Topic:Image To Image Translation
What is Image To Image Translation? Image-to-image translation is the process of converting an image from one domain to another using deep learning techniques.
Papers and Code
May 12, 2025
Abstract:With the continuous increase in the number and resolution of video surveillance cameras, the burden of transmitting and storing surveillance video is growing. Traditional communication methods based on Shannon's theory are facing optimization bottlenecks. Semantic communication, as an emerging communication method, is expected to break through this bottleneck and reduce the storage and transmission consumption of video. Existing semantic decoding methods often require many samples to train the neural network for each scene, which is time-consuming and labor-intensive. In this study, a semantic encoding and decoding method for surveillance video is proposed. First, the sketch was extracted as semantic information, and a sketch compression method was proposed to reduce the bit rate of semantic information. Then, an image translation network was proposed to translate the sketch into a video frame with a reference frame. Finally, a few-shot sketch decoding network was proposed to reconstruct video from sketch. Experimental results showed that the proposed method achieved significantly better video reconstruction performance than baseline methods. The sketch compression method could effectively reduce the storage and transmission consumption of semantic information with little compromise on video quality. The proposed method provides a novel semantic encoding and decoding method that only needs a few training samples for each surveillance scene, thus improving the practicality of the semantic communication system.
Via
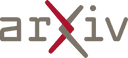
May 12, 2025
Abstract:Generalizing an object detector trained on a single domain to multiple unseen domains is a challenging task. Existing methods typically introduce image or feature augmentation to diversify the source domain to raise the robustness of the detector. Vision-Language Model (VLM)-based augmentation techniques have been proven to be effective, but they require that the detector's backbone has the same structure as the image encoder of VLM, limiting the detector framework selection. To address this problem, we propose Language-Driven Dual Style Mixing (LDDS) for single-domain generalization, which diversifies the source domain by fully utilizing the semantic information of the VLM. Specifically, we first construct prompts to transfer style semantics embedded in the VLM to an image translation network. This facilitates the generation of style diversified images with explicit semantic information. Then, we propose image-level style mixing between the diversified images and source domain images. This effectively mines the semantic information for image augmentation without relying on specific augmentation selections. Finally, we propose feature-level style mixing in a double-pipeline manner, allowing feature augmentation to be model-agnostic and can work seamlessly with the mainstream detector frameworks, including the one-stage, two-stage, and transformer-based detectors. Extensive experiments demonstrate the effectiveness of our approach across various benchmark datasets, including real to cartoon and normal to adverse weather tasks. The source code and pre-trained models will be publicly available at https://github.com/qinhongda8/LDDS.
* The source code and pre-trained models will be publicly available at
https://github.com/qinhongda8/LDDS
Via
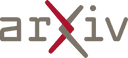
May 05, 2025
Abstract:Automatic segmentation of anatomical landmarks in endoscopic images can provide assistance to doctors and surgeons for diagnosis, treatments or medical training. However, obtaining the annotations required to train commonly used supervised learning methods is a tedious and difficult task, in particular for real images. While ground truth annotations are easier to obtain for synthetic data, models trained on such data often do not generalize well to real data. Generative approaches can add realistic texture to it, but face difficulties to maintain the structure of the original scene. The main contribution in this work is a novel image translation model that adds realistic texture to simulated endoscopic images while keeping the key scene layout information. Our approach produces realistic images in different endoscopy scenarios. We demonstrate these images can effectively be used to successfully train a model for a challenging end task without any real labeled data. In particular, we demonstrate our approach for the task of fold segmentation in colonoscopy images. Folds are key anatomical landmarks that can occlude parts of the colon mucosa and possible polyps. Our approach generates realistic images maintaining the shape and location of the original folds, after the image-style-translation, better than existing methods. We run experiments both on a novel simulated dataset for fold segmentation, and real data from the EndoMapper (EM) dataset. All our new generated data and new EM metadata is being released to facilitate further research, as no public benchmark is currently available for the task of fold segmentation.
