What is Recommendation? Recommendation is the task of providing personalized suggestions to users based on their preferences and behavior.
Papers and Code
May 29, 2025
Abstract:This white paper discusses and explores the various points of intersection between quantum computing and artificial intelligence (AI). It describes how quantum computing could support the development of innovative AI solutions. It also examines use cases of classical AI that can empower research and development in quantum technologies, with a focus on quantum computing and quantum sensing. The purpose of this white paper is to provide a long-term research agenda aimed at addressing foundational questions about how AI and quantum computing interact and benefit one another. It concludes with a set of recommendations and challenges, including how to orchestrate the proposed theoretical work, align quantum AI developments with quantum hardware roadmaps, estimate both classical and quantum resources - especially with the goal of mitigating and optimizing energy consumption - advance this emerging hybrid software engineering discipline, and enhance European industrial competitiveness while considering societal implications.
* 32 pages, 3 figures
Via
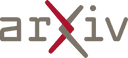
May 29, 2025
Abstract:A restaurant dinner or a hotel stay may lead to memorable experiences when guests encounter unexpected aspects that also match their interests. For example, an origami-making station in the waiting area of a restaurant may be both surprising and enjoyable for a customer who is passionate about paper crafts. Similarly, an exhibit of 18th century harpsichords would be atypical for a hotel lobby and likely pique the interest of a guest who has a passion for Baroque music. Motivated by this insight, in this paper we introduce the new task of engineering serendipity through recommendations of items with atypical aspects. We describe an LLM-based system pipeline that extracts atypical aspects from item reviews, then estimates and aggregates their user-specific utility in a measure of serendipity potential that is used to rerank a list of items recommended to the user. To facilitate system development and evaluation, we introduce a dataset of Yelp reviews that are manually annotated with atypical aspects and a dataset of artificially generated user profiles, together with crowdsourced annotations of user-aspect utility values. Furthermore, we introduce a custom procedure for dynamic selection of in-context learning examples, which is shown to improve LLM-based judgments of atypicality and utility. Experimental evaluations show that serendipity-based rankings generated by the system are highly correlated with ground truth rankings for which serendipity scores are computed from manual annotations of atypical aspects and their user-dependent utility. Overall, we hope that the new recommendation task and the associated system presented in this paper catalyze further research into recommendation approaches that go beyond accuracy in their pursuit of enhanced user satisfaction. The datasets and the code are made publicly available at https://github.com/ramituncc49er/ATARS .
* 25 pages of content + references and appendix. arXiv admin note: text
overlap with arXiv:2311.02702
Via
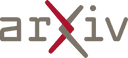
May 29, 2025
Abstract:Small Uncrewed Aerial Systems (sUAS) are increasingly deployed as autonomous swarms in search-and-rescue and other disaster-response scenarios. In these settings, they use computer vision (CV) to detect objects of interest and autonomously adapt their missions. However, traditional CV systems often struggle to recognize unfamiliar objects in open-world environments or to infer their relevance for mission planning. To address this, we incorporate large language models (LLMs) to reason about detected objects and their implications. While LLMs can offer valuable insights, they are also prone to hallucinations and may produce incorrect, misleading, or unsafe recommendations. To ensure safe and sensible decision-making under uncertainty, high-level decisions must be governed by cognitive guardrails. This article presents the design, simulation, and real-world integration of these guardrails for sUAS swarms in search-and-rescue missions.
* 16 pages, 8 figures
Via
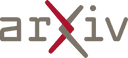
May 29, 2025
Abstract:Large language models (LLMs) have introduced new paradigms for recommender systems by enabling richer semantic understanding and incorporating implicit world knowledge. In this study, we propose a systematic taxonomy that classifies existing approaches into two categories: (1) Pure LLM Recommenders, which rely solely on LLMs, and (2) Augmented LLM Recommenders, which integrate additional non-LLM techniques to enhance performance. This taxonomy provides a novel lens through which to examine the evolving landscape of LLM-based recommendation. To support fair comparison, we introduce a unified evaluation platform that benchmarks representative models under consistent experimental settings, highlighting key design choices that impact effectiveness. We conclude by discussing open challenges and outlining promising directions for future research. This work offers both a comprehensive overview and practical guidance for advancing next-generation LLM-powered recommender.
Via
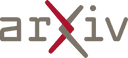
May 29, 2025
Abstract:This paper considers a contextual bandit problem involving multiple agents, where a learner sequentially observes the contexts and the agent's reported arms, and then selects the arm that maximizes the system's overall reward. Existing work in contextual bandits assumes that agents truthfully report their arms, which is unrealistic in many real-life applications. For instance, consider an online platform with multiple sellers; some sellers may misrepresent product quality to gain an advantage, such as having the platform preferentially recommend their products to online users. To address this challenge, we propose an algorithm, COBRA, for contextual bandit problems involving strategic agents that disincentivize their strategic behavior without using any monetary incentives, while having incentive compatibility and a sub-linear regret guarantee. Our experimental results also validate the different performance aspects of our proposed algorithm.
* This paper proposes a contextual bandit algorithm that prevents
strategic agents from misreporting while having approximate incentive
compatibility and a sub-linear regret guarantee
Via
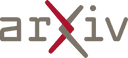
May 29, 2025
Abstract:The advancement of Large Language Models (LLMs) has led to significant improvements in various service domains, including search, recommendation, and chatbot applications. However, applying state-of-the-art (SOTA) research to industrial settings presents challenges, as it requires maintaining flexible conversational abilities while also strictly complying with service-specific constraints. This can be seen as two conflicting requirements due to the probabilistic nature of LLMs. In this paper, we propose our approach to addressing this challenge and detail the strategies we employed to overcome their inherent limitations in real-world applications. We conduct a practical case study of a conversational agent designed for the e-commerce domain, detailing our implementation workflow and optimizations. Our findings provide insights into bridging the gap between academic research and real-world application, introducing a framework for developing scalable, controllable, and reliable AI-driven agents.
