Topic:Infrared And Visible Image Fusion
What is Infrared And Visible Image Fusion? Infrared-and-visible image fusion is the process of combining images from different spectral bands to enhance visual perception.
Papers and Code
Jun 17, 2025
Abstract:In the field of image fusion, promising progress has been made by modeling data from different modalities as linear subspaces. However, in practice, the source images are often located in a non-Euclidean space, where the Euclidean methods usually cannot encapsulate the intrinsic topological structure. Typically, the inner product performed in the Euclidean space calculates the algebraic similarity rather than the semantic similarity, which results in undesired attention output and a decrease in fusion performance. While the balance of low-level details and high-level semantics should be considered in infrared and visible image fusion task. To address this issue, in this paper, we propose a novel attention mechanism based on Grassmann manifold for infrared and visible image fusion (GrFormer). Specifically, our method constructs a low-rank subspace mapping through projection constraints on the Grassmann manifold, compressing attention features into subspaces of varying rank levels. This forces the features to decouple into high-frequency details (local low-rank) and low-frequency semantics (global low-rank), thereby achieving multi-scale semantic fusion. Additionally, to effectively integrate the significant information, we develop a cross-modal fusion strategy (CMS) based on a covariance mask to maximise the complementary properties between different modalities and to suppress the features with high correlation, which are deemed redundant. The experimental results demonstrate that our network outperforms SOTA methods both qualitatively and quantitatively on multiple image fusion benchmarks. The codes are available at https://github.com/Shaoyun2023.
* 16 pages, 11 figures
Via
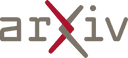
Jun 12, 2025
Abstract:The infrared and visible images fusion (IVIF) is receiving increasing attention from both the research community and industry due to its excellent results in downstream applications. Existing deep learning approaches often utilize convolutional neural networks to extract image features. However, the inherently capacity of convolution operations to capture global context can lead to information loss, thereby restricting fusion performance. To address this limitation, we propose an end-to-end fusion network named the Frequency-Spatial Attention Transformer Fusion Network (FSATFusion). The FSATFusion contains a frequency-spatial attention Transformer (FSAT) module designed to effectively capture discriminate features from source images. This FSAT module includes a frequency-spatial attention mechanism (FSAM) capable of extracting significant features from feature maps. Additionally, we propose an improved Transformer module (ITM) to enhance the ability to extract global context information of vanilla Transformer. We conducted both qualitative and quantitative comparative experiments, demonstrating the superior fusion quality and efficiency of FSATFusion compared to other state-of-the-art methods. Furthermore, our network was tested on two additional tasks without any modifications, to verify the excellent generalization capability of FSATFusion. Finally, the object detection experiment demonstrated the superiority of FSATFusion in downstream visual tasks. Our code is available at https://github.com/Lmmh058/FSATFusion.
Via
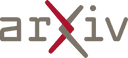
Jun 09, 2025
Abstract:Visible images offer rich texture details, while infrared images emphasize salient targets. Fusing these complementary modalities enhances scene understanding, particularly for advanced vision tasks under challenging conditions. Recently, deep learning-based fusion methods have gained attention, but current evaluations primarily rely on general-purpose metrics without standardized benchmarks or downstream task performance. Additionally, the lack of well-developed dual-spectrum datasets and fair algorithm comparisons hinders progress. To address these gaps, we construct a high-quality dual-spectrum dataset captured in campus environments, comprising 1,369 well-aligned visible-infrared image pairs across four representative scenarios: daytime, nighttime, smoke occlusion, and underpasses. We also propose a comprehensive and fair evaluation framework that integrates fusion speed, general metrics, and object detection performance using the lang-segment-anything model to ensure fairness in downstream evaluation. Extensive experiments benchmark several state-of-the-art fusion algorithms under this framework. Results demonstrate that fusion models optimized for downstream tasks achieve superior performance in target detection, especially in low-light and occluded scenes. Notably, some algorithms that perform well on general metrics do not translate to strong downstream performance, highlighting limitations of current evaluation practices and validating the necessity of our proposed framework. The main contributions of this work are: (1)a campus-oriented dual-spectrum dataset with diverse and challenging scenes; (2) a task-aware, comprehensive evaluation framework; and (3) thorough comparative analysis of leading fusion methods across multiple datasets, offering insights for future development.
