Topic:Time Series Analysis
What is Time Series Analysis? Time series analysis comprises statistical methods for analyzing a sequence of data points collected over an interval of time to identify interesting patterns and trends.
Papers and Code
Mar 09, 2025
Abstract:Recent advances in clinical AI have enabled remarkable progress across many clinical domains. However, existing benchmarks and models are primarily limited to a small set of modalities and tasks, which hinders the development of large-scale multimodal methods that can make holistic assessments of patient health and well-being. To bridge this gap, we introduce Clinical Large-Scale Integrative Multimodal Benchmark (CLIMB), a comprehensive clinical benchmark unifying diverse clinical data across imaging, language, temporal, and graph modalities. CLIMB comprises 4.51 million patient samples totaling 19.01 terabytes distributed across 2D imaging, 3D video, time series, graphs, and multimodal data. Through extensive empirical evaluation, we demonstrate that multitask pretraining significantly improves performance on understudied domains, achieving up to 29% improvement in ultrasound and 23% in ECG analysis over single-task learning. Pretraining on CLIMB also effectively improves models' generalization capability to new tasks, and strong unimodal encoder performance translates well to multimodal performance when paired with task-appropriate fusion strategies. Our findings provide a foundation for new architecture designs and pretraining strategies to advance clinical AI research. Code is released at https://github.com/DDVD233/climb.
Via
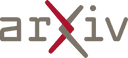
Feb 20, 2025
Abstract:dtaianomaly is an open-source Python library for time series anomaly detection, designed to bridge the gap between academic research and real-world applications. Our goal is to (1) accelerate the development of novel state-of-the-art anomaly detection techniques through simple extensibility; (2) offer functionality for large-scale experimental validation; and thereby (3) bring cutting-edge research to business and industry through a standardized API, similar to scikit-learn to lower the entry barrier for both new and experienced users. Besides these key features, dtaianomaly offers (1) a broad range of built-in anomaly detectors, (2) support for time series preprocessing, (3) tools for visual analysis, (4) confidence prediction of anomaly scores, (5) runtime and memory profiling, (6) comprehensive documentation, and (7) cross-platform unit testing. The source code of dtaianomaly, documentation, code examples and installation guides are publicly available at https://github.com/ML-KULeuven/dtaianomaly.
Via
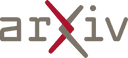
Mar 07, 2025
Abstract:Finance decision-making often relies on in-depth data analysis across various data sources, including financial tables, news articles, stock prices, etc. In this work, we introduce FinTMMBench, the first comprehensive benchmark for evaluating temporal-aware multi-modal Retrieval-Augmented Generation (RAG) systems in finance. Built from heterologous data of NASDAQ 100 companies, FinTMMBench offers three significant advantages. 1) Multi-modal Corpus: It encompasses a hybrid of financial tables, news articles, daily stock prices, and visual technical charts as the corpus. 2) Temporal-aware Questions: Each question requires the retrieval and interpretation of its relevant data over a specific time period, including daily, weekly, monthly, quarterly, and annual periods. 3) Diverse Financial Analysis Tasks: The questions involve 10 different tasks, including information extraction, trend analysis, sentiment analysis and event detection, etc. We further propose a novel TMMHybridRAG method, which first leverages LLMs to convert data from other modalities (e.g., tabular, visual and time-series data) into textual format and then incorporates temporal information in each node when constructing graphs and dense indexes. Its effectiveness has been validated in extensive experiments, but notable gaps remain, highlighting the challenges presented by our FinTMMBench.
* Under review
Via
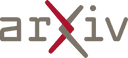
Mar 17, 2025
Abstract:Thermoacoustic instability in annular combustors, essential to aero engines and modern gas turbines, can severely impair operational stability and efficiency, accurately recognizing and understanding various combustion modes is the prerequisite for understanding and controlling combustion instabilities. However, the high-dimensional spatial-temporal dynamics of turbulent flames typically pose considerable challenges to mode recognition. Based on the bidirectional temporal and nonlinear dimensionality reduction models, this study introduces a two-layer bidirectional long short-term memory variational autoencoder, Bi-LSTM-VAE model, to effectively recognize dynamical modes in annular combustion systems. Specifically, leveraging 16 pressure signals from a swirl-stabilized annular combustor, the model maps complex dynamics into a low-dimensional latent space while preserving temporal dependency and nonlinear behavior features through the recurrent neural network structure. The results show that the novel Bi-LSTM-VAE method enables a clear representation of combustion states in two-dimensional state space. Analysis of latent variable distributions reveals distinct patterns corresponding to a wide range of equivalence ratios and premixed fuel and air mass flow rates, offering novel insights into mode classification and transitions, highlighting this model's potential for deciphering complex thermoacoustic phenomena.
