What is Topic Modeling? Topic modeling is a type of statistical modeling for discovering the abstract topics that occur in a collection of documents.
Papers and Code
May 21, 2025
Abstract:We investigate the impacts of NLP research published in top-tier conferences (i.e., ACL, EMNLP, and NAACL) from 1979 to 2024. By analyzing citations from research articles and external sources such as patents, media, and policy documents, we examine how different NLP topics are consumed both within the academic community and by the broader public. Our findings reveal that language modeling has the widest internal and external influence, while linguistic foundations have lower impacts. We also observe that internal and external impacts generally align, but topics like ethics, bias, and fairness show significant attention in policy documents with much fewer academic citations. Additionally, external domains exhibit distinct preferences, with patents focusing on practical NLP applications and media and policy documents engaging more with the societal implications of NLP models.
* 7 pages; Accepted to ACL 2025
Via
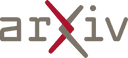
May 22, 2025
Abstract:As open-source language models (OSMs) grow more capable and are widely shared and finetuned, ensuring model provenance, i.e., identifying the origin of a given model instance, has become an increasingly important issue. At the same time, existing backdoor-based model fingerprinting techniques often fall short of achieving key requirements of real-world model ownership detection. In this work, we build on the observation that while current open-source model watermarks fail to achieve reliable content traceability, they can be effectively adapted to address the challenge of model provenance. To this end, we introduce the concept of domain-specific watermarking for model fingerprinting. Rather than watermarking all generated content, we train the model to embed watermarks only within specified subdomains (e.g., particular languages or topics). This targeted approach ensures detection reliability, while improving watermark durability and quality under a range of real-world deployment settings. Our evaluations show that domain-specific watermarking enables model fingerprinting with strong statistical guarantees, controllable false positive rates, high detection power, and preserved generation quality. Moreover, we find that our fingerprints are inherently stealthy and naturally robust to real-world variability across deployment scenarios.
Via
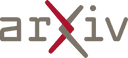
May 22, 2025
Abstract:Biological collections house millions of specimens documenting Earth's biodiversity, with digital images increasingly available through open-access platforms. Most imaging protocols were developed for human visual interpretation without considering computational analysis requirements. This paper aims to bridge the gap between current imaging practices and the potential for automated analysis by presenting key considerations for creating biological specimen images optimized for computer vision applications. We provide conceptual computer vision topics for context, addressing fundamental concerns including model generalization, data leakage, and comprehensive metadata documentation, and outline practical guidance on specimen imagine, and data storage. These recommendations were synthesized through interdisciplinary collaboration between taxonomists, collection managers, ecologists, and computer scientists. Through this synthesis, we have identified ten interconnected considerations that form a framework for successfully integrating biological specimen images into computer vision pipelines. The key elements include: (1) comprehensive metadata documentation, (2) standardized specimen positioning, (3) consistent size and color calibration, (4) protocols for handling multiple specimens in one image, (5) uniform background selection, (6) controlled lighting, (7) appropriate resolution and magnification, (8) optimal file formats, (9) robust data archiving strategies, and (10) accessible data sharing practices. By implementing these recommendations, collection managers, taxonomists, and biodiversity informaticians can generate images that support automated trait extraction, species identification, and novel ecological and evolutionary analyses at unprecedented scales. Successful implementation lies in thorough documentation of methodological choices.
Via
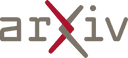
May 27, 2025
Abstract:Scientific paper retrieval is essential for supporting literature discovery and research. While dense retrieval methods demonstrate effectiveness in general-purpose tasks, they often fail to capture fine-grained scientific concepts that are essential for accurate understanding of scientific queries. Recent studies also use large language models (LLMs) for query understanding; however, these methods often lack grounding in corpus-specific knowledge and may generate unreliable or unfaithful content. To overcome these limitations, we propose SemRank, an effective and efficient paper retrieval framework that combines LLM-guided query understanding with a concept-based semantic index. Each paper is indexed using multi-granular scientific concepts, including general research topics and detailed key phrases. At query time, an LLM identifies core concepts derived from the corpus to explicitly capture the query's information need. These identified concepts enable precise semantic matching, significantly enhancing retrieval accuracy. Experiments show that SemRank consistently improves the performance of various base retrievers, surpasses strong existing LLM-based baselines, and remains highly efficient.
