May 24, 2025
Abstract:Vision-language models (VLMs), such as CLIP, have demonstrated strong performance across a range of downstream tasks. However, CLIP is still limited in negation understanding: the ability to recognize the absence or exclusion of a concept. Existing methods address the problem by using a large language model (LLM) to generate large-scale data of image captions containing negation for further fine-tuning CLIP. However, these methods are both time- and compute-intensive, and their evaluations are typically restricted to image-text matching tasks. To expand the horizon, we (1) introduce a training-time negation data generation pipeline such that negation captions are generated during the training stage, which only increases 2.5% extra training time, and (2) we propose the first benchmark, Neg-TtoI, for evaluating text-to-image generation models on prompts containing negation, assessing model's ability to produce semantically accurate images. We show that our proposed method, TNG-CLIP, achieves SOTA performance on diverse negation benchmarks of image-to-text matching, text-to-image retrieval, and image generation.
* 15 pages, 3 figures
Via
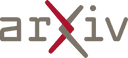