What is Recommendation? Recommendation is the task of providing personalized suggestions to users based on their preferences and behavior.
Papers and Code
Jun 24, 2025
Abstract:Traditional recommendation systems often grapple with "filter bubbles", underutilization of external knowledge, and a disconnect between model optimization and business policy iteration. To address these limitations, this paper introduces RecLLM-R1, a novel recommendation framework leveraging Large Language Models (LLMs) and drawing inspiration from the DeepSeek R1 methodology. The framework initiates by transforming user profiles, historical interactions, and multi-faceted item attributes into LLM-interpretable natural language prompts through a carefully engineered data construction process. Subsequently, a two-stage training paradigm is employed: the initial stage involves Supervised Fine-Tuning (SFT) to imbue the LLM with fundamental recommendation capabilities. The subsequent stage utilizes Group Relative Policy Optimization (GRPO), a reinforcement learning technique, augmented with a Chain-of-Thought (CoT) mechanism. This stage guides the model through multi-step reasoning and holistic decision-making via a flexibly defined reward function, aiming to concurrently optimize recommendation accuracy, diversity, and other bespoke business objectives. Empirical evaluations on a real-world user behavior dataset from a large-scale social media platform demonstrate that RecLLM-R1 significantly surpasses existing baseline methods across a spectrum of evaluation metrics, including accuracy, diversity, and novelty. It effectively mitigates the filter bubble effect and presents a promising avenue for the integrated optimization of recommendation models and policies under intricate business goals.
Via
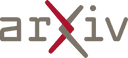
Jun 23, 2025
Abstract:Evaluating Generative 3D models remains challenging due to misalignment between automated metrics and human perception of quality. Current benchmarks rely on image-based metrics that ignore 3D structure or geometric measures that fail to capture perceptual appeal and real-world utility. To address this gap, we present 3D Arena, an open platform for evaluating image-to-3D generation models through large-scale human preference collection using pairwise comparisons. Since launching in June 2024, the platform has collected 123,243 votes from 8,096 users across 19 state-of-the-art models, establishing the largest human preference evaluation for Generative 3D. We contribute the iso3d dataset of 100 evaluation prompts and demonstrate quality control achieving 99.75% user authenticity through statistical fraud detection. Our ELO-based ranking system provides reliable model assessment, with the platform becoming an established evaluation resource. Through analysis of this preference data, we present insights into human preference patterns. Our findings reveal preferences for visual presentation features, with Gaussian splat outputs achieving a 16.6 ELO advantage over meshes and textured models receiving a 144.1 ELO advantage over untextured models. We provide recommendations for improving evaluation methods, including multi-criteria assessment, task-oriented evaluation, and format-aware comparison. The platform's community engagement establishes 3D Arena as a benchmark for the field while advancing understanding of human-centered evaluation in Generative 3D.
* 9 pages, 2 figures
Via
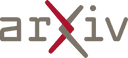
Jun 18, 2025
Abstract:Existing recommendation methods often struggle to model users' multifaceted preferences due to the diversity and volatility of user behavior, as well as the inherent uncertainty and ambiguity of item attributes in practical scenarios. Multi-interest recommendation addresses this challenge by extracting multiple interest representations from users' historical interactions, enabling fine-grained preference modeling and more accurate recommendations. It has drawn broad interest in recommendation research. However, current recommendation surveys have either specialized in frontier recommendation methods or delved into specific tasks and downstream applications. In this work, we systematically review the progress, solutions, challenges, and future directions of multi-interest recommendation by answering the following three questions: (1) Why is multi-interest modeling significantly important for recommendation? (2) What aspects are focused on by multi-interest modeling in recommendation? and (3) How can multi-interest modeling be applied, along with the technical details of the representative modules? We hope that this survey establishes a fundamental framework and delivers a preliminary overview for researchers interested in this field and committed to further exploration. The implementation of multi-interest recommendation summarized in this survey is maintained at https://github.com/WHUIR/Multi-Interest-Recommendation-A-Survey.
