of music through small datasets, implying the need for more equitable baseline music-language models that are designed for cross-cultural transfer learning.
* 17 pages, 5 figures, accepted to NAACL'25
Via
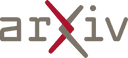
Mar 14, 2025
Abstract:Neural Audio Synthesis (NAS) models offer interactive musical control over high-quality, expressive audio generators. While these models can operate in real-time, they often suffer from high latency, making them unsuitable for intimate musical interaction. The impact of architectural choices in deep learning models on audio latency remains largely unexplored in the NAS literature. In this work, we investigate the sources of latency and jitter typically found in interactive NAS models. We then apply this analysis to the task of timbre transfer using RAVE, a convolutional variational autoencoder for audio waveforms introduced by Caillon et al. in 2021. Finally, we present an iterative design approach for optimizing latency. This culminates with a model we call BRAVE (Bravely Realtime Audio Variational autoEncoder), which is low-latency and exhibits better pitch and loudness replication while showing timbre modification capabilities similar to RAVE. We implement it in a specialized inference framework for low-latency, real-time inference and present a proof-of-concept audio plugin compatible with audio signals from musical instruments. We expect the challenges and guidelines described in this document to support NAS researchers in designing models for low-latency inference from the ground up, enriching the landscape of possibilities for musicians.
* See website at fcaspe.github.io/brave - 13 pages, 5 figures, accepted
to the Journal of the Audio Engineering Society
Via
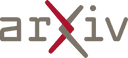
Mar 12, 2025
Abstract:Dance serves as a profound and universal expression of human culture, conveying emotions and stories through movements synchronized with music. Although some current works have achieved satisfactory results in the task of single-person dance generation, the field of multi-person dance generation remains relatively novel. In this work, we present a group choreography framework that leverages recent advancements in Large Language Models (LLM) by modeling the group dance generation problem as a sequence-to-sequence translation task. Our framework consists of a tokenizer that transforms continuous features into discrete tokens, and an LLM that is fine-tuned to predict motion tokens given the audio tokens. We show that by proper tokenization of input modalities and careful design of the LLM training strategies, our framework can generate realistic and diverse group dances while maintaining strong music correlation and dancer-wise consistency. Extensive experiments and evaluations demonstrate that our framework achieves state-of-the-art performance.
Via
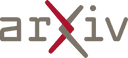
Feb 28, 2025
Abstract:Recent advances in generative artificial intelligence (AI) have created models capable of high-quality musical content generation. However, little consideration is given to how to use these models for real-time or cooperative jamming musical applications because of crucial required features: low latency, the ability to communicate planned actions, and the ability to adapt to user input in real-time. To support these needs, we introduce ReaLJam, an interface and protocol for live musical jamming sessions between a human and a Transformer-based AI agent trained with reinforcement learning. We enable real-time interactions using the concept of anticipation, where the agent continually predicts how the performance will unfold and visually conveys its plan to the user. We conduct a user study where experienced musicians jam in real-time with the agent through ReaLJam. Our results demonstrate that ReaLJam enables enjoyable and musically interesting sessions, and we uncover important takeaways for future work.
* Published in Extended Abstracts of the CHI Conference on Human
Factors in Computing Systems (CHI EA '25), April 26-May 1, 2025, Yokohama,
Japan
Via
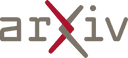
Mar 16, 2025
Abstract:Rhythm is a fundamental aspect of human behaviour, present from infancy and deeply embedded in cultural practices. Rhythm anticipation is a spontaneous cognitive process that typically occurs before the onset of actual beats. While most research in both neuroscience and artificial intelligence has focused on metronome-based rhythm tasks, studies investigating the perception of complex musical rhythm patterns remain limited. To address this gap, we propose a hierarchical oscillator-based model to better understand the perception of complex musical rhythms in biological systems. The model consists of two types of coupled neurons that generate oscillations, with different layers tuned to respond to distinct perception levels. We evaluate the model using several representative rhythm patterns spanning the upper, middle, and lower bounds of human musical perception. Our findings demonstrate that, while maintaining a high degree of synchronization accuracy, the model exhibits human-like rhythmic behaviours. Additionally, the beta band neuronal activity in the model mirrors patterns observed in the human brain, further validating the biological plausibility of the approach.
Via
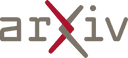
Feb 21, 2025
Abstract:Music composition has long been recognized as a significant art form. However, existing digital audio workstations and music production software often present high entry barriers for users lacking formal musical training. To address this, we introduce ComposeOn, a music theory-based tool designed for users with limited musical knowledge. ComposeOn enables users to easily extend their melodic ideas into complete compositions and offers simple editing features. By integrating music theory, it explains music creation at beginner, intermediate, and advanced levels. Our user study (N=10) compared ComposeOn with the baseline method, Suno AI, demonstrating that ComposeOn provides a more accessible and enjoyable composing and learning experience for individuals with limited musical skills. ComposeOn bridges the gap between theory and practice, offering an innovative solution as both a composition aid and music education platform. The study also explores the differences between theory-based music creation and generative music, highlighting the former's advantages in personal expression and learning.