Via
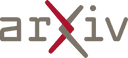
May 22, 2025
Abstract:The widespread use of Large Multimodal Models (LMMs) has raised concerns about model toxicity. However, current research mainly focuses on explicit toxicity, with less attention to some more implicit toxicity regarding prejudice and discrimination. To address this limitation, we introduce a subtler type of toxicity named dual-implicit toxicity and a novel toxicity benchmark termed MDIT-Bench: Multimodal Dual-Implicit Toxicity Benchmark. Specifically, we first create the MDIT-Dataset with dual-implicit toxicity using the proposed Multi-stage Human-in-loop In-context Generation method. Based on this dataset, we construct the MDIT-Bench, a benchmark for evaluating the sensitivity of models to dual-implicit toxicity, with 317,638 questions covering 12 categories, 23 subcategories, and 780 topics. MDIT-Bench includes three difficulty levels, and we propose a metric to measure the toxicity gap exhibited by the model across them. In the experiment, we conducted MDIT-Bench on 13 prominent LMMs, and the results show that these LMMs cannot handle dual-implicit toxicity effectively. The model's performance drops significantly in hard level, revealing that these LMMs still contain a significant amount of hidden but activatable toxicity. Data are available at https://github.com/nuo1nuo/MDIT-Bench.
* Findings of ACL 2025
Via
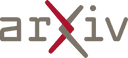
May 16, 2025
Abstract:Recommender systems filter contents/items valuable to users by inferring preferences from user features and historical behaviors. Mainstream approaches follow the learning-to-rank paradigm, which focus on discovering and modeling item topics (e.g., categories), and capturing user preferences on these topics based on historical interactions. However, this paradigm often neglects the modeling of user characteristics and their social roles, which are logical confounders influencing the correlated interest and user preference transition. To bridge this gap, we introduce the user role identification task and the behavioral logic modeling task that aim to explicitly model user roles and learn the logical relations between item topics and user social roles. We show that it is possible to explicitly solve these tasks through an efficient integration framework of Large Language Model (LLM) and recommendation systems, for which we propose TagCF. On the one hand, the exploitation of the LLM's world knowledge and logic inference ability produces a virtual logic graph that reveals dynamic and expressive knowledge of users, augmenting the recommendation performance. On the other hand, the user role aligns the user behavioral logic with the observed user feedback, refining our understanding of user behaviors. Additionally, we also show that the extracted user-item logic graph is empirically a general knowledge that can benefit a wide range of recommendation tasks, and conduct experiments on industrial and several public datasets as verification.
Via
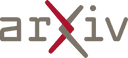
May 19, 2025
Abstract:DeepSeek recently released R1, a high-performing large language model (LLM) optimized for reasoning tasks. Despite its efficient training pipeline, R1 achieves competitive performance, even surpassing leading reasoning models like OpenAI's o1 on several benchmarks. However, emerging reports suggest that R1 refuses to answer certain prompts related to politically sensitive topics in China. While existing LLMs often implement safeguards to avoid generating harmful or offensive outputs, R1 represents a notable shift - exhibiting censorship-like behavior on politically charged queries. In this paper, we investigate this phenomenon by first introducing a large-scale set of heavily curated prompts that get censored by R1, covering a range of politically sensitive topics, but are not censored by other models. We then conduct a comprehensive analysis of R1's censorship patterns, examining their consistency, triggers, and variations across topics, prompt phrasing, and context. Beyond English-language queries, we explore censorship behavior in other languages. We also investigate the transferability of censorship to models distilled from the R1 language model. Finally, we propose techniques for bypassing or removing this censorship. Our findings reveal possible additional censorship integration likely shaped by design choices during training or alignment, raising concerns about transparency, bias, and governance in language model deployment.
