Topic:Information Extraction
What is Information Extraction? Information extraction is the process of automatically extracting structured information from unstructured text data.
Papers and Code
Jul 02, 2025
Abstract:This paper addresses the challenges posed by the unstructured nature and high-dimensional semantic complexity of electronic health record texts. A deep learning method based on attention mechanisms is proposed to achieve unified modeling for information extraction and multi-label disease prediction. The study is conducted on the MIMIC-IV dataset. A Transformer-based architecture is used to perform representation learning over clinical text. Multi-layer self-attention mechanisms are employed to capture key medical entities and their contextual relationships. A Sigmoid-based multi-label classifier is then applied to predict multiple disease labels. The model incorporates a context-aware semantic alignment mechanism, enhancing its representational capacity in typical medical scenarios such as label co-occurrence and sparse information. To comprehensively evaluate model performance, a series of experiments were conducted, including baseline comparisons, hyperparameter sensitivity analysis, data perturbation studies, and noise injection tests. Results demonstrate that the proposed method consistently outperforms representative existing approaches across multiple performance metrics. The model maintains strong generalization under varying data scales, interference levels, and model depth configurations. The framework developed in this study offers an efficient algorithmic foundation for processing real-world clinical texts and presents practical significance for multi-label medical text modeling tasks.
Via
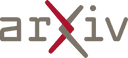
Jul 03, 2025
Abstract:Articulated object manipulation remains a critical challenge in robotics due to the complex kinematic constraints and the limited physical reasoning of existing methods. In this work, we introduce ArtGS, a novel framework that extends 3D Gaussian Splatting (3DGS) by integrating visual-physical modeling for articulated object understanding and interaction. ArtGS begins with multi-view RGB-D reconstruction, followed by reasoning with a vision-language model (VLM) to extract semantic and structural information, particularly the articulated bones. Through dynamic, differentiable 3DGS-based rendering, ArtGS optimizes the parameters of the articulated bones, ensuring physically consistent motion constraints and enhancing the manipulation policy. By leveraging dynamic Gaussian splatting, cross-embodiment adaptability, and closed-loop optimization, ArtGS establishes a new framework for efficient, scalable, and generalizable articulated object modeling and manipulation. Experiments conducted in both simulation and real-world environments demonstrate that ArtGS significantly outperforms previous methods in joint estimation accuracy and manipulation success rates across a variety of articulated objects. Additional images and videos are available on the project website: https://sites.google.com/view/artgs/home
* Accepted by IROS 2025
Via
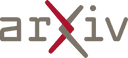
Jul 02, 2025
Abstract:While existing auditing techniques attempt to identify potential unwanted behaviours in large language models (LLMs), we address the complementary forensic problem of reconstructing the exact input that led to an existing LLM output - enabling post-incident analysis and potentially the detection of fake output reports. We formalize exact input reconstruction as a discrete optimisation problem with a unique global minimum and introduce SODA, an efficient gradient-based algorithm that operates on a continuous relaxation of the input search space with periodic restarts and parameter decay. Through comprehensive experiments on LLMs ranging in size from 33M to 3B parameters, we demonstrate that SODA significantly outperforms existing approaches. We succeed in fully recovering 79.5% of shorter out-of-distribution inputs from next-token logits, without a single false positive, but struggle to extract private information from the outputs of longer (15+ token) input sequences. This suggests that standard deployment practices may currently provide adequate protection against malicious use of our method. Our code is available at https://doi.org/10.5281/zenodo.15539879.
* 9 pages, ICML 2025 Workshop on Reliable and Responsible Foundation
Models
Via
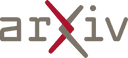
Jul 03, 2025
Abstract:Recent evidence suggests that modeling higher-order interactions (HOIs) in functional magnetic resonance imaging (fMRI) data can enhance the diagnostic accuracy of machine learning systems. However, effectively extracting and utilizing HOIs remains a significant challenge. In this work, we propose MvHo-IB, a novel multi-view learning framework that integrates both pairwise interactions and HOIs for diagnostic decision-making, while automatically compressing task-irrelevant redundant information. MvHo-IB introduces several key innovations: (1) a principled method that combines O-information from information theory with a matrix-based Renyi alpha-order entropy estimator to quantify and extract HOIs, (2) a purpose-built Brain3DCNN encoder to effectively utilize these interactions, and (3) a new multi-view learning information bottleneck objective to enhance representation learning. Experiments on three benchmark fMRI datasets demonstrate that MvHo-IB achieves state-of-the-art performance, significantly outperforming previous methods, including recent hypergraph-based techniques. The implementation of MvHo-IB is available at https://github.com/zky04/MvHo-IB.