* 11 pages, 13 figures
Via
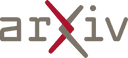
May 27, 2025
Abstract:Image fusion aims to combine complementary information from multiple source images to generate more comprehensive scene representations. Existing methods primarily rely on the stacking and design of network architectures to enhance the fusion performance, often ignoring the impact of dataset scene bias on model training. This oversight leads the model to learn spurious correlations between specific scenes and fusion weights under conventional likelihood estimation framework, thereby limiting fusion performance. To solve the above problems, this paper first re-examines the image fusion task from the causality perspective, and disentangles the model from the impact of bias by constructing a tailored causal graph to clarify the causalities among the variables in image fusion task. Then, the Back-door Adjustment based Feature Fusion Module (BAFFM) is proposed to eliminate confounder interference and enable the model to learn the true causal effect. Finally, Extensive experiments on three standard datasets prove that the proposed method significantly surpasses state-of-the-art methods in infrared and visible image fusion.
Via
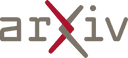
May 11, 2025
Abstract:Acquiring accurately aligned multi-modal image pairs is fundamental for achieving high-quality multi-modal image fusion. To address the lack of ground truth in current multi-modal image registration and fusion methods, we propose a novel self-supervised \textbf{B}i-directional \textbf{S}elf-\textbf{R}egistration framework (\textbf{B-SR}). Specifically, B-SR utilizes a proxy data generator (PDG) and an inverse proxy data generator (IPDG) to achieve self-supervised global-local registration. Visible-infrared image pairs with spatially misaligned differences are aligned to obtain global differences through the registration module. The same image pairs are processed by PDG, such as cropping, flipping, stitching, etc., and then aligned to obtain local differences. IPDG converts the obtained local differences into pseudo-global differences, which are used to perform global-local difference consistency with the global differences. Furthermore, aiming at eliminating the effect of modal gaps on the registration module, we design a neighborhood dynamic alignment loss to achieve cross-modal image edge alignment. Extensive experiments on misaligned multi-modal images demonstrate the effectiveness of the proposed method in multi-modal image alignment and fusion against the competing methods. Our code will be publicly available.
Via
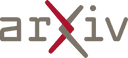
May 10, 2025
Abstract:Visible and infrared image fusion (VIF) has attracted significant attention in recent years. Traditional VIF methods primarily focus on generating fused images with high visual quality, while recent advancements increasingly emphasize incorporating semantic information into the fusion model during training. However, most existing segmentation-oriented VIF methods adopt a cascade structure comprising separate fusion and segmentation models, leading to increased network complexity and redundancy. This raises a critical question: can we design a more concise and efficient structure to integrate semantic information directly into the fusion model during training-Inspired by multi-task learning, we propose a concise and universal training framework, MultiTaskVIF, for segmentation-oriented VIF models. In this framework, we introduce a multi-task head decoder (MTH) to simultaneously output both the fused image and the segmentation result during training. Unlike previous cascade training frameworks that necessitate joint training with a complete segmentation model, MultiTaskVIF enables the fusion model to learn semantic features by simply replacing its decoder with MTH. Extensive experimental evaluations validate the effectiveness of the proposed method. Our code will be released upon acceptance.
Via
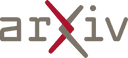
May 26, 2025
Abstract:The real world is dynamic, yet most image fusion methods process static frames independently, ignoring temporal correlations in videos and leading to flickering and temporal inconsistency. To address this, we propose Unified Video Fusion (UniVF), a novel framework for temporally coherent video fusion that leverages multi-frame learning and optical flow-based feature warping for informative, temporally coherent video fusion. To support its development, we also introduce Video Fusion Benchmark (VF-Bench), the first comprehensive benchmark covering four video fusion tasks: multi-exposure, multi-focus, infrared-visible, and medical fusion. VF-Bench provides high-quality, well-aligned video pairs obtained through synthetic data generation and rigorous curation from existing datasets, with a unified evaluation protocol that jointly assesses the spatial quality and temporal consistency of video fusion. Extensive experiments show that UniVF achieves state-of-the-art results across all tasks on VF-Bench. Project page: https://vfbench.github.io.
