Topic:Information Extraction
What is Information Extraction? Information extraction is the process of automatically extracting structured information from unstructured text data.
Papers and Code
Jun 12, 2025
Abstract:Music-to-dance generation aims to synthesize human dance motion conditioned on musical input. Despite recent progress, significant challenges remain due to the semantic gap between music and dance motion, as music offers only abstract cues, such as melody, groove, and emotion, without explicitly specifying the physical movements. Moreover, a single piece of music can produce multiple plausible dance interpretations. This one-to-many mapping demands additional guidance, as music alone provides limited information for generating diverse dance movements. The challenge is further amplified by the scarcity of paired music and dance data, which restricts the model\^a\u{A}\'Zs ability to learn diverse dance patterns. In this paper, we introduce DanceChat, a Large Language Model (LLM)-guided music-to-dance generation approach. We use an LLM as a choreographer that provides textual motion instructions, offering explicit, high-level guidance for dance generation. This approach goes beyond implicit learning from music alone, enabling the model to generate dance that is both more diverse and better aligned with musical styles. Our approach consists of three components: (1) an LLM-based pseudo instruction generation module that produces textual dance guidance based on music style and structure, (2) a multi-modal feature extraction and fusion module that integrates music, rhythm, and textual guidance into a shared representation, and (3) a diffusion-based motion synthesis module together with a multi-modal alignment loss, which ensures that the generated dance is aligned with both musical and textual cues. Extensive experiments on AIST++ and human evaluations show that DanceChat outperforms state-of-the-art methods both qualitatively and quantitatively.
Via
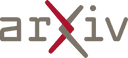
Jun 09, 2025
Abstract:Recent advancements in diffusion models have enabled high-fidelity and photorealistic image generation across diverse applications. However, these models also present security and privacy risks, including copyright violations, sensitive information leakage, and the creation of harmful or offensive content that could be exploited maliciously. In this study, we uncover a novel security threat where an attacker leverages diffusion model APIs to generate synthetic images, which are then used to train a high-performing substitute model. This enables the attacker to execute model extraction and transfer-based adversarial attacks on black-box classification models with minimal queries, without needing access to the original training data. The generated images are sufficiently high-resolution and diverse to train a substitute model whose outputs closely match those of the target model. Across the seven benchmarks, including CIFAR and ImageNet subsets, our method shows an average improvement of 27.37% over state-of-the-art methods while using just 0.01 times of the query budget, achieving a 98.68% success rate in adversarial attacks on the target model.
Via
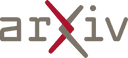
Jun 11, 2025
Abstract:Recent approaches have shown impressive proficiency in extracting and leveraging parametric knowledge from Large-Language Models (LLMs) and Vision-Language Models (VLMs). In this work, we consider how we can improve the identification and retrieval of videos related to complex real-world events by automatically extracting latent parametric knowledge about those events. We present Q2E: a Query-to-Event decomposition method for zero-shot multilingual text-to-video retrieval, adaptable across datasets, domains, LLMs, or VLMs. Our approach demonstrates that we can enhance the understanding of otherwise overly simplified human queries by decomposing the query using the knowledge embedded in LLMs and VLMs. We additionally show how to apply our approach to both visual and speech-based inputs. To combine this varied multimodal knowledge, we adopt entropy-based fusion scoring for zero-shot fusion. Through evaluations on two diverse datasets and multiple retrieval metrics, we demonstrate that Q2E outperforms several state-of-the-art baselines. Our evaluation also shows that integrating audio information can significantly improve text-to-video retrieval. We have released code and data for future research.
Via
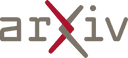
Jun 07, 2025
Abstract:Audio-visual speech separation (AVSS) aims to extract a target speech signal from a mixed signal by leveraging both auditory and visual (lip movement) cues. However, most existing AVSS methods exhibit complex architectures and rely on future context, operating offline, which renders them unsuitable for real-time applications. Inspired by the pipeline of RTFSNet, we propose a novel streaming AVSS model, named Swift-Net, which enhances the causal processing capabilities required for real-time applications. Swift-Net adopts a lightweight visual feature extraction module and an efficient fusion module for audio-visual integration. Additionally, Swift-Net employs Grouped SRUs to integrate historical information across different feature spaces, thereby improving the utilization efficiency of historical information. We further propose a causal transformation template to facilitate the conversion of non-causal AVSS models into causal counterparts. Experiments on three standard benchmark datasets (LRS2, LRS3, and VoxCeleb2) demonstrated that under causal conditions, our proposed Swift-Net exhibited outstanding performance, highlighting the potential of this method for processing speech in complex environments.