* Accepted to ACL 2025 Industry Track. 12 pages, 5 figures
Via
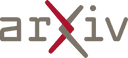
May 29, 2025
Abstract:Modern recommender systems powered by Graph Neural Networks (GNNs) excel at modeling complex user-item interactions, yet increasingly face scenarios requiring selective forgetting of training data. Beyond user requests to remove specific interactions due to privacy concerns or preference changes, regulatory frameworks mandate recommender systems' ability to eliminate the influence of certain user data from models. This recommendation unlearning challenge presents unique difficulties as removing connections within interaction graphs creates ripple effects throughout the model, potentially impacting recommendations for numerous users. Traditional approaches suffer from significant drawbacks: fragmentation methods damage graph structure and diminish performance, while influence function techniques make assumptions that may not hold in complex GNNs, particularly with self-supervised or random architectures. To address these limitations, we propose a novel model-agnostic pre-training paradigm UnlearnRec that prepares systems for efficient unlearning operations. Our Influence Encoder takes unlearning requests together with existing model parameters and directly produces updated parameters of unlearned model with little fine-tuning, avoiding complete retraining while preserving model performance characteristics. Extensive evaluation on public benchmarks demonstrates that our method delivers exceptional unlearning effectiveness while providing more than 10x speedup compared to retraining approaches. We release our method implementation at: https://github.com/HKUDS/UnlearnRec.
* Accepted to SIGIR 2025 Oral
Via
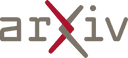
May 29, 2025
Abstract:Recent works of music representation learning mainly focus on learning acoustic music representations with unlabeled audios or further attempt to acquire multi-modal music representations with scarce annotated audio-text pairs. They either ignore the language semantics or rely on labeled audio datasets that are difficult and expensive to create. Moreover, merely modeling semantic space usually fails to achieve satisfactory performance on music recommendation tasks since the user preference space is ignored. In this paper, we propose a novel Hierarchical Two-stage Contrastive Learning (HTCL) method that models similarity from the semantic perspective to the user perspective hierarchically to learn a comprehensive music representation bridging the gap between semantic and user preference spaces. We devise a scalable audio encoder and leverage a pre-trained BERT model as the text encoder to learn audio-text semantics via large-scale contrastive pre-training. Further, we explore a simple yet effective way to exploit interaction data from our online music platform to adapt the semantic space to user preference space via contrastive fine-tuning, which differs from previous works that follow the idea of collaborative filtering. As a result, we obtain a powerful audio encoder that not only distills language semantics from the text encoder but also models similarity in user preference space with the integrity of semantic space preserved. Experimental results on both music semantic and recommendation tasks confirm the effectiveness of our method.
* ICMR 2025
Via
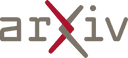
May 29, 2025
Abstract:With the increasing integration of visual and textual content in Social Networking Services (SNS), evaluating the multimodal capabilities of Large Language Models (LLMs) is crucial for enhancing user experience, content understanding, and platform intelligence. Existing benchmarks primarily focus on text-centric tasks, lacking coverage of the multimodal contexts prevalent in modern SNS ecosystems. In this paper, we introduce SNS-Bench-VL, a comprehensive multimodal benchmark designed to assess the performance of Vision-Language LLMs in real-world social media scenarios. SNS-Bench-VL incorporates images and text across 8 multimodal tasks, including note comprehension, user engagement analysis, information retrieval, and personalized recommendation. It comprises 4,001 carefully curated multimodal question-answer pairs, covering single-choice, multiple-choice, and open-ended tasks. We evaluate over 25 state-of-the-art multimodal LLMs, analyzing their performance across tasks. Our findings highlight persistent challenges in multimodal social context comprehension. We hope SNS-Bench-VL will inspire future research towards robust, context-aware, and human-aligned multimodal intelligence for next-generation social networking services.
Via
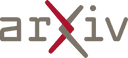
May 28, 2025
Abstract:Systematic reviews (SR), in which experts summarize and analyze evidence across individual studies to provide insights on a specialized topic, are a cornerstone for evidence-based clinical decision-making, research, and policy. Given the exponential growth of scientific articles, there is growing interest in using large language models (LLMs) to automate SR generation. However, the ability of LLMs to critically assess evidence and reason across multiple documents to provide recommendations at the same proficiency as domain experts remains poorly characterized. We therefore ask: Can LLMs match the conclusions of systematic reviews written by clinical experts when given access to the same studies? To explore this question, we present MedEvidence, a benchmark pairing findings from 100 SRs with the studies they are based on. We benchmark 24 LLMs on MedEvidence, including reasoning, non-reasoning, medical specialist, and models across varying sizes (from 7B-700B). Through our systematic evaluation, we find that reasoning does not necessarily improve performance, larger models do not consistently yield greater gains, and knowledge-based fine-tuning degrades accuracy on MedEvidence. Instead, most models exhibit similar behavior: performance tends to degrade as token length increases, their responses show overconfidence, and, contrary to human experts, all models show a lack of scientific skepticism toward low-quality findings. These results suggest that more work is still required before LLMs can reliably match the observations from expert-conducted SRs, even though these systems are already deployed and being used by clinicians. We release our codebase and benchmark to the broader research community to further investigate LLM-based SR systems.
Via
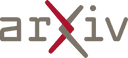