Topic:Information Extraction
What is Information Extraction? Information extraction is the process of automatically extracting structured information from unstructured text data.
Papers and Code
Jun 09, 2025
Abstract:Despite recent advances in retrieval-augmented generation (RAG) for video understanding, effectively understanding long-form video content remains underexplored due to the vast scale and high complexity of video data. Current RAG approaches typically segment videos into fixed-length chunks, which often disrupts the continuity of contextual information and fails to capture authentic scene boundaries. Inspired by the human ability to naturally organize continuous experiences into coherent scenes, we present SceneRAG, a unified framework that leverages large language models to segment videos into narrative-consistent scenes by processing ASR transcripts alongside temporal metadata. SceneRAG further sharpens these initial boundaries through lightweight heuristics and iterative correction. For each scene, the framework fuses information from both visual and textual modalities to extract entity relations and dynamically builds a knowledge graph, enabling robust multi-hop retrieval and generation that account for long-range dependencies. Experiments on the LongerVideos benchmark, featuring over 134 hours of diverse content, confirm that SceneRAG substantially outperforms prior baselines, achieving a win rate of up to 72.5 percent on generation tasks.
Via
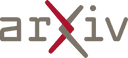
Jun 11, 2025
Abstract:This paper compares the performance of BiLSTM and CNN+GRU deep learning models for Human Activity Recognition (HAR) on two WiFi-based Channel State Information (CSI) datasets: UT-HAR and NTU-Fi HAR. The findings indicate that the CNN+GRU model has a higher accuracy on the UT-HAR dataset (95.20%) thanks to its ability to extract spatial features. In contrast, the BiLSTM model performs better on the high-resolution NTU-Fi HAR dataset (92.05%) by extracting long-term temporal dependencies more effectively. The findings strongly emphasize the critical role of dataset characteristics and preprocessing techniques in model performance improvement. We also show the real-world applicability of such models in applications like healthcare and intelligent home systems, highlighting their potential for unobtrusive activity recognition.
* This Paper has been Accepted and will appear in the 23rd IEEE/ACIS
International Conference on Software Engineering, Management and Applications
(SERA 2025)
Via
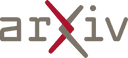
Jun 04, 2025
Abstract:Graph Retrieval Augmented Generation (GraphRAG) effectively enhances external knowledge integration capabilities by explicitly modeling knowledge relationships, thereby improving the factual accuracy and generation quality of Large Language Models (LLMs) in specialized domains. However, existing methods suffer from two inherent limitations: 1) Inefficient Information Aggregation: They rely on a single agent and fixed iterative patterns, making it difficult to adaptively capture multi-level textual, structural, and degree information within graph data. 2) Rigid Reasoning Mechanism: They employ preset reasoning schemes, which cannot dynamically adjust reasoning depth nor achieve precise semantic correction. To overcome these limitations, we propose Graph Counselor, an GraphRAG method based on multi-agent collaboration. This method uses the Adaptive Graph Information Extraction Module (AGIEM), where Planning, Thought, and Execution Agents work together to precisely model complex graph structures and dynamically adjust information extraction strategies, addressing the challenges of multi-level dependency modeling and adaptive reasoning depth. Additionally, the Self-Reflection with Multiple Perspectives (SR) module improves the accuracy and semantic consistency of reasoning results through self-reflection and backward reasoning mechanisms. Experiments demonstrate that Graph Counselor outperforms existing methods in multiple graph reasoning tasks, exhibiting higher reasoning accuracy and generalization ability. Our code is available at https://github.com/gjq100/Graph-Counselor.git.
