Feb 23, 2025
Abstract:We introduce PhotoDoodle, a novel image editing framework designed to facilitate photo doodling by enabling artists to overlay decorative elements onto photographs. Photo doodling is challenging because the inserted elements must appear seamlessly integrated with the background, requiring realistic blending, perspective alignment, and contextual coherence. Additionally, the background must be preserved without distortion, and the artist's unique style must be captured efficiently from limited training data. These requirements are not addressed by previous methods that primarily focus on global style transfer or regional inpainting. The proposed method, PhotoDoodle, employs a two-stage training strategy. Initially, we train a general-purpose image editing model, OmniEditor, using large-scale data. Subsequently, we fine-tune this model with EditLoRA using a small, artist-curated dataset of before-and-after image pairs to capture distinct editing styles and techniques. To enhance consistency in the generated results, we introduce a positional encoding reuse mechanism. Additionally, we release a PhotoDoodle dataset featuring six high-quality styles. Extensive experiments demonstrate the advanced performance and robustness of our method in customized image editing, opening new possibilities for artistic creation.
Via
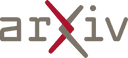