* 5 pages, 3 figures
Via
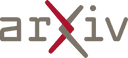
Feb 21, 2025
Abstract:Detecting out-of-distribution (OOD) data is a fundamental challenge in the deployment of machine learning models. From a security standpoint, this is particularly important because OOD test data can result in misleadingly confident yet erroneous predictions, which undermine the reliability of the deployed model. Although numerous models for OOD detection have been developed in computer vision and language, their adaptability to the time-series data domain remains limited and under-explored. Yet, time-series data is ubiquitous across manufacturing and security applications for which OOD is essential. This paper seeks to address this research gap by conducting a comprehensive analysis of modality-agnostic OOD detection algorithms. We evaluate over several multivariate time-series datasets, deep learning architectures, time-series specific data augmentations, and loss functions. Our results demonstrate that: 1) the majority of state-of-the-art OOD methods exhibit limited performance on time-series data, and 2) OOD methods based on deep feature modeling may offer greater advantages for time-series OOD detection, highlighting a promising direction for future time-series OOD detection algorithm development.
* Accepted for an oral presentation at AAAI-25 AI4TS
Via
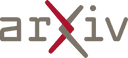
Mar 07, 2025
Abstract:Sea surface temperature (SST) is a fundamental physical parameter characterising the thermal state of sea surface. The Thermal Infrared Sensor (TIRS) onboard Landsat-8, with its 100-meter spatial resolution, offers a unique opportunity to uncover fine-scale coastal SST patterns that would otherwise be overlooked by coarser-resolution thermal sensors. In this study, we first develop an operational approach for SST retrieval from the TIRS sensor, and subsequently propose a novel algorithm for establishing daily SST climatology which serves as the baseline to detect anomalous SST events. We applied the proposed methods to temperate coastal waters in South Australia for the ten-year period from 2014 to 2023. For ground validation purposes, a buoy was deployed off the coast of Port Lincoln, South Australia, to record in-situ time-series SST. The spatiotemporal patterns of SST in the study area were analysed based on the ten years of satellite-derived SST imagery. The daily baseline climatology of SST with 100 m resolution was constructed, which allowed for the detection and analysis of anomalous SST events during the study period of 2014-2023. Our results suggest the following: (1) the satellite-derived SST data, generated with the proposed algorithm, aligned well with the in-situ measured SST values; (2) the semi-enclosed, shallow regions of Upper Spencer Gulf and Upper St Vincent Gulf showed higher temperatures during summer and cooler temperatures during winter than waters closer to the open ocean, resulting in a higher seasonal variation in SST; (3) the near-shore shallow areas in Spencer Gulf and St Vincent Gulf, and regions surrounding Kangaroo Island, were identified to have a higher probability of SST anomalies compared to the rest of the study area; and (4) anomalous SST events were more likely to happen during the warm months than the cool months.
* Submitted to GIScience & Remote Sensing
Via
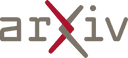
Feb 21, 2025
Abstract:Effective resource management and environmental planning in regions with high climatic variability, such as Chile, demand advanced predictive tools. This study addresses this challenge by employing an innovative and computationally efficient hybrid methodology that integrates machine learning (ML) methods for time series forecasting with established statistical techniques. The spatiotemporal data undergo decomposition using time-dependent Empirical Orthogonal Functions (EOFs), denoted as \(\phi_{k}(t)\), and their corresponding spatial coefficients, \(\alpha_{k}(s)\), to reduce dimensionality. Wavelet analysis provides high-resolution time and frequency information from the \(\phi_{k}(t)\) functions, while neural networks forecast these functions within a medium-range horizon \(h\). By utilizing various ML models, particularly a Wavelet - ANN hybrid model, we forecast \(\phi_{k}(t+h)\) up to a time horizon \(h\), and subsequently reconstruct the spatiotemporal data using these extended EOFs. This methodology is applied to a grid of climate data covering the territory of Chile. It transitions from a high-dimensional multivariate spatiotemporal data forecasting problem to a low-dimensional univariate forecasting problem. Additionally, cluster analysis with Dynamic Time Warping for defining similarities between rainfall time series, along with spatial coherence and predictability assessments, has been instrumental in identifying geographic areas where model performance is enhanced. This approach also elucidates the reasons behind poor forecast performance in regions or clusters with low spatial coherence and predictability. By utilizing cluster medoids, the forecasting process becomes more practical and efficient. This compound approach significantly reduces computational complexity while generating forecasts of reasonable accuracy and utility.
