What is music generation? Music generation is the task of generating music or music-like sounds from a model or algorithm.
Papers and Code
Mar 27, 2025
Abstract:Temporal dynamics are among the cues to expres siveness in music performance in different cultures. In the case of Hindustani music, it is well known that expert vocalists often take liberties with the beat, intentionally not aligning their singing precisely with the relatively steady beat provided by the accompanying tabla. This becomes evident when comparing performances of the same composition such as a bandish. We present a methodology for the quantitative study of differences across performed pieces using computational techniques. This is applied to small study of two performances of a popular bandish in raga Yaman, to demonstrate how we can effectively capture the nuances of timing variations that bring out stylistic constraints along with the individual signature of a performer. This work articulates an important step towards the broader goals of music analysis and generative modelling for Indian classical music performance.
Via
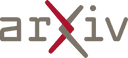
Mar 27, 2025
Abstract:Deep generative models have been used in style transfer tasks for images. In this study, we adapt and improve CycleGAN model to perform music style transfer on Jazz and Classic genres. By doing so, we aim to easily generate new songs, cover music to different music genres and reduce the arrangements needed in those processes. We train and use music genre classifier to assess the performance of the transfer models. To that end, we obtain 87.7% accuracy with Multi-layer Perceptron algorithm. To improve our style transfer baseline, we add auxiliary discriminators and triplet loss to our model. According to our experiments, we obtain the best accuracies as 69.4% in Jazz to Classic task and 39.3% in Classic to Jazz task with our developed genre classifier. We also run a subjective experiment and results of it show that the overall performance of our transfer model is good and it manages to conserve melody of inputs on the transferred outputs. Our code is available at https://github.com/ fidansamet/tune-it-up
Via
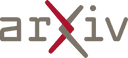
Mar 11, 2025
Abstract:We tackle the task of long-form music generation--particularly the challenging \textbf{lyrics-to-song} problem--by introducing YuE, a family of open foundation models based on the LLaMA2 architecture. Specifically, YuE scales to trillions of tokens and generates up to five minutes of music while maintaining lyrical alignment, coherent musical structure, and engaging vocal melodies with appropriate accompaniment. It achieves this through (1) track-decoupled next-token prediction to overcome dense mixture signals, (2) structural progressive conditioning for long-context lyrical alignment, and (3) a multitask, multiphase pre-training recipe to converge and generalize. In addition, we redesign the in-context learning technique for music generation, enabling versatile style transfer (e.g., converting Japanese city pop into an English rap while preserving the original accompaniment) and bidirectional generation. Through extensive evaluation, we demonstrate that YuE matches or even surpasses some of the proprietary systems in musicality and vocal agility. In addition, fine-tuning YuE enables additional controls and enhanced support for tail languages. Furthermore, beyond generation, we show that YuE's learned representations can perform well on music understanding tasks, where the results of YuE match or exceed state-of-the-art methods on the MARBLE benchmark. Keywords: lyrics2song, song generation, long-form, foundation model, music generation
Via
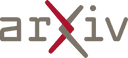
Apr 06, 2025
Abstract:Recent advances in dance generation have enabled automatic synthesis of 3D dance motions. However, existing methods still struggle to produce high-fidelity dance sequences that simultaneously deliver exceptional realism, precise dance-music synchronization, high motion diversity, and physical plausibility. Moreover, existing methods lack the flexibility to edit dance sequences according to diverse guidance signals, such as musical prompts, pose constraints, action labels, and genre descriptions, significantly restricting their creative utility and adaptability. Unlike the existing approaches, DanceMosaic enables fast and high-fidelity dance generation, while allowing multimodal motion editing. Specifically, we propose a multimodal masked motion model that fuses the text-to-motion model with music and pose adapters to learn probabilistic mapping from diverse guidance signals to high-quality dance motion sequences via progressive generative masking training. To further enhance the motion generation quality, we propose multimodal classifier-free guidance and inference-time optimization mechanism that further enforce the alignment between the generated motions and the multimodal guidance. Extensive experiments demonstrate that our method establishes a new state-of-the-art performance in dance generation, significantly advancing the quality and editability achieved by existing approaches.
Via
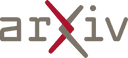
Mar 14, 2025
Abstract:Music-to-music-video generation is a challenging task due to the intrinsic differences between the music and video modalities. The advent of powerful text-to-video diffusion models has opened a promising pathway for music-video (MV) generation by first addressing the music-to-MV description task and subsequently leveraging these models for video generation. In this study, we focus on the MV description generation task and propose a comprehensive pipeline encompassing training data construction and multimodal model fine-tuning. We fine-tune existing pre-trained multimodal models on our newly constructed music-to-MV description dataset based on the Music4All dataset, which integrates both musical and visual information. Our experimental results demonstrate that music representations can be effectively mapped to textual domains, enabling the generation of meaningful MV description directly from music inputs. We also identify key components in the dataset construction pipeline that critically impact the quality of MV description and highlight specific musical attributes that warrant greater focus for improved MV description generation.