Via
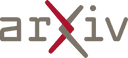
Jun 18, 2025
Abstract:When applicants get rejected by an algorithmic decision system, recourse explanations provide actionable suggestions for how to change their input features to get a positive evaluation. A crucial yet overlooked phenomenon is that recourse explanations are performative: When many applicants act according to their recommendations, their collective behavior may change statistical regularities in the data and, once the model is refitted, also the decision boundary. Consequently, the recourse algorithm may render its own recommendations invalid, such that applicants who make the effort of implementing their recommendations may be rejected again when they reapply. In this work, we formally characterize the conditions under which recourse explanations remain valid under performativity. A key finding is that recourse actions may become invalid if they are influenced by or if they intervene on non-causal variables. Based on our analysis, we caution against the use of standard counterfactual explanations and causal recourse methods, and instead advocate for recourse methods that recommend actions exclusively on causal variables.
* 34 pages, 3 figures, 1 table, Preprint
Via
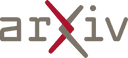
Jun 18, 2025
Abstract:The item cold-start problem is critical for online recommendation systems, as the success of this phase determines whether high-quality new items can transition to popular ones, receive essential feedback to inspire creators, and thus lead to the long-term retention of creators. However, modern recommendation systems still struggle to address item cold-start challenges due to the heavy reliance on item and historical interactions, which are non-trivial for cold-start items lacking sufficient exposure and feedback. Lookalike algorithms provide a promising solution by extending feedback for new items based on lookalike users. Traditional lookalike algorithms face such limitations: (1) failing to effectively model the lookalike users and further improve recommendations with the existing rule- or model-based methods; and (2) struggling to utilize the interaction signals and incorporate diverse features in modern recommendation systems. Inspired by lookalike algorithms, we propose Next-User Retrieval, a novel framework for enhancing cold-start recommendations via generative next-user modeling. Specifically, we employ a transformer-based model to capture the unidirectional relationships among recently interacted users and utilize these sequences to generate the next potential user who is most likely to interact with the item. The additional item features are also integrated as prefix prompt embeddings to assist the next-user generation. The effectiveness of Next-User Retrieval is evaluated through both offline experiments and online A/B tests. Our method achieves significant improvements with increases of 0.0142% in daily active users and +0.1144% in publications in Douyin, showcasing its practical applicability and scalability.
Via
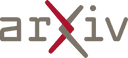
Jun 18, 2025
Abstract:Loss functions play a pivotal role in optimizing recommendation models. Among various loss functions, Softmax Loss (SL) and Cosine Contrastive Loss (CCL) are particularly effective. Their theoretical connections and differences warrant in-depth exploration. This work conducts comprehensive analyses of these losses, yielding significant insights: 1) Common strengths -- both can be viewed as augmentations of traditional losses with Distributional Robust Optimization (DRO), enhancing robustness to distributional shifts; 2) Respective limitations -- stemming from their use of different distribution distance metrics in DRO optimization, SL exhibits high sensitivity to false negative instances, whereas CCL suffers from low data utilization. To address these limitations, this work proposes a new loss function, DrRL, which generalizes SL and CCL by leveraging R\'enyi-divergence in DRO optimization. DrRL incorporates the advantageous structures of both SL and CCL, and can be demonstrated to effectively mitigate their limitations. Extensive experiments have been conducted to validate the superiority of DrRL on both recommendation accuracy and robustness.
* AAAI 2025
Via
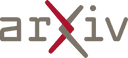
Jun 18, 2025
Abstract:Ensuring equitable public transit access remains challenging, particularly in densely populated cities like New York City (NYC), where low-income and minority communities often face limited transit accessibility. Bike-sharing systems (BSS) can bridge these equity gaps by providing affordable first- and last-mile connections. However, strategically expanding BSS into underserved neighborhoods is difficult due to uncertain bike-sharing demand at newly planned ("cold-start") station locations and limitations in traditional accessibility metrics that may overlook realistic bike usage potential. We introduce Transit for All (TFA), a spatial computing framework designed to guide the equitable expansion of BSS through three components: (1) spatially-informed bike-sharing demand prediction at cold-start stations using region representation learning that integrates multimodal geospatial data, (2) comprehensive transit accessibility assessment leveraging our novel weighted Public Transport Accessibility Level (wPTAL) by combining predicted bike-sharing demand with conventional transit accessibility metrics, and (3) strategic recommendations for new bike station placements that consider potential ridership and equity enhancement. Using NYC as a case study, we identify transit accessibility gaps that disproportionately impact low-income and minority communities in historically underserved neighborhoods. Our results show that strategically placing new stations guided by wPTAL notably reduces disparities in transit access related to economic and demographic factors. From our study, we demonstrate that TFA provides practical guidance for urban planners to promote equitable transit and enhance the quality of life in underserved urban communities.