* Accepted by ACL 2025
Via
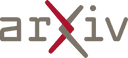
Jun 06, 2025
Abstract:Heterogeneous graph neural networks (HGNNs) excel at capturing structural and semantic information in heterogeneous graphs (HGs), while struggling to generalize across domains and tasks. Recently, some researchers have turned to integrating HGNNs with large language models (LLMs) for more generalizable heterogeneous graph learning. However, these approaches typically extract structural information via HGNNs as HG tokens, and disparities in embedding spaces between HGNNs and LLMs have been shown to bias the LLM's comprehension of HGs. Moreover, as these HG tokens are often derived from node-level tasks, the model's ability to generalize across tasks remains limited. To this end, we propose a simple yet effective Masked Language Modeling-based method, called MLM4HG. MLM4HG introduces metapath-based textual sequences instead of HG tokens to extract structural and semantic information inherent in HGs, and designs customized textual templates to unify different graph tasks into a coherent cloze-style "mask" token prediction paradigm. Specifically, MLM4HG first converts HGs from various domains to texts based on metapaths, and subsequently combines them with the unified task texts to form a HG-based corpus. Moreover, the corpus is fed into a pretrained LM for fine-tuning with a constrained target vocabulary, enabling the fine-tuned LM to generalize to unseen target HGs. Extensive cross-domain and multi-task experiments on four real-world datasets demonstrate the superior generalization performance of MLM4HG over state-of-the-art methods in both few-shot and zero-shot scenarios. Our code is available at https://github.com/BUPT-GAMMA/MLM4HG.
Via
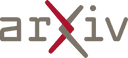
Jun 11, 2025
Abstract:Recently, multimodal tasks have strongly advanced the field of action recognition with their rich multimodal information. However, due to the scarcity of tri-modal data, research on tri-modal action recognition tasks faces many challenges. To this end, we have proposed a comprehensive multimodal action recognition solution that effectively utilizes multimodal information. First, the existing data are transformed and expanded by optimizing data enhancement techniques to enlarge the training scale. At the same time, more RGB datasets are used to pre-train the backbone network, which is better adapted to the new task by means of transfer learning. Secondly, multimodal spatial features are extracted with the help of 2D CNNs and combined with the Temporal Shift Module (TSM) to achieve multimodal spatial-temporal feature extraction comparable to 3D CNNs and improve the computational efficiency. In addition, common prediction enhancement methods, such as Stochastic Weight Averaging (SWA), Ensemble and Test-Time augmentation (TTA), are used to integrate the knowledge of models from different training periods of the same architecture and different architectures, so as to predict the actions from different perspectives and fully exploit the target information. Ultimately, we achieved the Top-1 accuracy of 99% and the Top-5 accuracy of 100% on the competition leaderboard, demonstrating the superiority of our solution.
Via
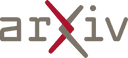
Jun 05, 2025
Abstract:Electric bicycles (e-bikes) are rapidly increasing in use, raising safety concerns due to a rise in accident reports. However, e-bike incident reports often use unstructured narrative formats, which hinders quantitative safety analysis. This study introduces E-bike agents, a framework that uses large language models (LLM) powered agents to classify and extract safety variables from unstructured incident reports. Our framework consists of four LLM agents, handling data classification, information extraction, injury cause determination, and component linkage, to extract the key factors that could lead to E-bike accidents and cause varying severity levels. Furthermore, we used an ordered logit model to examine the relationship between the severity of the incident and the factors retrieved, such as gender, the type of cause, and environmental conditions. Our research shows that equipment issues are slightly more common than human-related ones, but human-related incidents are more often fatal. Specifically, pedals, tires, and brakes are frequent contributors to accidents. The model achieves a high weighted F1 score of 0.87 in classification accuracy, highlighting the potential of using LLMs to extract unstructured data in niche domains, such as transportation. Our method offers a scalable solution to improve e-bike safety analytics and provides actionable information for policy makers, designers, and regulators.
Via
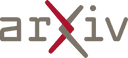
Jun 10, 2025
Abstract:Accurate segmentation of anatomical structures in the apical four-chamber (A4C) view of fetal echocardiography is essential for early diagnosis and prenatal evaluation of congenital heart disease (CHD). However, precise segmentation remains challenging due to ultrasound artifacts, speckle noise, anatomical variability, and boundary ambiguity across different gestational stages. To reduce the workload of sonographers and enhance segmentation accuracy, we propose DCD, an advanced deep learning-based model for automatic segmentation of key anatomical structures in the fetal A4C view. Our model incorporates a Dense Atrous Spatial Pyramid Pooling (Dense ASPP) module, enabling superior multi-scale feature extraction, and a Convolutional Block Attention Module (CBAM) to enhance adaptive feature representation. By effectively capturing both local and global contextual information, DCD achieves precise and robust segmentation, contributing to improved prenatal cardiac assessment.