Via
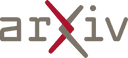
Mar 05, 2025
Abstract:As speech translation (ST) systems become increasingly prevalent, understanding their vulnerabilities is crucial for ensuring robust and reliable communication. However, limited work has explored this issue in depth. This paper explores methods of compromising these systems through imperceptible audio manipulations. Specifically, we present two innovative approaches: (1) the injection of perturbation into source audio, and (2) the generation of adversarial music designed to guide targeted translation, while also conducting more practical over-the-air attacks in the physical world. Our experiments reveal that carefully crafted audio perturbations can mislead translation models to produce targeted, harmful outputs, while adversarial music achieve this goal more covertly, exploiting the natural imperceptibility of music. These attacks prove effective across multiple languages and translation models, highlighting a systemic vulnerability in current ST architectures. The implications of this research extend beyond immediate security concerns, shedding light on the interpretability and robustness of neural speech processing systems. Our findings underscore the need for advanced defense mechanisms and more resilient architectures in the realm of audio systems. More details and samples can be found at https://adv-st.github.io.
* Preprint,17 pages, 17 figures
Via
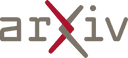
Feb 18, 2025
Abstract:Automatic music transcription converts audio recordings into symbolic representations, facilitating music analysis, retrieval, and generation. A musical note is characterized by pitch, onset, and offset in an audio domain, whereas it is defined in terms of pitch and note value in a musical score domain. A time-aligned score, derived from timing information along with pitch and note value, allows matching a part of the score with the corresponding part of the music audio, enabling various applications. In this paper, we consider an extended version of the traditional note-level transcription task that recognizes onset, offset, and pitch, through including extraction of additional note value to generate a time-aligned score from an audio input. To address this new challenge, we propose an end-to-end framework that integrates recognition of the note value, pitch, and temporal information. This approach avoids error accumulation inherent in multi-stage methods and enhances accuracy through mutual reinforcement. Our framework employs tokenized representations specifically targeted for this task, through incorporating note value information. Furthermore, we introduce a pseudo-labeling technique to address a scarcity problem of annotated note value data. This technique produces approximate note value labels from existing datasets for the traditional note-level transcription. Experimental results demonstrate the superior performance of the proposed model in note-level transcription tasks when compared to existing state-of-the-art approaches. We also introduce new evaluation metrics that assess both temporal and note value aspects to demonstrate the robustness of the model. Moreover, qualitative assessments via visualized musical scores confirmed the effectiveness of our model in capturing the note values.
* Accepted by IEEE Transactions on Audio, Speech and Language
Processing(TASLP)
Via
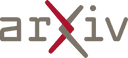
Feb 24, 2025
Abstract:We present X-Dancer, a novel zero-shot music-driven image animation pipeline that creates diverse and long-range lifelike human dance videos from a single static image. As its core, we introduce a unified transformer-diffusion framework, featuring an autoregressive transformer model that synthesize extended and music-synchronized token sequences for 2D body, head and hands poses, which then guide a diffusion model to produce coherent and realistic dance video frames. Unlike traditional methods that primarily generate human motion in 3D, X-Dancer addresses data limitations and enhances scalability by modeling a wide spectrum of 2D dance motions, capturing their nuanced alignment with musical beats through readily available monocular videos. To achieve this, we first build a spatially compositional token representation from 2D human pose labels associated with keypoint confidences, encoding both large articulated body movements (e.g., upper and lower body) and fine-grained motions (e.g., head and hands). We then design a music-to-motion transformer model that autoregressively generates music-aligned dance pose token sequences, incorporating global attention to both musical style and prior motion context. Finally we leverage a diffusion backbone to animate the reference image with these synthesized pose tokens through AdaIN, forming a fully differentiable end-to-end framework. Experimental results demonstrate that X-Dancer is able to produce both diverse and characterized dance videos, substantially outperforming state-of-the-art methods in term of diversity, expressiveness and realism. Code and model will be available for research purposes.
Via
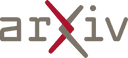
Feb 24, 2025
Abstract:The Musical Instrument Digital Interface (MIDI), introduced in 1983, revolutionized music production by allowing computers and instruments to communicate efficiently. MIDI files encode musical instructions compactly, facilitating convenient music sharing. They benefit Music Information Retrieval (MIR), aiding in research on music understanding, computational musicology, and generative music. The GigaMIDI dataset contains over 1.4 million unique MIDI files, encompassing 1.8 billion MIDI note events and over 5.3 million MIDI tracks. GigaMIDI is currently the largest collection of symbolic music in MIDI format available for research purposes under fair dealing. Distinguishing between non-expressive and expressive MIDI tracks is challenging, as MIDI files do not inherently make this distinction. To address this issue, we introduce a set of innovative heuristics for detecting expressive music performance. These include the Distinctive Note Velocity Ratio (DNVR) heuristic, which analyzes MIDI note velocity; the Distinctive Note Onset Deviation Ratio (DNODR) heuristic, which examines deviations in note onset times; and the Note Onset Median Metric Level (NOMML) heuristic, which evaluates onset positions relative to metric levels. Our evaluation demonstrates these heuristics effectively differentiate between non-expressive and expressive MIDI tracks. Furthermore, after evaluation, we create the most substantial expressive MIDI dataset, employing our heuristic, NOMML. This curated iteration of GigaMIDI encompasses expressively-performed instrument tracks detected by NOMML, containing all General MIDI instruments, constituting 31% of the GigaMIDI dataset, totalling 1,655,649 tracks.
* Published at Transactions of the International Society for Music
Information Retrieval (TISMIR), 8(1), 1-19
Via
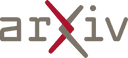