* Accepted by MICCAI-25, code is available at
\url{https://github.com/zky04/MvHo-IB}
Via
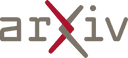
Jul 03, 2025
Abstract:Students disengaging from their tasks can have serious long-term consequences, including academic drop-out. This is particularly relevant for students in distance education. One way to measure the level of disengagement in distance education is to observe participation in non-mandatory exercises in different online courses. In this paper, we detect student disengagement in the non-mandatory quizzes of 42 courses in four semesters from a distance-based university. We carefully identified the most informative student log data that could be extracted and processed from Moodle. Then, eight machine learning algorithms were trained and compared to obtain the highest possible prediction accuracy. Using the SHAP method, we developed an explainable machine learning framework that allows practitioners to better understand the decisions of the trained algorithm. The experimental results show a balanced accuracy of 91\%, where about 85\% of disengaged students were correctly detected. On top of the highly predictive performance and explainable framework, we provide a discussion on how to design a timely intervention to minimise disengagement from voluntary tasks in online learning.
Via
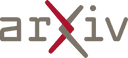
Jul 03, 2025
Abstract:General-purpose audio representations have proven effective across diverse music information retrieval applications, yet their utility in intelligent music production remains limited by insufficient understanding of audio effects (Fx). Although previous approaches have emphasized audio effects analysis at the mixture level, this focus falls short for tasks demanding instrument-wise audio effects understanding, such as automatic mixing. In this work, we present Fx-Encoder++, a novel model designed to extract instrument-wise audio effects representations from music mixtures. Our approach leverages a contrastive learning framework and introduces an "extractor" mechanism that, when provided with instrument queries (audio or text), transforms mixture-level audio effects embeddings into instrument-wise audio effects embeddings. We evaluated our model across retrieval and audio effects parameter matching tasks, testing its performance across a diverse range of instruments. The results demonstrate that Fx-Encoder++ outperforms previous approaches at mixture level and show a novel ability to extract effects representation instrument-wise, addressing a critical capability gap in intelligent music production systems.
* ISMIR 2025
Via
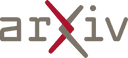
Jul 03, 2025
Abstract:Utilizing hyperspectral remote sensing technology enables the extraction of fine-grained land cover classes. Typically, satellite or airborne images used for training and testing are acquired from different regions or times, where the same class has significant spectral shifts in different scenes. In this paper, we propose a Bi-directional Domain Adaptation (BiDA) framework for cross-domain hyperspectral image (HSI) classification, which focuses on extracting both domain-invariant features and domain-specific information in the independent adaptive space, thereby enhancing the adaptability and separability to the target scene. In the proposed BiDA, a triple-branch transformer architecture (the source branch, target branch, and coupled branch) with semantic tokenizer is designed as the backbone. Specifically, the source branch and target branch independently learn the adaptive space of source and target domains, a Coupled Multi-head Cross-attention (CMCA) mechanism is developed in coupled branch for feature interaction and inter-domain correlation mining. Furthermore, a bi-directional distillation loss is designed to guide adaptive space learning using inter-domain correlation. Finally, we propose an Adaptive Reinforcement Strategy (ARS) to encourage the model to focus on specific generalized feature extraction within both source and target scenes in noise condition. Experimental results on cross-temporal/scene airborne and satellite datasets demonstrate that the proposed BiDA performs significantly better than some state-of-the-art domain adaptation approaches. In the cross-temporal tree species classification task, the proposed BiDA is more than 3\%$\sim$5\% higher than the most advanced method. The codes will be available from the website: https://github.com/YuxiangZhang-BIT/IEEE_TCSVT_BiDA.