* 8 pages, 5 figures
Via
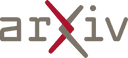
Jun 09, 2025
Abstract:Role-playing has emerged as an effective technique for enhancing the reasoning capabilities of large language models (LLMs). However, existing methods primarily rely on prompt engineering, which often lacks stability and interpretability. In this paper, we introduce Sparse Autoencoder Role-Playing Steering (SRPS), a novel framework that identifies and manipulates internal model features associated with role-playing behavior. Our approach extracts latent representations from role-play prompts, selects the most relevant features based on activation patterns, and constructs a steering vector that can be injected into the model's residual stream with controllable intensity. Our method enables fine-grained control over role-specific behavior and offers insights into how role information influences internal model activations. Extensive experiments across various reasoning benchmarks and model sizes demonstrate consistent performance gains. Notably, in the zero-shot chain-of-thought (CoT) setting, the accuracy of Llama3.1-8B on CSQA improves from 31.86% to 39.80%, while Gemma2-9B on SVAMP increases from 37.50% to 45.10%. These results highlight the potential of SRPS to enhance reasoning ability in LLMs, providing better interpretability and stability compared to traditional prompt-based role-playing.
* 21 pages, 8 figures, 8 tables
Via
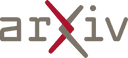
Jun 08, 2025
Abstract:We present HOI-PAGE, a new approach to synthesizing 4D human-object interactions (HOIs) from text prompts in a zero-shot fashion, driven by part-level affordance reasoning. In contrast to prior works that focus on global, whole body-object motion for 4D HOI synthesis, we observe that generating realistic and diverse HOIs requires a finer-grained understanding -- at the level of how human body parts engage with object parts. We thus introduce Part Affordance Graphs (PAGs), a structured HOI representation distilled from large language models (LLMs) that encodes fine-grained part information along with contact relations. We then use these PAGs to guide a three-stage synthesis: first, decomposing input 3D objects into geometric parts; then, generating reference HOI videos from text prompts, from which we extract part-based motion constraints; finally, optimizing for 4D HOI motion sequences that not only mimic the reference dynamics but also satisfy part-level contact constraints. Extensive experiments show that our approach is flexible and capable of generating complex multi-object or multi-person interaction sequences, with significantly improved realism and text alignment for zero-shot 4D HOI generation.
Via
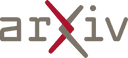
Jun 08, 2025
Abstract:Real-world surveillance often renders faces and license plates unrecognizable in individual low-resolution (LR) frames, hindering reliable identification. To advance temporal recognition models, we present FANVID, a novel video-based benchmark comprising nearly 1,463 LR clips (180 x 320, 20--60 FPS) featuring 63 identities and 49 license plates from three English-speaking countries. Each video includes distractor faces and plates, increasing task difficulty and realism. The dataset contains 31,096 manually verified bounding boxes and labels. FANVID defines two tasks: (1) face matching -- detecting LR faces and matching them to high-resolution mugshots, and (2) license plate recognition -- extracting text from LR plates without a predefined database. Videos are downsampled from high-resolution sources to ensure that faces and text are indecipherable in single frames, requiring models to exploit temporal information. We introduce evaluation metrics adapted from mean Average Precision at IoU > 0.5, prioritizing identity correctness for faces and character-level accuracy for text. A baseline method with pre-trained video super-resolution, detection, and recognition achieved performance scores of 0.58 (face matching) and 0.42 (plate recognition), highlighting both the feasibility and challenge of the tasks. FANVID's selection of faces and plates balances diversity with recognition challenge. We release the software for data access, evaluation, baseline, and annotation to support reproducibility and extension. FANVID aims to catalyze innovation in temporal modeling for LR recognition, with applications in surveillance, forensics, and autonomous vehicles.