Via
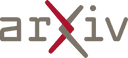
Jun 11, 2025
Abstract:California is a global leader in agricultural production, contributing 12.5% of the United States total output and ranking as the fifth-largest food and cotton supplier in the world. Despite the availability of extensive historical yield data from the USDA National Agricultural Statistics Service, accurate and timely crop yield forecasting remains a challenge due to the complex interplay of environmental, climatic, and soil-related factors. In this study, we introduce a comprehensive crop yield benchmark dataset covering over 70 crops across all California counties from 2008 to 2022. The benchmark integrates diverse data sources, including Landsat satellite imagery, daily climate records, monthly evapotranspiration, and high-resolution soil properties. To effectively learn from these heterogeneous inputs, we develop a multi-modal deep learning model tailored for county-level, crop-specific yield forecasting. The model employs stratified feature extraction and a timeseries encoder to capture spatial and temporal dynamics during the growing season. Static inputs such as soil characteristics and crop identity inform long-term variability. Our approach achieves an overall R2 score of 0.76 across all crops of unseen test dataset, highlighting strong predictive performance across California diverse agricultural regions. This benchmark and modeling framework offer a valuable foundation for advancing agricultural forecasting, climate adaptation, and precision farming. The full dataset and codebase are publicly available at our GitHub repository.
Via
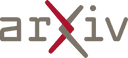
Jun 12, 2025
Abstract:Large language models (LLMs) often struggle with knowledge-intensive tasks due to a lack of background knowledge and a tendency to hallucinate. To address these limitations, integrating knowledge graphs (KGs) with LLMs has been intensively studied. Existing KG-enhanced LLMs focus on supplementary factual knowledge, but still struggle with solving complex questions. We argue that refining the relationships among facts and organizing them into a logically consistent reasoning path is equally important as factual knowledge itself. Despite their potential, extracting reliable reasoning paths from KGs poses the following challenges: the complexity of graph structures and the existence of multiple generated paths, making it difficult to distinguish between useful and redundant ones. To tackle these challenges, we propose the RRP framework to mine the knowledge graph, which combines the semantic strengths of LLMs with structural information obtained through relation embedding and bidirectional distribution learning. Additionally, we introduce a rethinking module that evaluates and refines reasoning paths according to their significance. Experimental results on two public datasets show that RRP achieves state-of-the-art performance compared to existing baseline methods. Moreover, RRP can be easily integrated into various LLMs to enhance their reasoning abilities in a plug-and-play manner. By generating high-quality reasoning paths tailored to specific questions, RRP distills effective guidance for LLM reasoning.
Via
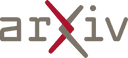
Jun 11, 2025
Abstract:Hyperspectral image (HSI) clustering assigns similar pixels to the same class without any annotations, which is an important yet challenging task. For large-scale HSIs, most methods rely on superpixel segmentation and perform superpixel-level clustering based on graph neural networks (GNNs). However, existing GNNs cannot fully exploit the spectral information of the input HSI, and the inaccurate superpixel topological graph may lead to the confusion of different class semantics during information aggregation. To address these challenges, we first propose a structural-spectral graph convolutional operator (SSGCO) tailored for graph-structured HSI superpixels to improve their representation quality through the co-extraction of spatial and spectral features. Second, we propose an evidence-guided adaptive edge learning (EGAEL) module that adaptively predicts and refines edge weights in the superpixel topological graph. We integrate the proposed method into a contrastive learning framework to achieve clustering, where representation learning and clustering are simultaneously conducted. Experiments demonstrate that the proposed method improves clustering accuracy by 2.61%, 6.06%, 4.96% and 3.15% over the best compared methods on four HSI datasets. Our code is available at https://github.com/jhqi/SSGCO-EGAEL.
Via
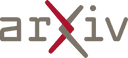