Via
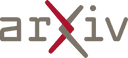
May 28, 2025
Abstract:Large Reasoning Models (LRMs) have made significant progress in mathematical capabilities in recent times. However, these successes have been primarily confined to competition-level problems. In this work, we propose AI Mathematician (AIM) framework, which harnesses the reasoning strength of LRMs to support frontier mathematical research. We have identified two critical challenges of mathematical research compared to competition, {\it the intrinsic complexity of research problems} and {\it the requirement of procedural rigor}. To address these challenges, AIM incorporates two core strategies: an exploration mechanism to foster longer solution paths, and the pessimistic reasonable verification method to ensure reliability. This early version of AIM already exhibits strong capability in tackling research-level tasks. We conducted extensive experiments across several real-world mathematical topics and obtained promising results. AIM is able to autonomously construct substantial portions of proofs and uncover non-trivial insights within each research area. These findings highlight the potential of LRMs in mathematical discovery and suggest that LRM-based agent systems could significantly accelerate mathematical research in the future.
* 95 pages, 1 figure
Via
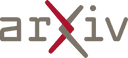
May 22, 2025
Abstract:Written by its inventors, this first tutorial on Beyond-Diagonal Reconfigurable Intelligent Surfaces (BD-RISs) provides the readers with the basics and fundamental tools necessary to appreciate, understand, and contribute to this emerging and disruptive technology. Conventional (Diagonal) RISs (D-RISs) are characterized by a diagonal scattering matrix $\mathbf{\Theta}$ such that the wave manipulation flexibility of D-RIS is extremely limited. In contrast, BD-RIS refers to a novel and general framework for RIS where its scattering matrix is not limited to be diagonal (hence, the ``beyond-diagonal'' terminology) and consequently, all entries of $\mathbf{\Theta}$ can potentially help shaping waves for much higher manipulation flexibility. This physically means that BD-RIS can artificially engineer and reconfigure coupling across elements of the surface thanks to inter-element reconfigurable components which allow waves absorbed by one element to flow through other elements. Consequently, BD-RIS opens the door to more general and versatile intelligent surfaces that subsumes existing RIS architectures as special cases. In this tutorial, we share all the secret sauce to model, design, and optimize BD-RIS and make BD-RIS transformative in many different applications. Topics discussed include physics-consistent and multi-port network-aided modeling; transmitting, reflecting, hybrid, and multi-sector mode analysis; reciprocal and non-reciprocal architecture designs and optimal performance-complexity Pareto frontier of BD-RIS; signal processing, optimization, and channel estimation for BD-RIS; hardware impairments (discrete-value impedance and admittance, lossy interconnections and components, wideband effects, mutual coupling) of BD-RIS; benefits and applications of BD-RIS in communications, sensing, power transfer.
* 42 pages, 37 figures, submitted to IEEE journal for future
publication
Via
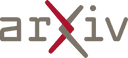
May 21, 2025
Abstract:The surge of user-generated online content presents a wealth of insights into customer preferences and market trends. However, the highly diverse, complex, and context-rich nature of such contents poses significant challenges to traditional opinion mining approaches. To address this, we introduce Online Opinion Mining Benchmark (OOMB), a novel dataset and evaluation protocol designed to assess the ability of large language models (LLMs) to mine opinions effectively from diverse and intricate online environments. OOMB provides extensive (entity, feature, opinion) tuple annotations and a comprehensive opinion-centric summary that highlights key opinion topics within each content, thereby enabling the evaluation of both the extractive and abstractive capabilities of models. Through our proposed benchmark, we conduct a comprehensive analysis of which aspects remain challenging and where LLMs exhibit adaptability, to explore whether they can effectively serve as opinion miners in realistic online scenarios. This study lays the foundation for LLM-based opinion mining and discusses directions for future research in this field.
* 8 pages, 6 figures
Via
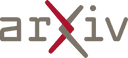