Aug 13, 2024
Abstract:With the goal of uncovering the challenges faced by European AI students during their research endeavors, we surveyed 28 AI doctoral candidates from 13 European countries. The outcomes underscore challenges in three key areas: (1) the findability and quality of AI resources such as datasets, models, and experiments; (2) the difficulties in replicating the experiments in AI papers; (3) and the lack of trustworthiness and interdisciplinarity. From our findings, it appears that although early stage AI researchers generally tend to share their AI resources, they lack motivation or knowledge to engage more in dataset and code preparation and curation, and ethical assessments, and are not used to cooperate with well-versed experts in application domains. Furthermore, we examine existing practices in data governance and reproducibility both in computer science and in artificial intelligence. For instance, only a minority of venues actively promote reproducibility initiatives such as reproducibility evaluations. Critically, there is need for immediate adoption of responsible and reproducible AI research practices, crucial for society at large, and essential for the AI research community in particular. This paper proposes a combination of social and technical recommendations to overcome the identified challenges. Socially, we propose the general adoption of reproducibility initiatives in AI conferences and journals, as well as improved interdisciplinary collaboration, especially in data governance practices. On the technical front, we call for enhanced tools to better support versioning control of datasets and code, and a computing infrastructure that facilitates the sharing and discovery of AI resources, as well as the sharing, execution, and verification of experiments.
* 8 pages, 4 figures, 1 appendix (interview questions)
Via
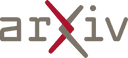