Via
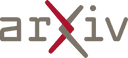
May 21, 2025
Abstract:Multispectral object detection aims to leverage complementary information from visible (RGB) and infrared (IR) modalities to enable robust performance under diverse environmental conditions. In this letter, we propose IC-Fusion, a multispectral object detector that effectively fuses visible and infrared features through a lightweight and modalityaware design. Motivated by wavelet analysis and empirical observations, we find that IR images contain structurally rich high-frequency information critical for object localization, while RGB images provide complementary semantic context. To exploit this, we adopt a compact RGB backbone and design a novel fusion module comprising a Multi-Scale Feature Distillation (MSFD) block to enhance RGB features and a three-stage fusion block with Cross-Modal Channel Shuffle Gate (CCSG) and Cross-Modal Large Kernel Gate (CLKG) to facilitate effective cross-modal interaction. Experiments on the FLIR and LLVIP benchmarks demonstrate the effectiveness and efficiency of our IR-centric fusion strategy. Our code is available at https://github.com/smin-hwang/IC-Fusion.
* Under Review
Via
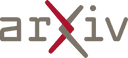
May 05, 2025
Abstract:Visible images provide rich details and color information only under well-lighted conditions while infrared images effectively highlight thermal targets under challenging conditions such as low visibility and adverse weather. Infrared-visible image fusion aims to integrate complementary information from infrared and visible images to generate a high-quality fused image. Existing methods exhibit critical limitations such as neglecting color structure information in visible images and performance degradation when processing low-quality color-visible inputs. To address these issues, we propose a quaternion infrared-visible image fusion (QIVIF) framework to generate high-quality fused images completely in the quaternion domain. QIVIF proposes a quaternion low-visibility feature learning model to adaptively extract salient thermal targets and fine-grained texture details from input infrared and visible images respectively under diverse degraded conditions. QIVIF then develops a quaternion adaptive unsharp masking method to adaptively improve high-frequency feature enhancement with balanced illumination. QIVIF further proposes a quaternion hierarchical Bayesian fusion model to integrate infrared saliency and enhanced visible details to obtain high-quality fused images. Extensive experiments across diverse datasets demonstrate that our QIVIF surpasses state-of-the-art methods under challenging low-visibility conditions.
Via
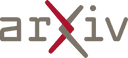
May 07, 2025
Abstract:Visible and infrared image fusion is one of the most crucial tasks in the field of image fusion, aiming to generate fused images with clear structural information and high-quality texture features for high-level vision tasks. However, when faced with severe illumination degradation in visible images, the fusion results of existing image fusion methods often exhibit blurry and dim visual effects, posing major challenges for autonomous driving. To this end, a Darkness-Free network is proposed to handle Visible and infrared image disentanglement and fusion all at Once (DFVO), which employs a cascaded multi-task approach to replace the traditional two-stage cascaded training (enhancement and fusion), addressing the issue of information entropy loss caused by hierarchical data transmission. Specifically, we construct a latent-common feature extractor (LCFE) to obtain latent features for the cascaded tasks strategy. Firstly, a details-extraction module (DEM) is devised to acquire high-frequency semantic information. Secondly, we design a hyper cross-attention module (HCAM) to extract low-frequency information and preserve texture features from source images. Finally, a relevant loss function is designed to guide the holistic network learning, thereby achieving better image fusion. Extensive experiments demonstrate that our proposed approach outperforms state-of-the-art alternatives in terms of qualitative and quantitative evaluations. Particularly, DFVO can generate clearer, more informative, and more evenly illuminated fusion results in the dark environments, achieving best performance on the LLVIP dataset with 63.258 dB PSNR and 0.724 CC, providing more effective information for high-level vision tasks. Our code is publicly accessible at https://github.com/DaVin-Qi530/DFVO.
Via
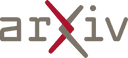