Via
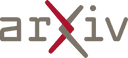
Jul 02, 2025
Abstract:The Internet of Vehicles (IoV) transforms the transportation ecosystem promising pervasive connectivity and data-driven approaches. Deep learning and generative Artificial Intelligence (AI) have the potential to significantly enhance the operation of applications within IoV by facilitating efficient decision-making and predictive capabilities, including intelligent navigation, vehicle safety monitoring, accident prevention, and intelligent traffic management. Nevertheless, efficiently transmitting and processing the massive volumes of data generated by the IoV in real-time remains a significant challenge, particularly in dynamic and unpredictable wireless channel conditions. To address these challenges, this paper proposes a semantic communication framework based on channel perception to improve the accuracy and efficiency of data transmission. The semantic communication model extracts and compresses the information to be transmitted. In addition, the wireless channel is estimated by using a generative diffusion model, which is employed to predict the dynamic channel states, thereby improving the quality of IoV service. In dynamic scenarios, however, the channel estimation performance may be degraded when substantially new scenarios take place, which will adversely affect user experience. To mitigate this limitation, we employ a large model to fine-tune the channel generation model to enhance its adaptability for varying scenarios. The performance and reliability of the proposed framework are evaluated on the two public datasets.
Via
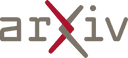
Jul 02, 2025
Abstract:In orchard automation, dense foliage during the canopy season severely occludes tree structures, minimizing visibility to various canopy parts such as trunks and branches, which limits the ability of a machine vision system. However, canopy structure is more open and visible during the dormant season when trees are defoliated. In this work, we present an information fusion framework that integrates multi-seasonal structural data to support robotic and automated crop load management during the entire growing season. The framework combines high-resolution RGB-D imagery from both dormant and canopy periods using YOLOv9-Seg for instance segmentation, Kinect Fusion for 3D reconstruction, and Fast Generalized Iterative Closest Point (Fast GICP) for model alignment. Segmentation outputs from YOLOv9-Seg were used to extract depth-informed masks, which enabled accurate 3D point cloud reconstruction via Kinect Fusion; these reconstructed models from each season were subsequently aligned using Fast GICP to achieve spatially coherent multi-season fusion. The YOLOv9-Seg model, trained on manually annotated images, achieved a mean squared error (MSE) of 0.0047 and segmentation mAP@50 scores up to 0.78 for trunks in dormant season dataset. Kinect Fusion enabled accurate reconstruction of tree geometry, validated with field measurements resulting in root mean square errors (RMSE) of 5.23 mm for trunk diameter, 4.50 mm for branch diameter, and 13.72 mm for branch spacing. Fast GICP achieved precise cross-seasonal registration with a minimum fitness score of 0.00197, allowing integrated, comprehensive tree structure modeling despite heavy occlusions during the growing season. This fused structural representation enables robotic systems to access otherwise obscured architectural information, improving the precision of pruning, thinning, and other automated orchard operations.
* 17 pages, 4 tables, 11 figures
Via
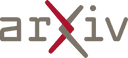
Jul 03, 2025
Abstract:We introduce a deepfake video detection approach that exploits pixel-wise temporal inconsistencies, which traditional spatial frequency-based detectors often overlook. Traditional detectors represent temporal information merely by stacking spatial frequency spectra across frames, resulting in the failure to detect temporal artifacts in the pixel plane. Our approach performs a 1D Fourier transform on the time axis for each pixel, extracting features highly sensitive to temporal inconsistencies, especially in areas prone to unnatural movements. To precisely locate regions containing the temporal artifacts, we introduce an attention proposal module trained in an end-to-end manner. Additionally, our joint transformer module effectively integrates pixel-wise temporal frequency features with spatio-temporal context features, expanding the range of detectable forgery artifacts. Our framework represents a significant advancement in deepfake video detection, providing robust performance across diverse and challenging detection scenarios.
* accepted by iccv 2025. code is will be available at
https://github.com/rama0126/PwTF-DVD
Via
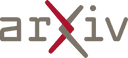