Jun 09, 2025
Abstract:Social media platforms are critical spaces for public discourse, shaping opinions and community dynamics, yet their widespread use has amplified harmful content, particularly hate speech, threatening online safety and inclusivity. While hate speech detection has been extensively studied in languages like English and Spanish, Urdu remains underexplored, especially using translation-based approaches. To address this gap, we introduce a trilingual dataset of 10,193 tweets in English (3,834 samples), Urdu (3,197 samples), and Spanish (3,162 samples), collected via keyword filtering, with a balanced distribution of 4,849 Hateful and 5,344 Not-Hateful labels. Our methodology leverages attention layers as a precursor to transformer-based models and large language models (LLMs), enhancing feature extraction for multilingual hate speech detection. For non-transformer models, we use TF-IDF for feature extraction. The dataset is benchmarked using state-of-the-art models, including GPT-3.5 Turbo and Qwen 2.5 72B, alongside traditional machine learning models like SVM and other transformers (e.g., BERT, RoBERTa). Three annotators, following rigorous guidelines, ensured high dataset quality, achieving a Fleiss' Kappa of 0.821. Our approach, integrating attention layers with GPT-3.5 Turbo and Qwen 2.5 72B, achieves strong performance, with macro F1 scores of 0.87 for English (GPT-3.5 Turbo), 0.85 for Spanish (GPT-3.5 Turbo), 0.81 for Urdu (Qwen 2.5 72B), and 0.88 for the joint multilingual model (Qwen 2.5 72B). These results reflect improvements of 8.75% in English (over SVM baseline 0.80), 8.97% in Spanish (over SVM baseline 0.78), 5.19% in Urdu (over SVM baseline 0.77), and 7.32% in the joint multilingual model (over SVM baseline 0.82). Our framework offers a robust solution for multilingual hate speech detection, fostering safer digital communities worldwide.
Via
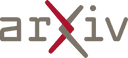