* 25 pages, 6 figures
Via
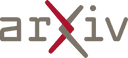
Feb 26, 2025
Abstract:Hutchinson estimators are widely employed in training divergence-based likelihoods for diffusion models to ensure optimal transport (OT) properties. However, this estimator often suffers from high variance and scalability concerns. To address these challenges, we investigate Hutch++, an optimal stochastic trace estimator for generative models, designed to minimize training variance while maintaining transport optimality. Hutch++ is particularly effective for handling ill-conditioned matrices with large condition numbers, which commonly arise when high-dimensional data exhibits a low-dimensional structure. To mitigate the need for frequent and costly QR decompositions, we propose practical schemes that balance frequency and accuracy, backed by theoretical guarantees. Our analysis demonstrates that Hutch++ leads to generations of higher quality. Furthermore, this method exhibits effective variance reduction in various applications, including simulations, conditional time series forecasts, and image generation.
* Accepted by AISTATS 2025
Via
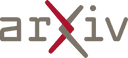
Mar 14, 2025
Abstract:This work presents a three-phase ML prediction framework designed to handle a high dimensionality and multivariate time series character of the electricity market curves. In the preprocessing phase, we transform the original data to achieve a unified structure and mitigate the effect of possible outliers. Further, to address the challenge of high dimensionality, we test three dimensionality reduction techniques (PCA, kPCA, UMAP). Finally, we predict supply and demand curves, once represented in a latent space, with a variety of machine learning methods (RF, LSTM, TSMixer). As our results on the MIBEL dataset show, a high dimensional structure of the market curves can be best handled by the nonlinear reduction technique UMAP. Regardless of the ML technique used for prediction, we achieved the lowest values for all considered precision metrics with a UMAP latent space representation in only two or three dimensions, even when compared to PCA and kPCA with five or six dimensions. Further, we demonstrate that the most promising machine learning technique to handle the complex structure of the electricity market curves is a novel TSMixer architecture. Finally, we fill the gap in the field of electricity market curves prediction literature: in addition to standard analysis on the supply side, we applied the ML framework and predicted demand curves too. We discussed the differences in the achieved results for these two types of curves.
* Submitted to Applied Soft Computing
Via
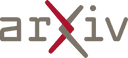
Feb 25, 2025
Abstract:Reconfigurable intelligent surfaces (RISs) have emerged as a promising solution that can provide dynamic control over the propagation of electromagnetic waves. The RIS technology is envisioned as a key enabler of sixth-generation networks by offering the ability to adaptively manipulate signal propagation through the smart configuration of its phase shift coefficients, thereby optimizing signal strength, coverage, and capacity. However, the realization of this technology's full potential hinges on the accurate acquisition of channel state information (CSI). In this paper, we propose an efficient CSI prediction framework for a RIS-assisted communication system based on the machine learning (ML) transformer architecture. Architectural modifications are introduced to the vanilla transformer for multivariate time series forecasting to achieve high prediction accuracy. The predicted channel coefficients are then used to optimize the RIS phase shifts. Simulation results present a comprehensive analysis of key performance metrics, including data rate and outage probability. Our results confirm the effectiveness of the proposed ML approach and demonstrate its superiority over other baseline ML-based CSI prediction schemes such as conventional deep neural networks and long short-term memory architectures, albeit at the cost of slightly increased complexity.
* Linkoping Electronic Conference Proceedings 212 (2024) 441-447
* Accepted for Scandinavian Simulation Society(SIMS) EUROSIM 2024
Via
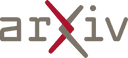