What is Recommendation? Recommendation is the task of providing personalized suggestions to users based on their preferences and behavior.
Papers and Code
May 27, 2025
Abstract:Fairness in federated learning has emerged as a rapidly growing area of research, with numerous works proposing formal definitions and algorithmic interventions. Yet, despite this technical progress, fairness in FL is often defined and evaluated in ways that abstract away from the sociotechnical contexts in which these systems are deployed. In this paper, we argue that existing approaches tend to optimize narrow system level metrics, such as performance parity or contribution-based rewards, while overlooking how harms arise throughout the FL lifecycle and how they impact diverse stakeholders. We support this claim through a critical analysis of the literature, based on a systematic annotation of papers for their fairness definitions, design decisions, evaluation practices, and motivating use cases. Our analysis reveals five recurring pitfalls: 1) fairness framed solely through the lens of server client architecture, 2) a mismatch between simulations and motivating use-cases and contexts, 3) definitions that conflate protecting the system with protecting its users, 4) interventions that target isolated stages of the lifecycle while neglecting upstream and downstream effects, 5) and a lack of multi-stakeholder alignment where multiple fairness definitions can be relevant at once. Building on these insights, we propose a harm centered framework that links fairness definitions to concrete risks and stakeholder vulnerabilities. We conclude with recommendations for more holistic, context-aware, and accountable fairness research in FL.
Via
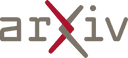
May 26, 2025
Abstract:Text-to-Image (T2I) models have demonstrated impressive capabilities in generating high-quality and diverse visual content from natural language prompts. However, uncontrolled reproduction of sensitive, copyrighted, or harmful imagery poses serious ethical, legal, and safety challenges. To address these concerns, the concept erasure paradigm has emerged as a promising direction, enabling the selective removal of specific semantic concepts from generative models while preserving their overall utility. This survey provides a comprehensive overview and in-depth synthesis of concept erasure techniques in T2I diffusion models. We systematically categorize existing approaches along three key dimensions: intervention level, which identifies specific model components targeted for concept removal; optimization structure, referring to the algorithmic strategies employed to achieve suppression; and semantic scope, concerning the complexity and nature of the concepts addressed. This multi-dimensional taxonomy enables clear, structured comparisons across diverse methodologies, highlighting fundamental trade-offs between erasure specificity, generalization, and computational complexity. We further discuss current evaluation benchmarks, standardized metrics, and practical datasets, emphasizing gaps that limit comprehensive assessment, particularly regarding robustness and practical effectiveness. Finally, we outline major challenges and promising future directions, including disentanglement of concept representations, adaptive and incremental erasure strategies, adversarial robustness, and new generative architectures. This survey aims to guide researchers toward safer, more ethically aligned generative models, providing foundational knowledge and actionable recommendations to advance responsible development in generative AI.
Via
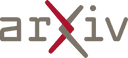
May 26, 2025
Abstract:Regret minimization in stochastic non-stationary bandits gained popularity over the last decade, as it can model a broad class of real-world problems, from advertising to recommendation systems. Existing literature relies on various assumptions about the reward-generating process, such as Bernoulli or subgaussian rewards. However, in settings such as finance and telecommunications, heavy-tailed distributions naturally arise. In this work, we tackle the heavy-tailed piecewise-stationary bandit problem. Heavy-tailed bandits, introduced by Bubeck et al., 2013, operate on the minimal assumption that the finite absolute centered moments of maximum order $1+\epsilon$ are uniformly bounded by a constant $v<+\infty$, for some $\epsilon \in (0,1]$. We focus on the most popular non-stationary bandit setting, i.e., the piecewise-stationary setting, in which the mean of reward-generating distributions may change at unknown time steps. We provide a novel Catoni-style change-point detection strategy tailored for heavy-tailed distributions that relies on recent advancements in the theory of sequential estimation, which is of independent interest. We introduce Robust-CPD-UCB, which combines this change-point detection strategy with optimistic algorithms for bandits, providing its regret upper bound and an impossibility result on the minimum attainable regret for any policy. Finally, we validate our approach through numerical experiments on synthetic and real-world datasets.
