Jul 03, 2025
Abstract:Interviews are a widely used technique in eliciting requirements to gather stakeholder needs, preferences, and expectations for a software system. Effective interviewing requires skilled interviewers to formulate appropriate interview questions in real time while facing multiple challenges, including lack of familiarity with the domain, excessive cognitive load, and information overload that hinders how humans process stakeholders' speech. Recently, large language models (LLMs) have exhibited state-of-the-art performance in multiple natural language processing tasks, including text summarization and entailment. To support interviewers, we investigate the application of GPT-4o to generate follow-up interview questions during requirements elicitation by building on a framework of common interviewer mistake types. In addition, we describe methods to generate questions based on interviewee speech. We report a controlled experiment to evaluate LLM-generated and human-authored questions with minimal guidance, and a second controlled experiment to evaluate the LLM-generated questions when generation is guided by interviewer mistake types. Our findings demonstrate that, for both experiments, the LLM-generated questions are no worse than the human-authored questions with respect to clarity, relevancy, and informativeness. In addition, LLM-generated questions outperform human-authored questions when guided by common mistakes types. This highlights the potential of using LLMs to help interviewers improve the quality and ease of requirements elicitation interviews in real time.
* 13 pages, 2 figures, accepted at the 33rd IEEE International
Requirements Engineering 2025
Via
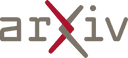