* In Int. Workshop on Simulation and Synthesis in Medical Imaging
(pp. 89-101). Springer Nature (2024)
Via
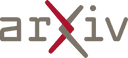
May 22, 2025
Abstract:Recent advances in static 3D generation have intensified the demand for physically consistent dynamic 3D content. However, existing video generation models, including diffusion-based methods, often prioritize visual realism while neglecting physical plausibility, resulting in implausible object dynamics. Prior approaches for physics-aware dynamic generation typically rely on large-scale annotated datasets or extensive model fine-tuning, which imposes significant computational and data collection burdens and limits scalability across scenarios. To address these challenges, we present MAGIC, a training-free framework for single-image physical property inference and dynamic generation, integrating pretrained image-to-video diffusion models with iterative LLM-based reasoning. Our framework generates motion-rich videos from a static image and closes the visual-to-physical gap through a confidence-driven LLM feedback loop that adaptively steers the diffusion model toward physics-relevant motion. To translate visual dynamics into controllable physical behavior, we further introduce a differentiable MPM simulator operating directly on 3D Gaussians reconstructed from the single image, enabling physically grounded, simulation-ready outputs without any supervision or model tuning. Experiments show that MAGIC outperforms existing physics-aware generative methods in inference accuracy and achieves greater temporal coherence than state-of-the-art video diffusion models.
Via
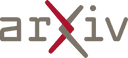
May 06, 2025
Abstract:The acquisition of information-rich images within a limited time budget is crucial in medical imaging. Medical image translation (MIT) can help enhance and supplement existing datasets by generating synthetic images from acquired data. While Generative Adversarial Nets (GANs) and Diffusion Models (DMs) have achieved remarkable success in natural image generation, their benefits - creativity and image realism - do not necessarily transfer to medical applications where highly accurate anatomical information is required. In fact, the imitation of acquisition noise or content hallucination hinder clinical utility. Here, we introduce YODA (You Only Denoise once - or Average), a novel 2.5D diffusion-based framework for volumetric MIT. YODA unites diffusion and regression paradigms to produce realistic or noise-free outputs. Furthermore, we propose Expectation-Approximation (ExpA) DM sampling, which draws inspiration from MRI signal averaging. ExpA-sampling suppresses generated noise and, thus, eliminates noise from biasing the evaluation of image quality. Through extensive experiments on four diverse multi-modal datasets - comprising multi-contrast brain MRI and pelvic MRI-CT - we show that diffusion and regression sampling yield similar results in practice. As such, the computational overhead of diffusion sampling does not provide systematic benefits in medical information translation. Building on these insights, we demonstrate that YODA outperforms several state-of-the-art GAN and DM methods. Notably, YODA-generated images are shown to be interchangeable with, or even superior to, physical acquisitions for several downstream tasks. Our findings challenge the presumed advantages of DMs in MIT and pave the way for the practical application of MIT in medical imaging.
Via
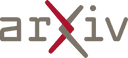
May 18, 2025
Abstract:Vision-Language-Action (VLA) models have recently advanced robotic manipulation by translating natural-language instructions and image information into sequential control actions. However, these models often underperform in open-world scenarios, as they are predominantly trained on successful expert demonstrations and exhibit a limited capacity for failure recovery. In this work, we present a Robotic Failure Analysis and Correction (RoboFAC) framework to address this issue. Firstly, we construct RoboFAC dataset comprising 9,440 erroneous manipulation trajectories and 78,623 QA pairs across 16 diverse tasks and 53 scenes in both simulation and real-world environments. Leveraging our dataset, we develop RoboFAC model, which is capable of Task Understanding, Failure Analysis and Failure Correction. Experimental results demonstrate that the RoboFAC model outperforms GPT-4o by 34.1% on our evaluation benchmark. Furthermore, we integrate the RoboFAC model into a real-world VLA control pipeline as an external supervision providing correction instructions, yielding a 29.1% relative improvement on average on four real-world tasks. The results show that our RoboFAC framework effectively handles robotic failures and assists the VLA model in recovering from failures.
Via
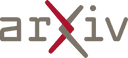
May 26, 2025
Abstract:Diffusion models have become the mainstream architecture for text-to-image generation, achieving remarkable progress in visual quality and prompt controllability. However, current inference pipelines generally lack interpretable semantic supervision and correction mechanisms throughout the denoising process. Most existing approaches rely solely on post-hoc scoring of the final image, prompt filtering, or heuristic resampling strategies-making them ineffective in providing actionable guidance for correcting the generative trajectory. As a result, models often suffer from object confusion, spatial errors, inaccurate counts, and missing semantic elements, severely compromising prompt-image alignment and image quality. To tackle these challenges, we propose MLLM Semantic-Corrected Ping-Pong-Ahead Diffusion (PPAD), a novel framework that, for the first time, introduces a Multimodal Large Language Model (MLLM) as a semantic observer during inference. PPAD performs real-time analysis on intermediate generations, identifies latent semantic inconsistencies, and translates feedback into controllable signals that actively guide the remaining denoising steps. The framework supports both inference-only and training-enhanced settings, and performs semantic correction at only extremely few diffusion steps, offering strong generality and scalability. Extensive experiments demonstrate PPAD's significant improvements.