Via
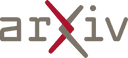
May 26, 2025
Abstract:Large language models (LLMs) have shown remarkable performance across diverse reasoning and generation tasks, and are increasingly deployed as agents in dynamic environments such as code generation and recommendation systems. However, many real-world applications, such as high-frequency trading and real-time competitive gaming, require decisions under strict latency constraints, where faster responses directly translate into higher rewards. Despite the importance of this latency quality trade off, it remains underexplored in the context of LLM based agents. In this work, we present the first systematic study of this trade off in real time decision making tasks. To support our investigation, we introduce two new benchmarks: HFTBench, a high frequency trading simulation, and StreetFighter, a competitive gaming platform. Our analysis reveals that optimal latency quality balance varies by task, and that sacrificing quality for lower latency can significantly enhance downstream performance. To address this, we propose FPX, an adaptive framework that dynamically selects model size and quantization level based on real time demands. Our method achieves the best performance on both benchmarks, improving win rate by up to 80% in Street Fighter and boosting daily yield by up to 26.52% in trading, underscoring the need for latency aware evaluation and deployment strategies for LLM based agents. These results demonstrate the critical importance of latency aware evaluation and deployment strategies for real world LLM based agents. Our benchmarks are available at Latency Sensitive Benchmarks.
Via
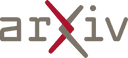
May 26, 2025
Abstract:Multi-Domain Recommendation (MDR) achieves the desirable recommendation performance by effectively utilizing the transfer information across different domains. Despite the great success, most existing MDR methods adopt a single structure to transfer complex domain-shared knowledge. However, the beneficial transferring information should vary across different domains. When there is knowledge conflict between domains or a domain is of poor quality, unselectively leveraging information from all domains will lead to a serious Negative Transfer Problem (NTP). Therefore, how to effectively model the complex transfer relationships between domains to avoid NTP is still a direction worth exploring. To address these issues, we propose a simple and dynamic Similar Domain Selection Principle (SDSP) for multi-domain recommendation in this paper. SDSP presents the initial exploration of selecting suitable domain knowledge for each domain to alleviate NTP. Specifically, we propose a novel prototype-based domain distance measure to effectively model the complexity relationship between domains. Thereafter, the proposed SDSP can dynamically find similar domains for each domain based on the supervised signals of the domain metrics and the unsupervised distance measure from the learned domain prototype. We emphasize that SDSP is a lightweight method that can be incorporated with existing MDR methods for better performance while not introducing excessive time overheads. To the best of our knowledge, it is the first solution that can explicitly measure domain-level gaps and dynamically select appropriate domains in the MDR field. Extensive experiments on three datasets demonstrate the effectiveness of our proposed method.
* Accepted by KDD 2025
Via
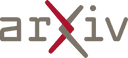
May 26, 2025
Abstract:The affective attitude of liking a recommended item reflects just one category in a wide spectrum of affective phenomena that also includes emotions such as entranced or intrigued, moods such as cheerful or buoyant, as well as more fine-grained affective states, such as "pleasantly surprised by the conclusion". In this paper, we introduce a novel recommendation task that can leverage a virtually unbounded range of affective states sought explicitly by the user in order to identify items that, upon consumption, are likely to induce those affective states. Correspondingly, we create a large dataset of user preferences containing expressions of fine-grained affective states that are mined from book reviews, and propose a Transformer-based architecture that leverages such affective expressions as input. We then use the resulting dataset of affective states preferences, together with the linked users and their histories of book readings, ratings, and reviews, to train and evaluate multiple recommendation models on the task of matching recommended items with affective preferences. Experiments show that the best results are obtained by models that can utilize textual descriptions of items and user affective preferences.
Via
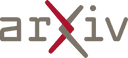
May 26, 2025
Abstract:Timely and personalized treatment decisions are essential across a wide range of healthcare settings where patient responses vary significantly and evolve over time. Clinical data used to support these decisions are often irregularly sampled, sparse, and noisy. Existing decision support systems commonly rely on discretization and imputation, which can distort critical temporal dynamics and degrade decision quality. Moreover, they often overlook the clinical significance of irregular recording frequencies, filtering out patterns in how and when data is collected. Reinforcement Learning (RL) is a natural fit for clinical decision-making, enabling sequential, long-term optimization in dynamic, uncertain environments. However, most existing treatment recommendation systems are model-free and trained solely on offline data, making them sample-inefficient, sensitive to data quality, and poorly generalizable across tasks or cohorts. To address these limitations, we propose MedDreamer, a two-phase model-based RL framework for personalized treatment recommendation. MedDreamer uses a world model with an Adaptive Feature Integration (AFI) module to effectively model irregular, sparse clinical data. Through latent imagination, it simulates plausible patient trajectories to enhance learning, refining its policy using a mix of real and imagined experiences. This enables learning policies that go beyond suboptimal historical decisions while remaining grounded in clinical data. To our knowledge, this is the first application of latent imagination to irregular healthcare data. Evaluations on sepsis and mechanical ventilation (MV) treatment using two large-scale EHR datasets show that MedDreamer outperforms both model-free and model-based baselines in clinical outcomes and off-policy metrics.