Via
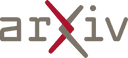
Jun 18, 2025
Abstract:Family caregivers of individuals with Alzheimer's Disease and Related Dementia (AD/ADRD) face significant emotional and logistical challenges that place them at heightened risk for stress, anxiety, and depression. Although recent advances in generative AI -- particularly large language models (LLMs) -- offer new opportunities to support mental health, little is known about how caregivers perceive and engage with such technologies. To address this gap, we developed Carey, a GPT-4o-based chatbot designed to provide informational and emotional support to AD/ADRD caregivers. Using Carey as a technology probe, we conducted semi-structured interviews with 16 family caregivers following scenario-driven interactions grounded in common caregiving stressors. Through inductive coding and reflexive thematic analysis, we surface a systemic understanding of caregiver needs and expectations across six themes -- on-demand information access, emotional support, safe space for disclosure, crisis management, personalization, and data privacy. For each of these themes, we also identified the nuanced tensions in the caregivers' desires and concerns. We present a mapping of caregiver needs, AI chatbot's strengths, gaps, and design recommendations. Our findings offer theoretical and practical insights to inform the design of proactive, trustworthy, and caregiver-centered AI systems that better support the evolving mental health needs of AD/ADRD caregivers.
Via
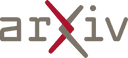
Jun 18, 2025
Abstract:Generative recommendation is emerging as a powerful paradigm that directly generates item predictions, moving beyond traditional matching-based approaches. However, current methods face two key challenges: token-item misalignment, where uniform token-level modeling ignores item-level granularity that is critical for collaborative signal learning, and semantic-collaborative signal entanglement, where collaborative and semantic signals exhibit distinct distributions yet are fused in a unified embedding space, leading to conflicting optimization objectives that limit the recommendation performance. To address these issues, we propose DiscRec, a novel framework that enables Disentangled Semantic-Collaborative signal modeling with flexible fusion for generative Recommendation.First, DiscRec introduces item-level position embeddings, assigned based on indices within each semantic ID, enabling explicit modeling of item structure in input token sequences.Second, DiscRec employs a dual-branch module to disentangle the two signals at the embedding layer: a semantic branch encodes semantic signals using original token embeddings, while a collaborative branch applies localized attention restricted to tokens within the same item to effectively capture collaborative signals. A gating mechanism subsequently fuses both branches while preserving the model's ability to model sequential dependencies. Extensive experiments on four real-world datasets demonstrate that DiscRec effectively decouples these signals and consistently outperforms state-of-the-art baselines. Our codes are available on https://github.com/Ten-Mao/DiscRec.
Via
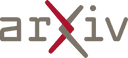
Jun 17, 2025
Abstract:This paper focuses on small-scale one-class classification with some negative samples available. We propose Generalized Reference Kernel with Negative Samples (GRKneg) for One-class Support Vector Machine (OC-SVM). We study different ways to select/generate the reference vectors and recommend an approach for the problem at hand. It is worth noting that the proposed method does not use any labels in the model optimization but uses the original OC-SVM implementation. Only the kernel used in the process is improved using the negative data. We compare our method with the standard OC-SVM and with the binary Support Vector Machine (SVM) using different amounts of negative samples. Our approach consistently outperforms the standard OC-SVM using Radial Basis Function kernel. When there are plenty of negative samples, the binary SVM outperforms the one-class approaches as expected, but we show that for the lowest numbers of negative samples the proposed approach clearly outperforms the binary SVM.
* Accepted to EUSIPCO2025
Via
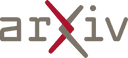