Via
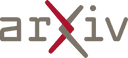
May 21, 2025
Abstract:Vision-Language Models (VLMs) are essential for multimodal tasks, especially compositional reasoning (CR) tasks, which require distinguishing fine-grained semantic differences between visual and textual embeddings. However, existing methods primarily fine-tune the model by generating text-based hard negative samples, neglecting the importance of image-based negative samples, which results in insufficient training of the visual encoder and ultimately impacts the overall performance of the model. Moreover, negative samples are typically treated uniformly, without considering their difficulty levels, and the alignment of positive samples is insufficient, which leads to challenges in aligning difficult sample pairs. To address these issues, we propose Adaptive Hard Negative Perturbation Learning (AHNPL). AHNPL translates text-based hard negatives into the visual domain to generate semantically disturbed image-based negatives for training the model, thereby enhancing its overall performance. AHNPL also introduces a contrastive learning approach using a multimodal hard negative loss to improve the model's discrimination of hard negatives within each modality and a dynamic margin loss that adjusts the contrastive margin according to sample difficulty to enhance the distinction of challenging sample pairs. Experiments on three public datasets demonstrate that our method effectively boosts VLMs' performance on complex CR tasks. The source code is available at https://github.com/nynu-BDAI/AHNPL.
* Accepted at the International Joint Conference on Artificial
Intelligence (IJCAI 2025)
Via
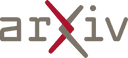
May 14, 2025
Abstract:This study, we propose a novel Q-space Guided Collaborative Attention Translation Networks (Q-CATN) for multi-shell, high-angular resolution DWI (MS-HARDI) synthesis from flexible q-space sampling, leveraging the commonly acquired structural MRI data. Q-CATN employs a collaborative attention mechanism to effectively extract complementary information from multiple modalities and dynamically adjust its internal representations based on flexible q-space information, eliminating the need for fixed sampling schemes. Additionally, we introduce a range of task-specific constraints to preserve anatomical fidelity in DWI, enabling Q-CATN to accurately learn the intrinsic relationships between directional DWI signal distributions and q-space. Extensive experiments on the Human Connectome Project (HCP) dataset demonstrate that Q-CATN outperforms existing methods, including 1D-qDL, 2D-qDL, MESC-SD, and QGAN, in estimating parameter maps and fiber tracts both quantitatively and qualitatively, while preserving fine-grained details. Notably, its ability to accommodate flexible q-space sampling highlights its potential as a promising toolkit for clinical and research applications. Our code is available at https://github.com/Idea89560041/Q-CATN.
* MICCAI 2025
Via
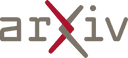
May 12, 2025
Abstract:Background and Objective. Research in the cross-modal medical image translation domain has been very productive over the past few years in tackling the scarce availability of large curated multimodality datasets with the promising performance of GAN-based architectures. However, only a few of these studies assessed task-based related performance of these synthetic data, especially for the training of deep models. Method. We design and compare different GAN-based frameworks for generating synthetic brain [18F]fluorodeoxyglucose (FDG) PET images from T1 weighted MRI data. We first perform standard qualitative and quantitative visual quality evaluation. Then, we explore further impact of using these fake PET data in the training of a deep unsupervised anomaly detection (UAD) model designed to detect subtle epilepsy lesions in T1 MRI and FDG PET images. We introduce novel diagnostic task-oriented quality metrics of the synthetic FDG PET data tailored to our unsupervised detection task, then use these fake data to train a use case UAD model combining a deep representation learning based on siamese autoencoders with a OC-SVM density support estimation model. This model is trained on normal subjects only and allows the detection of any variation from the pattern of the normal population. We compare the detection performance of models trained on 35 paired real MR T1 of normal subjects paired either on 35 true PET images or on 35 synthetic PET images generated from the best performing generative models. Performance analysis is conducted on 17 exams of epilepsy patients undergoing surgery. Results. The best performing GAN-based models allow generating realistic fake PET images of control subject with SSIM and PSNR values around 0.9 and 23.8, respectively and in distribution (ID) with regard to the true control dataset. The best UAD model trained on these synthetic normative PET data allows reaching 74% sensitivity. Conclusion. Our results confirm that GAN-based models are the best suited for MR T1 to FDG PET translation, outperforming transformer or diffusion models. We also demonstrate the diagnostic value of these synthetic data for the training of UAD models and evaluation on clinical exams of epilepsy patients. Our code and the normative image dataset are available.
* Computer Methods and Programs in Biomedicine, 2025, 265, pp.108727
Via
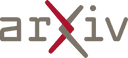