Via
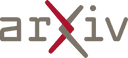
May 26, 2025
Abstract:Large Language Models (LLMs) have garnered significant attention in Recommendation Systems (RS) due to their extensive world knowledge and robust reasoning capabilities. However, a critical challenge lies in enabling LLMs to effectively comprehend and extract insights from massive user behaviors. Current approaches that directly leverage LLMs for user interest learning face limitations in handling long sequential behaviors, effectively extracting interest, and applying interest in practical scenarios. To address these issues, we propose a Hierarchical Tree Search-based User Lifelong Behavior Modeling framework (HiT-LBM). HiT-LBM integrates Chunked User Behavior Extraction (CUBE) and Hierarchical Tree Search for Interest (HTS) to capture diverse interests and interest evolution of user. CUBE divides user lifelong behaviors into multiple chunks and learns the interest and interest evolution within each chunk in a cascading manner. HTS generates candidate interests through hierarchical expansion and searches for the optimal interest with process rating model to ensure information gain for each behavior chunk. Additionally, we design Temporal-Ware Interest Fusion (TIF) to integrate interests from multiple behavior chunks, constructing a comprehensive representation of user lifelong interests. The representation can be embedded into any recommendation model to enhance performance. Extensive experiments demonstrate the effectiveness of our approach, showing that it surpasses state-of-the-art methods.
Via
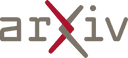
May 26, 2025
Abstract:In this paper, we focus on the often-overlooked issue of embedding collapse in existing diffusion-based sequential recommendation models and propose ADRec, an innovative framework designed to mitigate this problem. Diverging from previous diffusion-based methods, ADRec applies an independent noise process to each token and performs diffusion across the entire target sequence during training. ADRec captures token interdependency through auto-regression while modeling per-token distributions through token-level diffusion. This dual approach enables the model to effectively capture both sequence dynamics and item representations, overcoming the limitations of existing methods. To further mitigate embedding collapse, we propose a three-stage training strategy: (1) pre-training the embedding weights, (2) aligning these weights with the ADRec backbone, and (3) fine-tuning the model. During inference, ADRec applies the denoising process only to the last token, ensuring that the meaningful patterns in historical interactions are preserved. Our comprehensive empirical evaluation across six datasets underscores the effectiveness of ADRec in enhancing both the accuracy and efficiency of diffusion-based sequential recommendation systems.
* Proceedings of the 31st ACM SIGKDD Conference on Knowledge
Discovery and Data Mining (KDD '25), ACM, 2025
Via
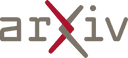
May 26, 2025
Abstract:As Large Language Models (LLMs) become deeply integrated into human life and increasingly influence decision-making, it's crucial to evaluate whether and to what extent they exhibit subjective preferences, opinions, and beliefs. These tendencies may stem from biases within the models, which may shape their behavior, influence the advice and recommendations they offer to users, and potentially reinforce certain viewpoints. This paper presents the Preference, Opinion, and Belief survey (POBs), a benchmark developed to assess LLMs' subjective inclinations across societal, cultural, ethical, and personal domains. We applied our benchmark to evaluate leading open- and closed-source LLMs, measuring desired properties such as reliability, neutrality, and consistency. In addition, we investigated the effect of increasing the test-time compute, through reasoning and self-reflection mechanisms, on those metrics. While effective in other tasks, our results show that these mechanisms offer only limited gains in our domain. Furthermore, we reveal that newer model versions are becoming less consistent and more biased toward specific viewpoints, highlighting a blind spot and a concerning trend. POBS: https://ibm.github.io/POBS
Via
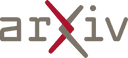