Via
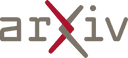
Jun 09, 2025
Abstract:Large Language Models (LLMs) memorize, and thus, among huge amounts of uncontrolled data, may memorize Personally Identifiable Information (PII), which should not be stored and, consequently, not leaked. In this paper, we introduce Private Memorization Editing (PME), an approach for preventing private data leakage that turns an apparent limitation, that is, the LLMs' memorization ability, into a powerful privacy defense strategy. While attacks against LLMs have been performed exploiting previous knowledge regarding their training data, our approach aims to exploit the same kind of knowledge in order to make a model more robust. We detect a memorized PII and then mitigate the memorization of PII by editing a model knowledge of its training data. We verify that our procedure does not affect the underlying language model while making it more robust against privacy Training Data Extraction attacks. We demonstrate that PME can effectively reduce the number of leaked PII in a number of configurations, in some cases even reducing the accuracy of the privacy attacks to zero.
* To be published at ACL 2025 (Main)
Via
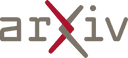
Jun 09, 2025
Abstract:With the rapid development of digital pathology, virtual staining has become a key technology in multimedia medical information systems, offering new possibilities for the analysis and diagnosis of pathological images. However, existing H&E-to-IHC studies often overlook the cross-channel correlations between cell nuclei and cell membranes. To address this issue, we propose a novel Cross-Channel Perception Learning (CCPL) strategy. Specifically, CCPL first decomposes HER2 immunohistochemical staining into Hematoxylin and DAB staining channels, corresponding to cell nuclei and cell membranes, respectively. Using the pathology foundation model Gigapath's Tile Encoder, CCPL extracts dual-channel features from both the generated and real images and measures cross-channel correlations between nuclei and membranes. The features of the generated and real stained images, obtained through the Tile Encoder, are also used to calculate feature distillation loss, enhancing the model's feature extraction capabilities without increasing the inference burden. Additionally, CCPL performs statistical analysis on the focal optical density maps of both single channels to ensure consistency in staining distribution and intensity. Experimental results, based on quantitative metrics such as PSNR, SSIM, PCC, and FID, along with professional evaluations from pathologists, demonstrate that CCPL effectively preserves pathological features, generates high-quality virtual stained images, and provides robust support for automated pathological diagnosis using multimedia medical data.
Via
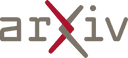
Jun 10, 2025
Abstract:In the era of information explosion, efficiently leveraging large-scale unlabeled data while minimizing the reliance on high-quality pixel-level annotations remains a critical challenge in the field of medical imaging. Semi-supervised learning (SSL) enhances the utilization of unlabeled data by facilitating knowledge transfer, significantly improving the performance of fully supervised models and emerging as a highly promising research direction in medical image analysis. Inspired by the ability of Vision Foundation Models (e.g., SAM-2) to provide rich prior knowledge, we propose SSS (Semi-Supervised SAM-2), a novel approach that leverages SAM-2's robust feature extraction capabilities to uncover latent knowledge in unlabeled medical images, thus effectively enhancing feature support for fully supervised medical image segmentation. Specifically, building upon the single-stream "weak-to-strong" consistency regularization framework, this paper introduces a Discriminative Feature Enhancement (DFE) mechanism to further explore the feature discrepancies introduced by various data augmentation strategies across multiple views. By leveraging feature similarity and dissimilarity across multi-scale augmentation techniques, the method reconstructs and models the features, thereby effectively optimizing the salient regions. Furthermore, a prompt generator is developed that integrates Physical Constraints with a Sliding Window (PCSW) mechanism to generate input prompts for unlabeled data, fulfilling SAM-2's requirement for additional prompts. Extensive experiments demonstrate the superiority of the proposed method for semi-supervised medical image segmentation on two multi-label datasets, i.e., ACDC and BHSD. Notably, SSS achieves an average Dice score of 53.15 on BHSD, surpassing the previous state-of-the-art method by +3.65 Dice. Code will be available at https://github.com/AIGeeksGroup/SSS.
Via
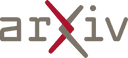