* Accepted by RepL4NLP 2025 @ NAACL 2025
Via
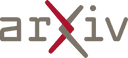
Mar 29, 2025
Abstract:Music similarity retrieval is fundamental for managing and exploring relevant content from large collections in streaming platforms. This paper presents a novel cross-modal contrastive learning framework that leverages the open-ended nature of text descriptions to guide music similarity modeling, addressing the limitations of traditional uni-modal approaches in capturing complex musical relationships. To overcome the scarcity of high-quality text-music paired data, this paper introduces a dual-source data acquisition approach combining online scraping and LLM-based prompting, where carefully designed prompts leverage LLMs' comprehensive music knowledge to generate contextually rich descriptions. Exten1sive experiments demonstrate that the proposed framework achieves significant performance improvements over existing benchmarks through objective metrics, subjective evaluations, and real-world A/B testing on the Huawei Music streaming platform.
* Accepted by ICME2025
Via
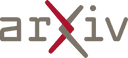
Mar 14, 2025
Abstract:Musical expressivity and coherence are indispensable in music composition and performance, while often neglected in modern AI generative models. In this work, we introduce a listening-based data-processing technique that captures the expressivity in musical performance. This technique derived from Weber's law reflects the human perceptual truth of listening and preserves musical subtlety and expressivity in the training input. To facilitate musical coherence, we model the output interdependencies among multiple arguments in the music data such as pitch, duration, velocity, etc. in the neural networks based on the probabilistic chain rule. In practice, we decompose the multi-output sequential model into single-output submodels and condition previously sampled outputs on the subsequent submodels to induce conditional distributions. Finally, to select eligible sequences from all generations, a tentative measure based on the output entropy was proposed. The entropy sequence is set as a criterion to select predictable and stable generations, which is further studied under the context of informational aesthetic measures to quantify musical pleasure and information gain along the music tendency.
* 7 pages, 4 figures
Via
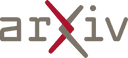
Apr 14, 2025
Abstract:Patterns are fundamental to human cognition, enabling the recognition of structure and regularity across diverse domains. In this work, we focus on structural repeats, patterns that arise from the repetition of hierarchical relations within sequential data, and develop a candidate computational model of how humans detect and understand such structural repeats. Based on a weighted deduction system, our model infers the minimal generative process of a given sequence in the form of a Template program, a formalism that enriches the context-free grammar with repetition combinators. Such representation efficiently encodes the repetition of sub-computations in a recursive manner. As a proof of concept, we demonstrate the expressiveness of our model on short sequences from music and action planning. The proposed model offers broader insights into the mental representations and cognitive mechanisms underlying human pattern recognition.
Via
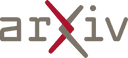
Mar 13, 2025
Abstract:Audio and music generation have emerged as crucial tasks in many applications, yet existing approaches face significant limitations: they operate in isolation without unified capabilities across modalities, suffer from scarce high-quality, multi-modal training data, and struggle to effectively integrate diverse inputs. In this work, we propose AudioX, a unified Diffusion Transformer model for Anything-to-Audio and Music Generation. Unlike previous domain-specific models, AudioX can generate both general audio and music with high quality, while offering flexible natural language control and seamless processing of various modalities including text, video, image, music, and audio. Its key innovation is a multi-modal masked training strategy that masks inputs across modalities and forces the model to learn from masked inputs, yielding robust and unified cross-modal representations. To address data scarcity, we curate two comprehensive datasets: vggsound-caps with 190K audio captions based on the VGGSound dataset, and V2M-caps with 6 million music captions derived from the V2M dataset. Extensive experiments demonstrate that AudioX not only matches or outperforms state-of-the-art specialized models, but also offers remarkable versatility in handling diverse input modalities and generation tasks within a unified architecture. The code and datasets will be available at https://zeyuet.github.io/AudioX/
* The code and datasets will be available at
https://zeyuet.github.io/AudioX/
Via
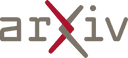
Apr 09, 2025
Abstract:The rapid advancement of audio generation technologies has escalated the risks of malicious deepfake audio across speech, sound, singing voice, and music, threatening multimedia security and trust. While existing countermeasures (CMs) perform well in single-type audio deepfake detection (ADD), their performance declines in cross-type scenarios. This paper is dedicated to studying the alltype ADD task. We are the first to comprehensively establish an all-type ADD benchmark to evaluate current CMs, incorporating cross-type deepfake detection across speech, sound, singing voice, and music. Then, we introduce the prompt tuning self-supervised learning (PT-SSL) training paradigm, which optimizes SSL frontend by learning specialized prompt tokens for ADD, requiring 458x fewer trainable parameters than fine-tuning (FT). Considering the auditory perception of different audio types,we propose the wavelet prompt tuning (WPT)-SSL method to capture type-invariant auditory deepfake information from the frequency domain without requiring additional training parameters, thereby enhancing performance over FT in the all-type ADD task. To achieve an universally CM, we utilize all types of deepfake audio for co-training. Experimental results demonstrate that WPT-XLSR-AASIST achieved the best performance, with an average EER of 3.58% across all evaluation sets. The code is available online.
Via
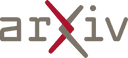