Jun 12, 2025
Abstract:Artificial Intelligence (AI)-powered features have rapidly proliferated across mobile apps in various domains, including productivity, education, entertainment, and creativity. However, how users perceive, evaluate, and critique these AI features remains largely unexplored, primarily due to the overwhelming volume of user feedback. In this work, we present the first comprehensive, large-scale study of user feedback on AI-powered mobile apps, leveraging a curated dataset of 292 AI-driven apps across 14 categories with 894K AI-specific reviews from Google Play. We develop and validate a multi-stage analysis pipeline that begins with a human-labeled benchmark and systematically evaluates large language models (LLMs) and prompting strategies. Each stage, including review classification, aspect-sentiment extraction, and clustering, is validated for accuracy and consistency. Our pipeline enables scalable, high-precision analysis of user feedback, extracting over one million aspect-sentiment pairs clustered into 18 positive and 15 negative user topics. Our analysis reveals that users consistently focus on a narrow set of themes: positive comments emphasize productivity, reliability, and personalized assistance, while negative feedback highlights technical failures (e.g., scanning and recognition), pricing concerns, and limitations in language support. Our pipeline surfaces both satisfaction with one feature and frustration with another within the same review. These fine-grained, co-occurring sentiments are often missed by traditional approaches that treat positive and negative feedback in isolation or rely on coarse-grained analysis. To this end, our approach provides a more faithful reflection of the real-world user experiences with AI-powered apps. Category-aware analysis further uncovers both universal drivers of satisfaction and domain-specific frustrations.
* 12 pages, 6 figures, 5 tables
Via
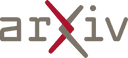